the Creative Commons Attribution 4.0 License.
the Creative Commons Attribution 4.0 License.
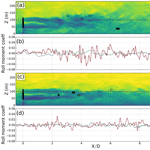
Do wind turbines pose roll hazards to light aircraft?
Jessica M. Tomaszewski
Julie K. Lundquist
Matthew J. Churchfield
Patrick J. Moriarty
Wind energy accounted for 5.6 % of all electricity generation in the United States in 2016. Much of this development has occurred in rural locations, where open spaces favorable for harnessing wind also serve general aviation airports. As such, nearly 40 % of all United States wind turbines exist within 10 km of a small airport. Wind turbines generate electricity by extracting momentum from the atmosphere, creating downwind wakes characterized by wind-speed deficits and increased turbulence. Recently, the concern that turbine wakes pose hazards for small aircraft has been used to limit wind-farm development. Herein, we assess roll hazards to small aircraft using large-eddy simulations (LES) of a utility-scale turbine wake. Wind-generated lift forces and subsequent rolling moments are calculated for hypothetical aircraft transecting the wake in various orientations. Stably and neutrally stratified cases are explored, with the stable case presenting a possible worst-case scenario due to longer-persisting wakes permitted by lower ambient turbulence. In both cases, only 0.001 % of rolling moments experienced by hypothetical aircraft during down-wake and cross-wake transects lead to an increased risk of rolling.
- Article
(6493 KB) - Full-text XML
- BibTeX
- EndNote
Due to its appeal as a renewable, low-carbon energy source, wind energy development has increased rapidly, accounting for 5.6 % of electricity generation in the United States at the end of 2016 (EIA, 2017). Wind turbines generate electricity by extracting momentum from the atmosphere, thereby creating wakes characterized by a wind-speed deficit and increased turbulence downwind (Baker and Walker, 1984; Lissaman, 1979; Sforza et al., 1981). As the size of turbine rotors increases, turbine wakes also increase, which may pose a greater hazard to nearby flying aircraft (Wang et al., 2015). Therefore, improved understanding of turbine wake characteristics is crucial to assess hazards to aircraft posed by wakes.
Numerous studies have investigated the structure, duration, and decay of wind turbine wakes. Most of these studies address wake impacts on surface temperature (Rajewski et al., 2013; Smith et al., 2013; Zhou et al., 2012) or surface fluxes (Rajewski et al., 2014, 2016). Detailed measurements of winds in turbine wakes have been taken with lidars (e.g., Aitken et al., 2014a; Iungo et al., 2012; Käsler et al., 2010; Rhodes and Lundquist, 2013). Wind tunnel experiments have also been used to further aerodynamic research on wind turbines (Chamorro and Porté-Agel, 2009; Hancock and Zhang, 2015; Snel et al., 2007; Vermeer et al., 2003; Yang et al., 2012). Scaling such experiments to utility-scale turbines can be challenging: wind tunnel measurements typically cannot account for complexities in atmospheric profiles, such as inversions and wind veer. Computational fluid dynamics (CFD) simulations of wind turbine wakes provide insights into turbine wakes (Sanderse et al., 2011). Both actuator-disk (Calaf et al., 2010; Mirocha et al., 2014; Vanderwende et al., 2016; Vermeer et al., 2003) and actuator-line (Churchfield et al., 2012; Marjanovic et al., 2017; Sørensen and Shen, 2002; Troldborg et al., 2010) methods have been used to simulate the rotor and downstream flow to study wake structure.
Extensive wind development occurs in rural locations, where open spaces favorable for wind energy are also home to numerous general aviation airports. Almost 40 % of all wind turbines in the United States exist within 10 km of a small airport and about 5 % exist within 5 km of a small airport (Fig. 1, based on data from OurAirports, 2016; USGS, 2014). Currently, neither the Federal Aviation Administration (FAA) of the United States nor the Civil Aviation Authority (CAA) of the United Kingdom have detailed recommendations on the effects of wind-turbine-induced roll hazards for aircraft (CAA, 2012; Ho et al., 2014).
General aviation pilots are typically most concerned with the rolling moment (Wang et al., 2015), and we thus focus our study on this hazard. The rolling moment is the tendency for an aerodynamic force applied at a distance from an aircraft's center of mass to cause the aircraft to undergo angular acceleration about its roll axis, considered a torque in this study (Etkin and Reid, 1996). The roll axis is the longitudinal axis, running from the nose to the tail of the aircraft. Other wake hazards of concern to pilots are those generated from the wings of preceding aircraft during take-off as a consequence of lift (Gerz et al., 2009; Holzäpfel et al., 2007).
Previous work has argued that turbine wakes present, in particular, a serious roll hazard to general aviation aircraft. Mulinazzi and Zheng (2014) used a helical vortex model to represent a wind turbine wake from which aircraft roll hazards were calculated. The helical vortex model was scaled up from a wind tunnel study using miniature turbines with a rotor diameter of 0.254 m. The 4 m s−1 inflow was meant to trigger transient helical tip vortices (Yang et al., 2012). Mulinazzi and Zheng (2014) scaled up these wind tunnel results to the atmosphere by assuming comparable near- and far-wake turbulent flow structures between the tip vortices measured in the wind tunnel and the full wake produced in a real atmosphere by a utility-scale turbine. With calculations derived from this scaling, they suggest wind turbine wakes pose a significant roll hazard to general aviation aircraft at downwind distances as far as 4.57 km (2.84 miles) (Mulinazzi and Zheng, 2014). These findings have been used in multiple states to limit wind energy development. Reid Bell, airport manager at Pratt Regional Airport in Kansas, confirmed that the Pratt wind-farm project was relocated further away from the airport as a direct result of the Mulinazzi and Zheng (2014) study (Williams, 2014). The study has been used as a warning to aviators in Virginia as well (Hamilton, 2014).
Other researchers have also investigated the hazards that wind turbines pose to aircraft by computing the roll hazards from analytic representations of wakes. van der Wall et al. (2016) and van der Wall and Lehmann (2017) found that typical onshore turbines could pose hazards for gliders or ultralight helicopters but perhaps not for general aviation aircraft. Wang et al. (2015, 2017) computed a wake wind field derived using the Beddoes circulation formula (Madsen and Rasmussen, 2004), proven to adequately match lidar field observations made of a wind turbine wake. For a 30 m rotor diameter wind turbine, Wang et al. (2015, 2017) find that the wake does not pose any roll hazards for aircraft five rotor diameters downstream from the turbine.
Rather than approximating wakes, we seek to explicitly resolve turbine wakes within a dynamic, non-stationary atmosphere. We therefore use large-eddy simulations (LES) to assess wind-generated roll hazards to small aircraft from the wake of a utility-scale wind turbine. Section 2 provides details on our simulations and methodology for analyzing the model data. Section 3 presents the results of our analysis, specifically the quantified roll hazards on hypothetical aircraft. We conclude in Sect. 4 with a discussion of our results and suggestions for future work.
2.1 Simulations
LES is a well-established method for studying wind turbine wakes. LES have been used to investigate wind turbine wake turbulence (Churchfield et al., 2012; Jha et al., 2015), the wind-farm boundary layer (Calaf et al., 2010), and the evolution of the wake structure in variable stability conditions and throughout the diurnal cycle (Abkar et al., 2016; Aitken et al., 2014b; Bhaganagar and Debnath, 2014; Englberger and Dörnbrack, 2018; Lu and Porté-Agel, 2011; Mirocha et al., 2014, 2015). Jimenez et al. (2007) find good agreement between LES and experimental data of turbine wakes, which has led to the use of LES to correct for instrumentation error (e.g., Lundquist et al., 2015).
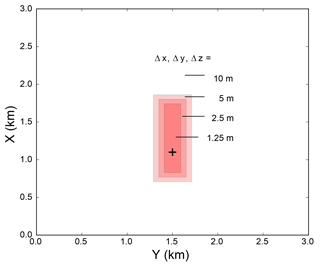
Figure 2Schematic of the SOWFA mesh, with model resolutions labeled at their respective locations in the domain. Turbine location is denoted by the plus sign.
Representing turbines in LES can be done via actuator disks, where the turbine rotor is represented by a permeable circular disk with uniformly distributed thrust forces (Aitken et al., 2014b; Calaf et al., 2010; Mirocha et al., 2014; Vanderwende et al., 2016; Vermeer et al., 2003) or by actuator lines, which represent the turbine blades as separate rotating lines (Nilsson et al., 2015; Porté-Agel et al., 2011; Sørensen and Shen, 2002). Martínez-Tossas et al. (2015) compare actuator-line and actuator-disk models and conclude that they produce similar wake profiles; however, the actuator-line model can generate fine flow structures near the blades such as root and tip vortices that the actuator-disk model cannot.
We perform our simulations using the Simulator fOr Wind Farm Applications (SOWFA; Churchfield and Lee, 2014; Fleming et al., 2013). SOWFA is a computational fluid dynamics solver coupled with a turbine dynamics model. The LES solver is based on the Open Field Operations and Manipulation (OpenFOAM) toolbox version 2.4.x (OpenCFD, 2016). OpenFOAM is a set of C++ libraries and applications for use in solving the partial differential equations describing fluid flow. The simulations utilize the same two-step methodology as described in Churchfield et al. (2012), briefly summarized here. First, a precursor LES generates turbulent atmospheric flow on a domain with idealized periodic lateral boundaries. Once the turbulent boundary layer reaches quasi-equilibrium, a plane of turbulent data from the upwind lateral boundary is saved at each time step to be used as inflow boundary conditions for the simulation with the turbine. Next, a turbine is introduced into the flow initialized from the quasi-equilibrium precursor flow field. The side boundaries remain periodic, but the saved velocity and temperature data from the precursor are used as inflow Dirichlet boundary conditions for this simulation. The outflow boundary uses Neumann, zero-normal-gradient conditions. Like the precursor, the overall domain size is 3 km × 3 km in the horizontal and 1 km in height, but variable resolution is used. Most of the domain has 10 m resolution, like the precursor, but around the turbine and its wake the resolution is gradually refined to 1.25 m (Fig. 2). The time step in the wind turbine simulation is restricted to capture the motion of the blade tips.
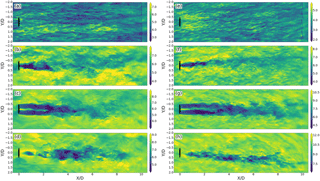
Figure 3Contoured horizontal cross sections of horizontal wind speeds (m s−1) comparing the neutral (a–d) and stable (e–h) cases at the near surface (1 m; a, e), rotor bottom (40 m; b, f), rotor center (80 m; c, g), and rotor top (120 m; d, h).
The turbine model consists of an actuator-line representation of turbine blades (Sørensen and Shen, 2002), proven to adequately capture the generation and downstream evolution of helical tip vortices (Churchfield et al., 2017; Ivanell et al., 2010; Lignarolo et al., 2015; Martínez-Tossas et al., 2017; Toloui et al., 2015). We model the GE 1.5-MW SLE wind turbine (Mendoza et al., 2015), a utility-scale turbine often deployed in standard industrial wind farms. This horizontal-axis, upwind turbine has a three-bladed rotor 77 m in diameter, with a hub height of 80 m. While not the largest turbine type currently available, the GE 1.5-MW SLE is one of the most widely deployed turbines worldwide, making the wake effects on general aviation aircraft explored herein broadly applicable to numerous wind farms.
A subvolume of the flow surrounding the turbine and wake was sampled at 1 Hz. This sampled subvolume uses a Cartesian coordinate system, within which positive x (y) corresponds to downwind (crosswind) and positive z corresponds to height above the surface. In all cases, the sampled subvolume extends 11 rotor diameters (D) in the x (downwind) direction, 4 D in the y (crosswind) direction, and 3 D in the vertical direction. The computational grid resolution is approximately 1.25 m, uniform in all directions (Fig. 2). This high resolution is necessary to adequately resolve flow structures that impact small (10 m) general aviation aircraft.
Previous observations note turbine wakes tend to diffuse more rapidly in convective conditions because the high ambient turbulent kinetic energy (TKE) of the surrounding air induces mechanical mixing to erode the wake (Baker and Walker, 1984; Bhaganagar and Debnath, 2014; Magnusson and Smedman, 1994; Mirocha et al., 2015). We thus hypothesize that stable conditions, with low TKE and a low turbulent eddy dissipation rate (EDR) (Bodini et al., 2018), present a worst-case scenario for general aviation aircraft due to longer-persisting wakes permitted by the reduced ambient TKE and EDR. As such, we simulate a neutral case and a stable case. Each simulation is spun up for 30 000 s, after which 100 s of data are output. The neutral case has a hub height inflow wind speed of 7.4 m s−1 and a constant potential temperature profile of 300 K, while the stable case has a hub height inflow wind speed of 9.4 m s−1 and potential temperature lapse rate of −0.024 K m−1 over the vertical extent of the domain. These wind speeds were selected because of their location on the power curve: above the cut-in wind speed (3 m s−1) and below the rated wind speed (12 m s−1), which is a range conducive for generating wakes and typical for general aviation flight conditions.
Horizontal slices of the horizontal wind speed (Fig. 3) show the wake characteristics at the near surface and bottom, center, and top of the rotor disk for both cases. The wake in the neutral case is approximately symmetric throughout the rotor disk (Fig. 3b–d), with the two-lobed profile becoming approximately Gaussian around 3 D (Fig. 3c). In the stable case, the wake exhibits a more asymmetric structure and veers with altitude (Fig. 3f–h) due to ambient background veer, as in other stable simulations of wakes (Lundquist et al., 2015). The horizontal extent and width of the wake also differ with stability. The stable conditions enable the wake to maintain a narrower structure and longer downwind presence, as opposed to the neutral conditions that form a comparatively more diffuse and shorter wake. The noise visible in both cases past 8 D is due to a combination of the coarser grid resolution and high sampling resolution in that region of the domain.
2.2 Data analysis
We use the simulation data to quantify potential roll hazards on hypothetical general aviation aircraft transecting the turbulent wake. The Cessna 172, a common general aviation aircraft, has a wingspan of 10 m, a planform area of 16 m2, and an aspect ratio of 7 (Cessna Aircraft Company, 2004). We represent the aircraft in the LES data as a 10 m line. The 1.25 m resolution of the data allows the line to be divided into eight segments – four points diverging from center to represent the two wings. We then use the LES wind vectors observed at each point on the aircraft to make calculations of the roll hazard metrics: rolling moment and rolling moment coefficient.
The rolling moment is computed from the lift distribution across the aircraft's wingspan. At each of the eight points on the aircraft, we sample the oncoming wind velocity vector nonparallel to the wing line. We assume a typical landing flight speed of a general aviation aircraft of 35 m s−1; the sampled wind vectors are relative to the movement of the aircraft. Thus, the total velocity vector, or true airspeed, is calculated at each segment: the nonparallel component of the wind vector impinging on the wing, added to the flight velocity. The elevation angle of each motion-relative total velocity vector forms an angle of attack α along the wing:
where w is the instantaneous component of velocity in z and V is the true instantaneous airspeed. From each angle of attack, a coefficient of lift Cl is derived – eight in total along the aircraft:
where Cl, 0 is the lift coefficient at zero angle of attack, Cl, b is the base coefficient of lift, is the lift perturbation, and 2πα is the chosen Cl–α curve provided by thin airfoil theory, an accurate idealization for most airfoils at small angles of attack.
The dimensional lift perturbation of each segment of the aircraft is then derived from the coefficient of lift:
where A is the planform area of the segment and ρ is the standard near-sea-level atmosphere density of 1.225 kg m−3 to reflect the altitude of interest for wake-transecting aircraft.
To calculate the rolling moment Mroll, we sum the eight lift values across the length of the wing
where N is the total number of points along the aircraft and ri is the distance from the center of the aircraft, which is positive (negative) to the left (right) of center from the perspective of the aircraft.
Finally, the rolling moment coefficient Croll is calculated by normalizing the rolling moment by the size of the aircraft
where is the true airspeed averaged across the eight segments, S is the total planform area of the aircraft, and b is the wing span of the aircraft, which we set to be 10 m to align with the general aviation Cessna 172 detailed earlier (Etkin and Reid, 1996).
The representation of an aircraft as a straight line within the LES data requires several assumptions. For simplicity, we assume a rectangular wing with some base lift equal to the aircraft weight, a result of defining a finite Cl, 0 and some constant aircraft angle of attack. We then only consider a lift perturbation from this base lift, as the remaining lift distribution is equal on both sides of the wing, contributing no rolling moment. We also note that our calculations assume that the aircraft and the flow field do not interact beyond the first-order effects on the aircraft and thus do not account for any aircraft motion response due to varying forces. A true depiction of aircraft motion would require a 6-degrees-of-freedom aircraft model and solver, which is more complicated than this study warrants. We recognize that by not accounting for the aircraft motion response, these calculations may omit cases when the changing wind components on the wing could result in higher rolling moments. However, such an additional series of calculations would introduce uncertainty because of the role of pilot response, and we argue that the wake roll hazards encountered (to be explained in Sect. 3) would allow a pilot to quickly correct against wake-turbulence-induced roll instead of allowing roll to escalate.
We define 540 total flight tracks through the LES data to sample the wind vectors and make the above calculations. These flights are conducted with two different orientations: down-wake transects and cross-wake transects. The down-wake group, 100 aircraft flight paths separated by 15 grid points (∼18.5 m) in y and z, is centered over the wake. We march these aircraft downwind from the turbine through the x extent of the domain. The cross-wake group is comprised of 44 rows and 10 columns of aircraft flight paths separated by 15 grid points (∼18.5 m) in x and z initially positioned at , which is the furthest west point of the domain. We march these aircraft through the y extent of the domain, perpendicular to the wake. The cross-wake aircraft descend at a 3∘ angle to emulate a typical landing pattern. At each advancing increment in the transect (682 points down-wake, 247 points cross-wake), the rolling moment (Eq. 4) and rolling moment coefficient (Eq. 5) are calculated for all 540 aircraft, yielding 176 880 instances for each roll hazard calculation. We define one additional down-wake flight track far outside of the wake (, z=100 m) to serve as a constant, wake-free flight path for comparison. The aircraft do not interact with each other nor modify the flow field as they transect the wake. All calculations are made during a single one-second time step where the aircraft fly across a frozen domain. This process is then repeated for each of the 100 time steps available, yielding 17 688 000 total hazard calculations.
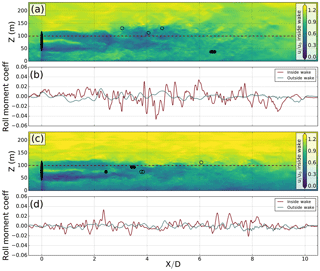
Figure 4Comparison of rolling moment coefficient (b, d) between the flight path inside (red) and outside (blue) of the turbine wake in the neutral (a, b) and stable (c, d) cases. The vertical cross section of the downwind component of the wind velocity u, scaled by inflow wind speed u0, 7.4 and 9.4 m s−1 in neutral and stable conditions, respectively, at the turbine location (y=0; a, c) is contoured for reference, over which the height of the flight paths (z=100 m) is traced in a red dashed line. The dots represent the downwind and vertical location of the three highest rolling moment coefficients experienced by the down-wake (black) and cross-wake (empty) array of aircraft.
We first analyze the turbine wake impacts on a hypothetical general aviation aircraft by comparing roll hazard calculations of a sample flight track outside of the wake to those within the wake. Rolling moment coefficient (Eq. 5) is calculated for an aircraft inside and outside the wake for the neutral (Fig. 4b) and stable (Fig. 4d) cases to categorize each moment as a “low”, “medium”, or “high” hazard as used in the Mulinazzi and Zheng (2014) and Wang et al. (2015) assessments of wake roll hazards. These assessment criteria are based on the maximum rolling moment that the aileron on a typical aircraft can generate to counteract a moment induced by the wake field (Wang et al., 2015). A wake-induced rolling moment coefficient above 0.28 is considered a high hazard, between 0.1 and 0.28 a medium hazard, and below 0.1 a low hazard. As expected, in both stability conditions, the aircraft inside the wake experiences higher values of rolling moment coefficient, indicating increased turbulence of the turbine wake. However, rolling moment coefficients experienced in both stability cases for the aircraft inside the wake remain within the low hazard threshold of .
While these sample aircraft flight tracks in Fig. 4b, d show an example for one location in the wake, the LES data set allows us to calculate more instances of roll hazards throughout the entire wake. More conclusive results can be seen by assessing the 540 flight paths down and across the wake spanning all 100 s of data. We summarize occurrences of roll hazard calculations via histograms of neutral (Fig. 5a, b) and stable (Fig. 5c, d) cases and down-wake (Fig. 5a, c) and cross-wake (Fig. 5b, d) transects as a function of the downwind distance from the turbine.
In all transects and stabilities, over 99.99 % of all calculations exist within the low hazard threshold. Table 1 lists detailed counts of instances classified as medium hazards (). The stable cross-wake configuration has the greatest fraction of medium hazards at 188 out of 10 868 000. Across all cases, no moments reach the high hazard threshold. Further, the vast majority of calculated roll hazards are even smaller than the maximum low hazard limit () and are contained within (Fig. 5). The decreasing frequency of rolling moment coefficients greater than 0.02 beyond 8 D suggests that roll hazards decrease with downstream distance at this point (Fig. 5). While we hypothesized the stable case would generate more hazardous rolling moment coefficients, we find similar fractions of medium hazards in the stable conditions as in neutral (Fig. 5). Even though stable conditions enable the wake to persist longer downwind than in convective conditions, they do not increase turbulence that would pose hazards for general aviation aircraft.
The largest roll hazards, as indicated by Croll, occur most frequently about 5 D downwind from the turbine (Fig. 5). The three highest roll hazards in the neutral, down-wake case () all occur in a sequential line downwind near 6.5 D in the bottom-left quadrant of the rotor disk as looking downwind (black circles in Fig. 4a). These rolling moment coefficients are all positive, indicating clockwise rotation about the aircraft's longitudinal axis when looking downwind. In the neutral, cross-wake case, the three highest rolling moments () occur between 3 and 4.5 D in the top-right quadrant of the rotor disk (empty circles in Fig. 4a). For the stable, down-wake case, the highest two roll hazards (, −0.12) occur at 3.5 D downwind, in the top-left quadrant of the rotor (Fig. 4c). The next highest hazard () occurs 2.5 D downwind, differing from the prior hazards by residing in the bottom-left quadrant of the rotor (Fig. 4c). The largest hazards across all cases occur in the stable, cross-wake case at Croll=0.14, 0.14, 0.136, with the first two in the bottom-right quadrant of the rotor disk and the third in the top left (empty circles in Fig. 4c). All of these peak hazards are located in the high-shear zone at the edge of the wake between 3 and 7 D downwind from the turbine (and not closer to the turbine, e.g., 0.5–1 D), which suggests that the rolling moment is more influenced by horizontal shear in the flow than by wake rotation.
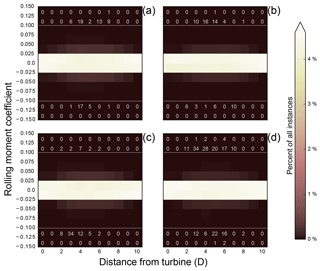
Figure 5Two-dimensional histograms of all calculations of rolling moment coefficient experienced by all aircraft in the neutral (a, b) and stable (c, d) cases for down-wake (a, c) and cross-wake (b, d) transects as a function of the downwind distance from the turbine (in rotor diameters D). “Non-low” hazards () are explicitly totalled in their respective bins.
As wind energy development increases in the vicinity of general aviation airports, concerns for turbine-wake-induced roll hazards on aircraft grow. Of particular concern is the rolling moment, the aerodynamic force applied at a distance from an aircraft's center of mass that causes the aircraft to undergo angular acceleration about its roll axis. Using LES of stable and neutral flow past a utility-scale wind turbine, we quantify the roll hazards experienced by general aviation aircraft transecting the wake.
We represent a typical general aviation aircraft in the LES data as a 10 m line comprised of eight segments that are 1.25 m in length. At each point along the aircraft, we sample the LES wind vectors to calculate rolling moment and the rolling moment coefficient. We define 540 flight tracks to march through the wake to make the roll hazard calculations. The flight tracks have down-wake and cross-wake orientations and extend through the entire downwind 682 points and 247 points, respectively, yielding 176 880 calculations of a roll hazard for each stability case for each time step of data.
The rolling moment coefficient serves as our primary roll hazard metric, as it normalizes the rolling moment on the aircraft by the aircraft size, shape, and airspeed to produce a standard hazard index. This index has been classified by past studies into thresholds for low, medium, and high roll hazards. When comparing a sample flight path outside of the wake's influence to one within the wake center, we find that an aircraft flying within the wake experiences higher rolling moment coefficient than an aircraft flying in a wake-free environment. However, analyses of all 540 flight paths through the wake reveal that over 99.99 % of hazard calculations remained within the low criterion in both neutrally stratified and stably stratified conditions across 100 s of data. In neutral conditions, the largest of these hazards are classified as medium hazards and exist 6.5 D downwind of the turbine in the bottom-left portion of the rotor disk. The highest hazards in the stable case also remained within the medium threshold and are located in two separate regions of the wake: approximately 4 D downwind in the bottom-right quadrant of the rotor and 6 D downwind in the top-left quadrant of the rotor.
Our calculated roll hazards differ from those in Mulinazzi and Zheng (2014), who used a helical vortex model scaled up from wind tunnel measurements to find significant roll hazards far downwind from a wind turbine. Conversely, our results using an actuator-line representation of a wind turbine in the SOWFA LES model rarely surpass the low roll hazard threshold. Rather, our results agree with those of Wang et al. (2015, 2017), whose calculations are computed from the Beddoes circulation formula (Madsen and Rasmussen, 2004), as opposed to the wind tunnel extrapolation in Mulinazzi and Zheng (2014). Past successes in turbine wake modeling using LES actuator-line methods can in part validate our results (e.g., Churchfield et al., 2012; Jha et al., 2015; Nilsson et al., 2015).
This study presents a simple method for quantifying turbine-wake-induced roll hazards on general aviation aircraft and is constrained by the assumptions made to represent an aircraft within LES data of a single turbine wake. While we have shown that individual wakes in neutrally stratified and stably stratified conditions are unlikely to pose hazards to general aviation aircraft, interactions between wakes could also be explored. Future simulations of wind plants that allow for wake interaction (as in Vanderwende et al., 2016) and larger turbine types could be useful. Our conclusions are drawn from rolling moment calculations, though additional calculations of yawing and pitching moments could also be beneficial. Our results may also be supported by field tests, in which tethersondes (Lundquist and Bariteau, 2015) or unmanned aircraft vehicles (Baserud et al., 2014; Kocer et al., 2012; Lawrence and Balsley, 2013) would fly through the wake of a real utility-scale turbine during variable conditions to directly measure roll hazards. Future studies could integrate simulations like these with flight simulators to understand the coupling of the atmospheric behavior to pilot response for an integrated assessment of roll hazard, as in Wang et al. (2015).
Model output and code from this study are stored on the University of Colorado's PetaLibrary and are available from the authors upon request. Parsed data used to display results are available for immediate download on GitHub (https://doi.org/10.5281/zenodo.1475224; Tomaszewski, 2018).
JKL and PJM conceived the research. MJC designed and carried out the large-eddy simulations. JMT designed and executed the method for calculating roll hazards with significant input from PJM and MJC. JMT wrote the paper with significant contributions from JKL. All co-authors contributed to refining the text and conclusions.
The authors declare that they have no conflict of interest.
This work was supported by a seed grant from Renewable and Sustainable
Energy Institute with cooperation from NREL. NREL is a national laboratory of
the United States Department of Energy, Office of Energy Efficiency and Renewable
Energy, operated by the Alliance for Sustainable Energy, LLC. JMT was
partially supported by an NSF Graduate Research Fellowship under grant number
1144083. Roll hazard calculations were conducted using the Extreme Science
and Engineering Discovery Environment (XSEDE), which is supported by National
Science Foundation grant number ACI1053575. JKL's effort was supported by an
agreement with NREL under APUP UGA-0-41026-65. The United States Government retains
and the publisher, by accepting the article for publication, acknowledges
that the United States Government retains a nonexclusive, paid-up, irrevocable,
worldwide license to publish or reproduce the published form of this work, or
allow others to do so, for United States Government purposes.
Edited by: Luciano Castillo
Reviewed by: three anonymous referees
Abkar, M., Sharifi, A., and Porté-Agel, F.: Wake flow in a wind farm during a diurnal cycle, J. Turbul., 17, 420–441, https://doi.org/10.1080/14685248.2015.1127379, 2016. a
Aitken, M. L., Banta, R. M., Pichugina, Y. L., and Lundquist, J. K.: Quantifying Wind Turbine Wake Characteristics from Scanning Remote Sensor Data, J. Atmos. Ocean. Tech., 31, 765–787, https://doi.org/10.1175/JTECH-D-13-00104.1, 2014a. a
Aitken, M. L., Kosović, B., Mirocha, J. D., and Lundquist, J. K.: Large eddy simulation of wind turbine wake dynamics in the stable boundary layer using the Weather Research and Forecasting Model, J. Renew. Sustain. Ener., 6, 033137, https://doi.org/10.1063/1.4885111, 2014b. a, b
Baker, R. W. and Walker, S. N.: Wake measurements behind a large horizontal axis wind turbine generator, Sol. Energy, 33, 5–12, 1984. a, b
Bserud, L., Flügge, M., Bhandari, A., and Reuder, J.: Characterization of the SUMO Turbulence Measurement System for Wind Turbine Wake Assessment, Enrgy Proced., 53, 173–183, https://doi.org/10.1016/j.egypro.2014.07.226, 2014. a
Bhaganagar, K. and Debnath, M.: Implications of Stably Stratified Atmospheric Boundary Layer Turbulence on the Near-Wake Structure of Wind Turbines, Energies, 7, 5740–5763, https://doi.org/10.3390/en7095740, 2014. a, b
Bodini, N., Lundquist, J. K., and Newsom, R. K.: Estimation of turbulence dissipation rate and its variability from sonic anemometer and wind Doppler lidar during the XPIA field campaign, Atmos. Meas. Tech., 11, 4291–4308, https://doi.org/10.5194/amt-11-4291-2018, 2018. a
CAA: CAA Policy and Guidelines on Wind Turbines, Stationery Office, google-Books-ID: 4PNZlwEACAAJ, 2012. a
Calaf, M., Meneveau, C., and Meyers, J.: Large eddy simulation study of fully developed wind-turbine array boundary layers, Phys. Fluids, 22, 015110, https://doi.org/10.1063/1.3291077, 2010. a, b, c
Cessna Aircraft Company: 172S Skyhawk – Information Manual, available at: http://www.gaceflyingclub.com/Member Download/172S Skyhawk Information Manual Searchable.pdf (last access: 29 October 2018), 2004. a
Chamorro, L. P. and Porté-Agel, F.: A Wind-Tunnel Investigation of Wind-Turbine Wakes: Boundary-Layer Turbulence Effects, Bound.-Lay. Meteorol., 132, 129–149, https://doi.org/10.1007/s10546-009-9380-8, 2009. a
Churchfield, M. J. and Lee, S.: SOWFA | NWTC Information Portal, available at: https://nwtc.nrel.gov/SOWFA (last access: 29 October 2018), 2014. a
Churchfield, M. J., Lee, S., Michalakes, J., and Moriarty, P. J.: A numerical study of the effects of atmospheric and wake turbulence on wind turbine dynamics, J. Turbul., 13, N14, https://doi.org/10.1080/14685248.2012.668191, 2012. a, b, c, d
Churchfield, M. J., Schreck, S. J., Martínez, L. A., Meneveau, C., and Spalart, P. R.: An Advanced Actuator Line Method for Wind Energy Applications and Beyond, in: 35th Wind Energy Symposium, American Institute of Aeronautics and Astronautics, Grapevine, Texas, https://doi.org/10.2514/6.2017-1998, 2017. a
EIA: United States Energy Information Administration – Electricity Data, available at: http://www.eia.gov/electricity/monthly (last access: 29 October 2018), 2017. a
Englberger, A. and Dörnbrack, A.: Impact of the Diurnal Cycle of the Atmospheric Boundary Layer on Wind-Turbine Wakes: A Numerical Modelling Study, Bound.-Lay. Meteorol., 166, 423–448, https://doi.org/10.1007/s10546-017-0309-3, 2018. a
Etkin, B. and Reid, L. D.: Dynamics of flight: stability and control, Wiley, New York, 3rd edn., 1996. a, b
Fleming, P., Gebraad, P., van Wingerden, J. W., Lee, S., Churchfield, M. J., Scholbrock, A., Michalakes, J., Johnson, K., and Moriarty, P. J.: SOWFA Super-Controller: A High-Fidelity Tool for Evaluating Wind Plant Control Approaches, Tech. Rep. NREL/CP-5000-57175, National Renewable Energy Laboratory (NREL), Golden, CO, 2013. a
Gerz, T., Holzäpfel, F., Frech, M., Kober, K., Dengler, K., Rahm, S., Gerling, W., and Scharnweber, A.: The Wake Vortex Prediction and Monitoring System WSVBS Part II: Performance and ATC Integration at Frankfurt Airport, Air Traffic Control Quarterly, 17, 323–346, https://doi.org/10.2514/atcq.17.4.323, 2009. a
Hamilton, P.: State Aviation Journal – Spring 2014, Issuu, available at: https://issuu.com/stateaviationjournal/docs/spring_magazine_2014 (last access: 29 October 2018), 2014. a
Hancock, P. E. and Zhang, S.: A Wind-Tunnel Simulation of the Wake of a Large Wind Turbine in a Weakly Unstable Boundary Layer, Bound.-Lay. Meteorol., 156, 395–413, https://doi.org/10.1007/s10546-015-0037-5, 2015. a
Ho, C. K., Lambert, J. R., Vigilante, M. L., DeVita, P. M., and Zhang, Y.: Guidebook for Energy Facilities Compatibility with Airports and Airspace | Chapter 3 – Energy Technologies and Aviation Safety Impacts, The National Academies Press, Washington, D.C., 2014. a
Holzäpfel, F., Gerz, T., and Baumann, R.: Aircraft wake vortices – prediction and mitigation, PAMM, 7, 1100801–1100802, https://doi.org/10.1002/pamm.200700569, 2007. a
Iungo, G. V., Wu, Y.-T., and Porté-Agel, F.: Field Measurements of Wind Turbine Wakes with Lidars, J. Atmos. Ocean. Tech., 30, 274–287, https://doi.org/10.1175/JTECH-D-12-00051.1, 2012. a
Ivanell, S., Mikkelsen, R., Sørensen, J. N., and Henningson, D.: Stability analysis of the tip vortices of a wind turbine, Wind Energy, 13, 705–715, https://doi.org/10.1002/we.391, 2010. a
Jha, P., Duque, E., Bashioum, J., and Schmitz, S.: Unraveling the Mysteries of Turbulence Transport in a Wind Farm, Energies, 8, 6468–6496, https://doi.org/10.3390/en8076468, 2015. a, b
Jimenez, A., Crespo, A., Migoya, E., and Garcia, J.: Advances in large-eddy simulation of a wind turbine wake, J. Phys. Conf. Ser., 75, 012041, 2007. a
Käsler, Y., Rahm, S., Simmet, R., and Kühn, M.: Wake Measurements of a Multi-MW Wind Turbine with Coherent Long-Range Pulsed Doppler Wind Lidar, J. Atmos. Ocean. Tech., 27, 1529–1532, https://doi.org/10.1175/2010JTECHA1483.1, 2010. a
Kocer, G., Chokani, N., and Abhari, R.: Wake Structure of a 2 MW Wind Turbine Measured Using an Instrumented UAV, 50th AIAA Aerospace Sciences Meeting, Volume: AIAA Paper 2012-0231, https://doi.org/10.2514/6.2012-231, 2012. a
Lawrence, D. A. and Balsley, B. B.: High-Resolution Atmospheric Sensing of Multiple Atmospheric Variables Using the DataHawk Small Airborne Measurement System, J. Atmos. Ocean. Tech., 30, 2352–2366, https://doi.org/10.1175/JTECH-D-12-00089.1, 2013. a
Lignarolo, L., Ragni, D., Scarano, F., Simão Ferreira, C., and van Bussel, G.: Tip-vortex instability and turbulent mixing in wind-turbine wakes, J. Fluid Mech., 781, 467–493, https://doi.org/10.1017/jfm.2015.470, 2015. a
Lissaman, P. B. S.: Energy Effectiveness of Arbitrary Arrays of Wind Turbines, J. Energy, 3, 323–328, https://doi.org/10.2514/3.62441, 1979. a
Lu, H. and Porté-Agel, F.: Large-eddy simulation of a very large wind farm in a stable atmospheric boundary layer, Phys. Fluids, 23, 065101, https://doi.org/10.1063/1.3589857, 2011. a
Lundquist, J. K. and Bariteau, L.: Dissipation of Turbulence in the Wake of a Wind Turbine, Bound.-Lay. Meteorol., 154, 229–241, https://doi.org/10.1007/s10546-014-9978-3, 2015. a
Lundquist, J. K., Churchfield, M. J., Lee, S., and Clifton, A.: Quantifying error of lidar and sodar Doppler beam swinging measurements of wind turbine wakes using computational fluid dynamics, Atmos. Meas. Tech., 8, 907–920, https://doi.org/10.5194/amt-8-907-2015, 2015. a, b
Madsen, H. E. and Rasmussen, F.: A near wake model for trailing vorticity compared with the blade element momentum theory, Wind Energy, 7, 325–341, https://doi.org/10.1002/we.131, 2004. a, b
Magnusson, M. and Smedman, A.: Influence of atmospheric stability on wind turbine wakes, J. Wind Eng. Ind. Aerod., 80, 147–167, https://doi.org/10.1016/S0167-6105(98)00125-1, 1994. a
Marjanovic, N., Mirocha, J. D., Kosović, B., Lundquist, J. K., and Chow, F. K.: Implementation of a generalized actuator line model for wind turbine parameterization in the Weather Research and Forecasting model, J. Renew. Sustain. Ener., 9, 063308, https://doi.org/10.1063/1.4989443, 2017. a
Martínez-Tossas, L. A., Churchfield, M. J., and Meneveau, C.: Large eddy simulation of wind turbine wakes: detailed comparisons of two codes focusing on effects of numerics and subgrid modeling, J. Phys. Conf. Ser., 625, 012024, https://doi.org/10.1088/1742-6596/625/1/012024, 2015. a
Martínez-Tossas, L. A., Churchfield, M. J., and Meneveau, C.: Optimal smoothing length scale for actuator line models of wind turbine blades based on Gaussian body force distribution: Wind energy, actuator line model, Wind Energy, 20, 1083–1096, https://doi.org/10.1002/we.2081, 2017. a
Mendoza, I., Hur, J., Thao, S., and Curtis, A.: Power Performance Test Report for the U.S. Department of Energy 1.5-Megawatt Wind Turbine, NREL/TP-5000-63684, available at: https://www.nrel.gov/docs/fy15osti/63684.pdf (last access: 29 October 2018), 2015. a
Mirocha, J. D., Kosović, B., Aitken, M. L., and Lundquist, J. K.: Implementation of a generalized actuator disk wind turbine model into the weather research and forecasting model for large-eddy simulation applications, J. Renew. Sustain. Ener., 6, 013104, https://doi.org/10.1063/1.4861061, 2014. a, b, c
Mirocha, J. D., Rajewski, D. A., Marjanovic, N., Lundquist, J. K., Kosović, B., Draxl, C., and Churchfield, M. J.: Investigating wind turbine impacts on near-wake flow using profiling lidar data and large-eddy simulations with an actuator disk model, J. Renew. Sustain. Ener., 7, 043143, https://doi.org/10.1063/1.4928873, 2015. a, b
Mulinazzi, T. E. and Zheng, Z. C.: Wind Farm Turbulence Impacts on General Aviation Airports in Kansas, K-TRAN: KU-13-6, available at: http://dmsweb.ksdot.org/AppNetProd/docpop/docpop.aspx?clienttype=html&docid=9011677 (last access: 29 October 2018), 2014. a, b, c, d, e
Nilsson, K., Shen, W. Z., Sørensen, J. N., Breton, S., and Ivanell, S.: Validation of the actuator line method using near wake measurements of the MEXICO rotor: Validation of the ACL method, Wind Energy, 18, 499–514, https://doi.org/10.1002/we.1714, 2015. a, b
OpenCFD: The Open Source CFD Toolbox, User's Manual, Version 1.7.1, OpenCFD, Reading, Berkshire, UK, 2016. a
OurAirports: Open data @ OurAirports, available at: http://ourairports.com/data/ (last access: 29 October 2018), 2016. a
Porté-Agel, F., Wu, Y., Lu, H., and Conzemius, R. J.: Large-eddy simulation of atmospheric boundary layer flow through wind turbines and wind farms, J. Wind Eng. Ind. Aerod., 99, 154–168, https://doi.org/10.1016/j.jweia.2011.01.011, 2011. a
Rajewski, D. A., Takle, E. S., Lundquist, J. K., Oncley, S., Prueger, J. H., Horst, T. W., Rhodes, M. E., Pfeiffer, R., Hatfield, J. L., Spoth, K. K., and Doorenbos, R. K.: Crop Wind Energy Experiment (CWEX): Observations of Surface-Layer, Boundary Layer, and Mesoscale Interactions with a Wind Farm, B. Am. Meteorol. Soc., 94, 655–672, https://doi.org/10.1175/BAMS-D-11-00240.1, 2013. a
Rajewski, D. A., Takle, E. S., Lundquist, J. K., Prueger, J. H., Pfeiffer, R. L., Hatfield, J. L., Spoth, K. K., and Doorenbos, R. K.: Changes in fluxes of heat, H2O, and CO2 caused by a large wind farm, Agr. Forest Entomol., 194, 175–187, https://doi.org/10.1016/j.agrformet.2014.03.023, 2014. a
Rajewski, D. A., Takle, E. S., Prueger, J. H., and Doorenbos, R. K.: Toward understanding the physical link between turbines and microclimate impacts from in situ measurements in a large wind farm: Microclimate With Turbines ON Versus OFF, J. Geophys. Res.-Atmos., 121, 13392–13414, https://doi.org/10.1002/2016JD025297, 2016. a
Rhodes, M. E. and Lundquist, J. K.: The Effect of Wind-Turbine Wakes on Summertime US Midwest Atmospheric Wind Profiles as Observed with Ground-Based Doppler Lidar, Bound.-Lay. Meteorol., 149, 85–103, https://doi.org/10.1007/s10546-013-9834-x, 2013. a
Sanderse, B., van der Pijl, S. P., and Koren, B.: Review of computational fluid dynamics for wind turbine wake aerodynamics, Wind Energy, 14, 799–819, https://doi.org/10.1002/we.458, 2011. a
Sforza, P. M., Sheerin, P., and Smorto, M.: Three-Dimensional Wakes of Simulated Wind Turbines, AIAA J., 19, 1101–1107, https://doi.org/10.2514/3.60049, 1981. a
Smith, C. M., Barthelmie, R. J., and Pryor, S. C.: In situ observations of the influence of a large onshore wind farm on near-surface temperature, turbulence intensity and wind speed profiles, Environ. Res. Lett., 8, 034006, https://doi.org/10.1088/1748-9326/8/3/034006, 2013. a
Snel, H., Schepers, J. G., and Montgomerie, B.: The MEXICO project (Model Experiments in Controlled Conditions): The database and first results of data processing and interpretation, J. Phys. Conf. Ser., 75, 012014, https://doi.org/10.1088/1742-6596/75/1/012014, 2007. a
Sørensen, J. N. and Shen, W. Z.: Numerical Modeling of Wind Turbine Wakes, J. Fluid. Eng., 124, 393–399, https://doi.org/10.1115/1.1471361, 2002. a, b, c
Toloui, M., Chamorro, L. P., and Hong, J.: Detection of tip-vortex signatures behind a 2.5 MW wind turbine, J. Wind Eng. Ind. Aerod., 143, 105–112, https://doi.org/10.1016/j.jweia.2015.05.001, 2015. a
Tomaszewski, J. M.: First release of WES-2018-42-roll-hazards (Version v1.0), Zenodo, https://doi.org/10.5281/zenodo.1475224, 2018. a
Troldborg, N., Sørensen, J. N., and Mikkelsen, R. F.: Numerical simulations of wake characteristics of a wind turbine in uniform inflow, Wind Energy, 13, 86–99, https://doi.org/10.1002/we.345, 2010. a
USGS: Other Energy Studies | Wind, USGS–ERP, available at: https://energy.usgs.gov/OtherEnergy/WindEnergy.aspx#4312358-data (last access: 29 October 2018), 2014. a
van der Wall, B. G. and Lehmann, P. H.: Helicopter Rotor Trim and Blade Motion Perturbations Caused by Wake Vortices of Wind Turbines and Fixed Wing Aircraft, in: 6th ARF & Heli Japan 2017, 1–21, available at: https://elib.dlr.de/112961/ (last access: 29 October 2018), 2017. a
van der Wall, B. G., Fischenberg, D., Lehmann, P. H., and van der Wall, L. B.: Impact of Wind Energy Rotor Wakes on Fixed-Wing Aircraft and Helicopters, in: 42nd European Rotorcraft Forum, 1–28, available at: https://elib.dlr.de/104396/ (last access: 29 October 2018), 2016. a
Vanderwende, B. J., Kosović, B., Lundquist, J. K., and Mirocha, J. D.: Simulating effects of a wind-turbine array using LES and RANS: Simulating turbines using LES and RANS, J. Adv. Model Earth Sy., 8, 1376–1390, https://doi.org/10.1002/2016MS000652, 2016. a, b, c
Vermeer, L., Sørensen, J., and Crespo, A.: Wind turbine wake aerodynamics, Prog. Aerosp. Sci., 39, 467–510, https://doi.org/10.1016/S0376-0421(03)00078-2, 2003. a, b, c
Wang, Y., White, M., and Barakos, G.: Wind Turbine Wake Encounter Study, Technical Report, available at: https://www.liverpool.ac.uk/media/livacuk/flightscience/projects/cfd/wakeencounter/caa_wind_turbine_report.pdf (last access: 29 October 2018), 2015. a, b, c, d, e, f, g, h
Wang, Y., White, M., and Barakos, G. N.: Wind-Turbine Wake Encounter by Light Aircraft, J. Aircraft, 54, 367–370, https://doi.org/10.2514/1.C033870, 2017. a, b, c
Williams, M.: Wind farms could endanger small aircraft, study says | The Kansas City Star, available at: https://www.kansascity.com/news/local/article336745/Wind-farms-could-endanger-small-aircraft-study-says.html (last access: 29 October 2018), 2014. a
Yang, Z., Sarkar, P., and Hu, H.: Visualization of the tip vortices in a wind turbine wake, J. Visual., 15, 39–44, https://doi.org/10.1007/s12650-011-0112-z, 2012. a, b
Zhou, L., Tian, Y., Baidya Roy, S., Thorncroft, C., Bosart, L. F., and Hu, Y.: Impacts of wind farms on land surface temperature, Nat. Clim. Change, 2, 539–543, https://doi.org/10.1038/nclimate1505, 2012. a