the Creative Commons Attribution 4.0 License.
the Creative Commons Attribution 4.0 License.
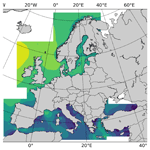
Europe's offshore winds assessed with synthetic aperture radar, ASCAT and WRF
Andrea N. Hahmann
Tobias Ahsbahs
Ioanna Karagali
Tija Sile
Merete Badger
Jakob Mann
Europe's offshore wind resource mapping is part of the New European Wind Atlas (NEWA) international consortium effort. This study presents the results of analysis of synthetic aperture radar (SAR) ocean wind maps based on Envisat and Sentinel-1 with a brief description of the wind retrieval process and Advanced Scatterometer (ASCAT) ocean wind maps. The wind statistics at 10 and 100 m above mean sea level (a.m.s.l.) height using an extrapolation procedure involving simulated long-term stability over oceans are presented for both SAR and ASCAT. Furthermore, the Weather Research and Forecasting (WRF) offshore wind atlas of NEWA is presented. This has 3 km grid spacing with data every 30 min for 30 years from 1989 to 2018, while ASCAT has 12.5 km and SAR has 2 km grid spacing. Offshore mean wind speed maps at 100 m a.m.s.l. height from ASCAT, SAR, WRF and ERA5 at a European scale are compared. A case study on offshore winds near Crete compares SAR and WRF for flow from the north, west and all directions.
The paper highlights the ability of the WRF model to simulate the overall European wind climatology and the near-coastal winds constrained by the resolution of the coastal topography in the WRF model simulations.
- Article
(3935 KB) - Full-text XML
- BibTeX
- EndNote
The extraction of energy from wind is part of the clean energy transition. It supports society to reach the objectives of the Paris Climate Change agreement and the Sustainable Development Goals. Wind energy in Europe provided 14 % of total electricity consumption in 2018. This share will increase in coming years. By the end of 2018, the installed offshore capacity reached 18.5 GW, which is approximately 10 % of Europe's total wind energy capacity (Wind Europe, 2019).
Beyond the beneficial impact on reducing carbon dioxide emissions, the offshore wind energy industry is a significant economical factor. According to the Organisation for Economic Co-operation and Development (OECD, 2016), the gross value added of all ocean-based industries globally will double from USD 1.5 trillion in 2010 to USD 3 trillion by 2030. Offshore wind energy has the highest relative growth rate of the ocean-based industries. In Europe alone, the investments in 2018 in new offshore wind amounted to EUR 10.3 billion, a 37 % increase from 2017 (Wind Europe, 2019).
Many countries in Europe have operating offshore wind farms. The North Sea accounts for 70 % of all installed offshore wind capacity in Europe, followed by the Irish Sea (16 %), the Baltic Sea (12 %) and the Atlantic Ocean (2 %). The longest distance from shore of operating wind turbines exceeds 100 km while permits are given for installation as far as 200 km offshore (Wind Europe, 2019). The expectation is that offshore wind energy will expand to more European seas and that new wind farms are erected in clusters, which already exist in parts of the North Sea (4C Offshore, 2019).
The New European Wind Atlas (NEWA) project focused on experimental campaigns across Europe in different terrain types. These experiments provide unique data for validation of wind models (Petersen et al., 2014; Mann et al., 2017; Witze, 2017). Two of the field experiments are relevant for offshore wind resource mapping. The first is the coastal experiment RUNE with a floating lidar system, three long-range horizontally scanning wind lidars and several vertical wind profiling lidars installed at the North Sea coastline (Floors et al., 2016) close to the tall meteorological masts at Høvsøre in Denmark (Peña et al., 2015). The second is the wind profiling lidar installed at the ferry link between Kiel and Klaipėda in the Baltic Sea (Gottschall et al., 2018). The two experiments had a duration of around 6 months. In addition to the dedicated experiments, several years of meteorological observations from tall offshore masts all located in the northern European seas are used in preparation of the NEWA offshore wind atlas.
The NEWA project (2015–2019) produced the novel state-of-the-art offshore wind atlas for European seas covering a minimum distance up to 100 km offshore and the entire North Sea and Baltic Sea, excluding Iceland. In addition to the entire wind atlas simulated using the Weather, Research and Forecasting (WRF) model (Hahmann et al., 2020), satellite synthetic aperture radar (SAR) and Advanced Scatterometer (ASCAT) ocean winds are also processed and analysed for wind resource assessment.
The overall objective of the study is to present the new European Offshore Wind Atlas and to examine the similarities and differences of wind maps based on ASCAT, SAR and the WRF model. The study focuses on how to use satellite observations for model comparison beyond single cases and specifically to investigate how different the 100 m a.m.s.l. mean winds are based on ASCAT, SAR and WRF.
In the planning phase of a wind farm project there is need for information on the wind resource (Emeis, 2012; Landberg, 2016; Petersen and Troen, 2012). The methodologies for offshore wind resource assessment rely on wind observations from offshore meteorological masts, wind lidar, SODAR (sound detection and ranging), satellite images and modelling (Sempreviva et al., 2008). The first atlas of European wind resources covered only land (Troen and Petersen, 1989) and was later extended to offshore (Petersen, 1992). Modelling of wind resources has a long tradition starting with the above-mentioned wind atlas. Recent offshore model-based wind atlases for the European seas include the German Bight (Jimenez et al., 2006), the Mediterranean Sea (Lavignini et al., 2006), the UK (UK Renewables Atlas, 2008; The Crowne Estate, 2015), the North Sea (Berge et al., 2009), the European seas (EEA, 2009), the south Baltic Sea (Peña et al., 2011), the Baltic and North seas (Hahmann et al., 2015), and the Dutch waters (KNMI, 2019).
Offshore wind resource assessment based on in situ meteorological wind observations in the Baltic and North seas (see review in Sempreviva et al., 2008), Italy (Casale et al., 2010), and Malta (Farrugia and Sant, 2016) provides local information. Furthermore, the meteorological observations are useful for comparison to model results to select suitable atmospheric model setup and to assess the model performance (Jimenez et al., 2006; Berge et al., 2009; Hahmann et al., 2015).
Satellite remote sensing used to assess offshore wind resources for the European seas includes scatterometer and SAR measurements. Scatterometer estimates have been validated for the Mediterranean Sea with buoy data (Furevik et al., 2011) and for the northern European seas with meteorological mast data (Karagali et al., 2013a, 2014, 2018a). Soukissian et al. (2017) used a blended satellite product based on six different satellites for the Mediterranean Sea and compared to buoy data.
Satellite SAR was used for resource assessment for the North Sea (Hasager et al., 2005, 2015b; Christiansen et al., 2016; Badger et al., 2010) and the Baltic Sea (Hasager et al., 2011; Badger et al., 2016) and was compared to meteorological mast data. Coastal mast data and mesoscale model results were compared to SAR-based wind resource estimates for the Icelandic waters (Hasager et al., 2015a). Scatterometer data (ASCAT) were also compared to WRF mesoscale model results in all the European seas (Karagali et al., 2018a, b).
There is potential to also compare model results and satellite data to wind profiling lidar (light detection and ranging) data at offshore platforms (Hasager et al., 2013) and floating wind profile lidar systems (OWA, 2018; Bischoff et al., 2018). These are local point data similar to buoy data and meteorological mast data. Recently, new technological advancements have provided opportunities for horizontal spatial data comparison, e.g. the dual Doppler radar (Nygaard and Newcombe, 2018; Valldecabres et al., 2018). Three other spatial data types are horizontally scanning lidar, long rows of turbines providing SCADA (supervisory control and data acquisition) data and ship-mounted vertical profiling lidar.
Recently, offshore winds observed with long-range scanning lidar at a coastal site at the North Sea (Floors et al., 2016) were compared to SAR winds and showed good comparison within 2 to 5 km from the North Sea coastline. The good agreement was unexpected because the geophysical model function (GMF) used to retrieve winds from SAR is valid in open ocean and not near the coast. The conclusion of the study is that SAR winds are mapped well as close as 2 km from the coastline at the site investigated (Ahsbahs et al., 2017). Documentation at a more complex coastline remains open.
Another recent study found that the SAR-based winds compare slightly better than mesoscale model results to the wind speed observed at a 20 km long row of turbines. The turbines are operating in an area with a strong horizontal wind gradient along the coast (Ahsbahs et al., 2018).
The third novel spatial comparison method was based on a vertical profiling lidar installed on board a ferry sailing daily across the Baltic Sea for several hundred kilometres; measurements compared well to mesoscale model results (Gottschall et al., 2018). Data near the harbours were excluded from the analysis. The WRF mesoscale model results are generally better offshore than near coastlines due to the differences between land and sea influencing the atmospheric flow (Hahmann et al., 2010, 2015; Floors et al., 2018). The flow is more complex near the coastline than further offshore and fine-scale structures such as land–sea breeze, not resolved by the model, may prevail.
The presentation of methodology for wind mapping based on ASCAT, SAR and WRF is given in Sect. 3. Section 4 presents the results for all the European seas from ASCAT, SAR and WRF, their inter-comparisons, and cross-comparison to ERA5. Section 5 is a case study of offshore winds around western Crete using SAR and WRF, thus providing insight into specific details on the two types of data. Section 6 covers discussion and perspectives regarding the results, followed by conclusions in Sect. 7.
3.1 Area of interest and time period
The offshore part of NEWA covers the European Union, associated states, and Turkey from the coastline and at least 100 km offshore. For the WRF model, the simulations are performed for 10 separate subdomains and later merged to provide one unified atlas (Fig. 1). The WRF modelling covers 30 years from 1989 to 2018. For the satellite data collection, processing and analysis, it is convenient to select an area of interest within latitudes (here 33.5 to 72.2∘ N) and longitudes (here 19.4∘ W to 47∘ E).
3.2 ASCAT and SAR ocean wind processing
The scatterometer ASCAT is on board the meteorological MetOp-A and MetOp-B satellites observing from 2007 and 2012, respectively, to present. MetOp-C was launched in 2018 although its data are not used in the present study. All are operated by the European Organisation for the Exploitation of Meteorological Satellites (EUMETSAT). The Level 3 data obtained through the Copernicus Marine Environmental Monitoring Service are the coastal stress equivalent wind product (WIND_GLO_WIND_L3_NRT_OBSERVATIONS_012 _002). They include wind speed and wind direction at 10 m height above sea level at a spatial resolution of 12.5 km (De Kloe et al., 2017; CMEMS, 2019). Depending on the area of interest, satellite overpass times can range from two to four per day, while the measurements are considered instantaneous (CMEMS-OSI-PUM-012-002, 2019). Near coastlines, quality control omits pixels contaminated by land that cause fundamentally different scattering than ocean waves. Level 1 Wide Swath Mode (WSM) acquisitions from the Envisat ASAR (Advanced SAR) mission, from 2002 to 2012, are collected in their entirety for the area of interest. The scenes used in this study include co-polarized VV and HH scenes (VV is vertical receiving and vertical transmitting, and HH is horizontal receiving and transmitting). Envisat was a research mission of the European Space Agency (ESA).
Level 1 Extra Wide (EW) and Interferometric Wide (IW) mode acquisitions from the Sentinel-1A mission (2014–present) and Sentinel-1B (2016–present) are collected in their entirety for the area of interest. The scenes used in this study include EW and IW mode and VV and HH polarization. Sentinel-1A/B are parts of Copernicus, the European Commission's monitoring programme. Table 1 lists the source data from ASCAT, Envisat and Sentinel-1.
ASCAT, Envisat and Sentinel-1 are polar-orbiting satellites. The number of samples of ocean wind data in any pixel (grid cell) depends upon the data recordings in time and space. For Envisat this was inhomogeneous due to various research priorities in the beginning of the mission. During later years (2008 to 2012), recording was high and consistent in the area of interest. ASCAT-A/B and Sentinel-1A/B are operational monitoring satellites and have frequent coverage in the entire domain since launch. For all satellites, there are more samples available at higher latitudes due to the polar-orbital paths. The SAR wind retrieval is based upon calibrated radar backscatter values (the normalized radar cross section) and application of the GMF CMOD5.N (Hersbach, 2010). CMOD5.N gives the equivalent neutral wind at 10 m height above sea level. This is for radar data in VV polarization. There is no GMF for HH data; therefore a conversion, the so-called polarization ratio linking the VV and HH data together, needs to be applied before wind retrieval. For HH data, the polarization ratio of Mouche et al. (2005) is selected. The a priori wind directions needed to perform wind retrieval are selected at 10 m height from the NCEP/NCAR Climate Forecast System Reanalysis (CFSR) reanalysis data until 2010, and the Global Forecast System (GFS) data are used from 2011 onward. To match the SAR images, an interpolation of wind directions is performed. The SAR Ocean Products System (SAROPS) software from Johns Hopkins University Applied Physics Laboratory and National Ocean and Atmosphere Agency (JHU APL and NOAA) is used for the processing (Monaldo et al., 2014), which occurs operationally at DTU Wind Energy; all wind retrievals are openly available through https://satwinds.windenergy.dtu.dk/ (last access: 24 March 2020). In regions with sea ice, ocean winds cannot be retrieved, and thus these areas are masked out using the National Ice Center's Interactive Multisensor Snow and Ice Mapping System (IMS) with daily data at 4 km resolution (National Ice Center, 2008).
Satellite winds retrieved at 10 m height are averaged into wind resource statistics using the software for SAR-based wind resource assessment (Hasager et al., 2008, 2011; Ahsbahs et al., 2019) and for ASCAT using the methodology presented in Karagali et al. (2018b). Wind turbines offshore have hub heights at around 100 m height. Therefore, an extrapolation of wind speed from 10 to 100 m height is applied. Previous investigations show that applying a long-term stability correction is superior to a neutral logarithmic wind profile in the Baltic Sea (Badger et al., 2016) and in the North Sea (Karagali et al., 2018a). For the NEWA offshore wind atlas, the extrapolation is performed similarly to Karagali et al. (2018a, b) using 10 years of WRF model simulations from Nuño Martinez et al. (2018) for the long-term stability correction.
3.3 Mesoscale modelling
The WRF model (Skamarock et al., 2008) used for the production run of the New European Wind Atlas is a limited-area weather forecast model. The WRF model is a public domain, open-source modelling system, which has previously been used to produce a wind atlas for South Africa (Hahmann et al., 2015b), the North Sea and Baltic Sea (Hahmann et al., 2015b), and Denmark (Peña and Hahmann, 2017) and wind statistics for Europe (Nuño Martines et al., 2018).
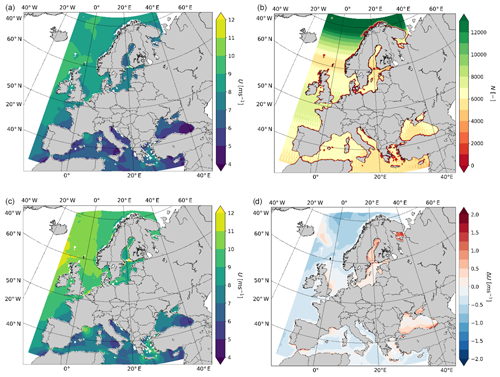
Figure 2For ASCAT the mean wind speed (m s−1) at 10 m height (a), number of samples (b), mean wind speed at 100 m including long-term stability correction for extrapolation (c) and difference in wind speed at 100 m a.m.s.l. height based on long-term stability correction minus neutral wind profile assumption (d) are shown.
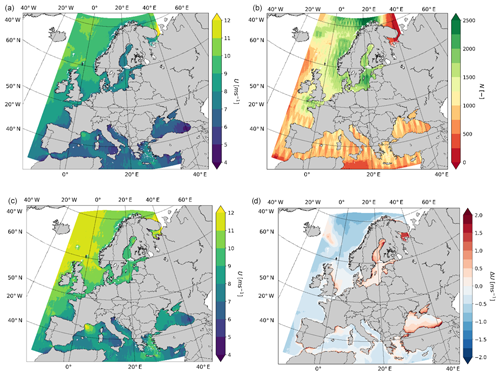
Figure 3For Envisat ASAR and Sentinel-1 combined mean wind speed (m s−1) at 10 m height (a), number of samples (b), mean wind speed at 100 m a.m.s.l. including long-term stability correction for extrapolation (c) and difference on wind speed at 100 m a.m.s.l. height based on long-term stability correction minus neutral wind profile assumption (d) are shown.
The production run for NEWA (see Fig. 1) was computed on the HPC cluster MareNostrum at the Barcelona Supercomputing Center and on HPC Cluster EDDY at the University of Oldenburg. In order to determine the optimal model scheme and forcing, surface input, and land surface model, a series of sensitivity tests were conducted and compared to tall meteorological mast data masts and lidar data in northern Europe and the North Sea. No setting was optimal for all, so a compromise was made, which provided the best verification statistics (see Witha et al., 2019, for more details). In brief, the production run was set up for 10 separate WRF domains, which shared the same outer domain and map projection, and later merged to provide one unified atlas (https://map.neweuropeanwindatlas.eu, last access: 24 March 2020). The WRF model used was a modified version of 3.8.1, set up with the Mellor–Yamada–Nakanishi–Niino (MYNN) planetary boundary layer (Nakanishi and Niino, 2009) and Monin–Obukhov surface layer (Monin and Obukhov, 1954) schemes. Forcing for the simulations was from ERA5 reanalysis (ERA5, 2017) at grid spacing and OSTIA sea surface temperature (Donlon et al., 2012) at 1∕20∘ grid spacing. The CORINE land cover data at 100 m resolution were used to define the land use classes (Copernicus Land Monitoring Service, 2019), except for areas they do not cover, in which case ESA CCI data are used. The NOAH land surface model and icing WSM5 plus ice code and sum of cloud and ice humidity are used. The WRF simulations used three nested domains at 27, 9 and 3 km and 61 vertical layers, with 8 d overlapping runs using spectral nudging with 24 h spin-up (see Hahmann et al., 2020, for details on the technique). There are 20 model levels below 1 km, and the lowest levels are located at 5.6, 21.8, 40.4, 56.6, 72.8, 90.7, 113.2, 140.1, 170.7, 205.3 and 244.5 m above ground level. The years covered and spatial resolution are listed in Table 1.
4.1 Satellite-based offshore wind speed maps
Figure 2 shows the offshore wind speed maps for the European seas based on the entire archive of ASCAT at 10 and 100 m height, the number of samples, and wind speed difference at 100 m using extrapolation with long-term stability correction minus neutral profile extrapolation. Similar results for SAR are shown in Fig. 3.
The same colour scale is used for ASCAT and SAR in Figs. 2 and 3, except for the number of samples due to the difference in sample maxima between ASCAT and SAR. The polar orbits result in more frequent sampling at higher latitudes. The harlequin pattern in sampling is due to the ascending and descending orbits for both ASCAT and SAR, but it is most noticeable for SAR due to the swaths and orbital settings.
For the European seas, the number of samples in the grid cells for ASCAT is greater than 4000 and in most places greater than 6000, up to more than 12 000 at high latitudes (see Fig. 2). The number of samples for SAR is between 500 and 2500 (see Fig. 3). For the WRF model, the number is constant at all locations covered with 525 912 samples (every 30 min from 1989 to 2018).
The mean wind speed consistently shows higher values for the 100 m height than 10 m height in both ASCAT and SAR. The wind speed difference maps at 100 m based on long-term stability correction minus neutral wind profile assumption show very similar spatial patterns between ASCAT and SAR, as expected. The variation is up to ±2 m s−1 with high positive values in the Baltic Sea and Black Sea and with high negative values in the Norwegian Sea. Positive values occur for stable conditions. The continental climate dominating the flow in the Baltic Sea and the Black Sea causes the variations. Negative values occur for unstable conditions prevalent in the Norwegian Sea and in the Mediterranean Sea. According to Kara et al. (2009) the overall stability in the Mediterranean Sea is slightly unstable. In the North Sea, a gradient is observed with slightly negative values along the continental coast and positive values along the UK coast. This corresponds well with the average stability over the North Sea (Peña and Hahmann, 2012), where unstable conditions prevail along the continental coast and stable conditions prevail near the UK. The Mediterranean Sea has mixed wind speed difference variations dominated by moderately negative values in the central part and positive values in the Greek archipelago and the French Riviera.
4.2 WRF offshore wind speed map
The long-term offshore wind speed map at 100 m height in the European seas based on the WRF production run is shown in Fig. 4, using the same colour scale as for ASCAT and SAR in Figs. 2 and 3.
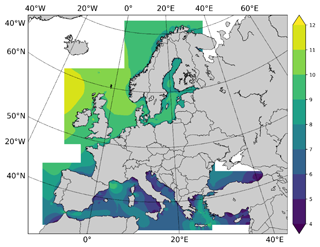
Figure 4WRF New European Wind Atlas production run mean wind speed (m s−1) at 100 m height for 1989 to 2018 with 3 km spatial grid spacing.
ASCAT and WRF have many similarities in the spatial wind speed patterns and the range of mean wind speeds at 100 m height. The SAR mean wind speed at 100 m height appears to be higher than ASCAT and WRF. Furthermore, SAR shows more fine-scale spatial variations than both ASCAT and WRF.
4.3 Comparison of offshore mean wind speed maps at 100 m height
Comparisons of the ASCAT, SAR and WRF mean wind speed maps at 100 m height performed using the long-term stability-corrected versions from ASCAT and SAR are shown in Fig. 5. ASCAT versus WRF (panel a) shows lower differences in mean wind speed than SAR versus WRF (panel b). ASCAT minus SAR (panels c) shows a consistent negative bias of winds from SAR, except for some artefact in ASCAT near the Dutch coastline, attributed to higher backscatter from the surface due to the congestion of large ships to and from Rotterdam (notice distinct yellow area in Fig. 2 that without ships would be green; in other words, the high backscatter translates into falsely high wind speed).
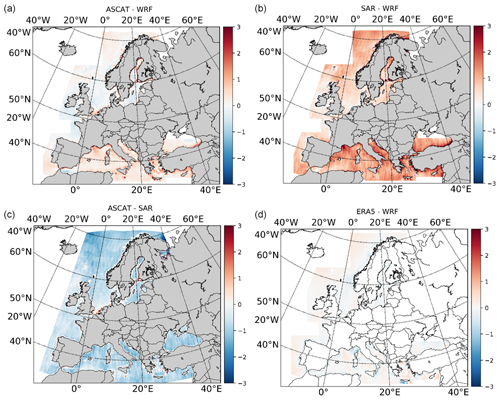
Figure 5Comparison of mean wind speed (m s−1) at 100 m height: ASCAT minus WRF (a), SAR minus WRF (b), ASCAT minus SAR (c), and ERA5 minus WRF (d).
The ERA5 mean wind speed at 100 m height is included for comparison with WRF in Fig. 5 (panel d). Only grid cells with more than 95 % sea according to the ERA5 land mask are included. The mean wind speed difference map of ERA5 minus WRF shows some variations. There are both large positive and large negative values in the Mediterranean Sea. The differences are smaller in the northern European seas. Along several coastlines such as the Norwegian Sea, the Atlantic Sea and the Mediterranean Sea large differences are found between the two datasets. These are attributed to the lack of ability in ERA5 to properly resolve the coastal atmospheric flow phenomena such as land–sea breeze and flow intensification. See Beal et al. (2015) for further details on coastal atmospheric flow phenomena observed in SAR. ERA5 has a coarse spatial resolution omitting islands such as Bornholm and the Isle of Man. Coastal atmospheric flow phenomena near Crete are investigated in Sect. 5.
Please note the number of samples and the grid spacing are different. WRF has 30 min values from 30 years (525 912 samples) with 3 km resolution. ERA5 has hourly values from 30 years (262 956 samples) with about 27 km resolution.
With regards to spatial resolution, it is obvious that SAR resolves finer spatial detail than other products. From spectral analysis of SAR vs. scatterometer winds, it was found that SAR resolves around 4 km features, and the scatterometer resolves around 25 km features (Karagali et al., 2013b). The latter is comparable in scale to what the WRF model at 3 km grid spacing resolves, i.e. around 20 km (Skamarock, 2004). ERA5 resolves scales around 150 km. The use of structure functions and spectra from other numerical weather models has shown the effective model resolution to be about 6 times lower than the size of the grid cell (Frehlich and Sharman, 2008).
The wind speed difference error distributions between wind speed at 100 m height for ASCAT minus WRF, SAR minus WRF, ASCAT minus SAR and ERA5 minus WRF are shown in Fig. 6. ASCAT minus WRF has a slightly positive bias and narrow range. ASCAT minus SAR has a negative bias and moderate range. SAR minus WRF has a positive bias and broad range. The narrow range is expected for products that resolve similar length scales while broader ranges are expected for products that resolve different length scales. The results shown in Fig. 6 support this very well, as ASCAT and WRF resolve similar scales and SAR and WRF resolve very different scales. SAR generally shows higher wind speeds than ASCAT and WRF. The long positive tails of ASCAT minus WRF and SAR minus WRF are explained by coastal winds in the Mediterranean Sea. ERA5 minus WRF has a slightly positive bias and narrow range. All probability density distributions except ASCAT minus SAR show bimodal distributions explained by the differences in area of the northern seas and Mediterranean Sea; see Fig. 5.
5.1 Motivation and aim
The motivation for presenting a case study is twofold. Firstly, by looking into a small area of interest, spatial details in winds observed can be analysed and used as an example for characterizing the SAR and WRF data sources. More specifically, the goal of this case study is to study the interaction between large-scale flow and orography. The second motivation is to stimulate interest for further investigation using the different data sources at other locations in Europe and outline the methodology.
5.2 Selection of data
The sea surrounding western Crete is chosen due to interesting mesoscale flow patterns. Figures 7, 8, 10 and 11 show spatial wind patterns in the area. The area of investigation is located between 23.4 to 24.8∘ E and 34.6 to 36.0∘ N. The SAR scenes available from the database satwinds.dtu.dk at DTU Wind Energy are selected. To have spatial consistency between SAR and WRF, only SAR scenes that fully cover the area (consecutive scenes are merged) are selected.
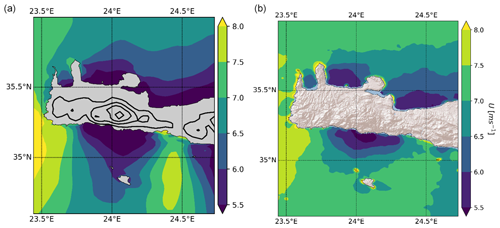
Figure 7Mean wind speed (m s−1; 2002–2018) at 10 m a.m.s.l. for (a) WRF and (b) SAR. All 549 coinciding scenes are used in both datasets.
There are 549 SAR scenes between 2002 and 2018 in total from Envisat and Sentinel-1. Only coinciding WRF data are selected. The SAR and WRF mean wind speeds at 10 m height are displayed in Fig. 7. Some wind features are similar in SAR and WRF, e.g. lower wind speeds south and north of Crete close to the shore. A distinct jet south of the island is much more pronounced in the WRF data than in SAR. Figure 7a shows the height contour lines from the elevation map used in the WRF model. To characterize the complex landscape in Crete, a more detailed elevation map is embedded in the SAR map in Fig. 7b. Small-scale elevation features not represented in the WRF model may explain wind speed differences between WRF and SAR. The jet could be weaker or absent since the fine-scale elevation features, neglected in WRF, block the atmospheric flow. For instance, what is a simple valley without any obstacles in WRF orography, in reality (and therefore in SAR data), could be blocked by a small mountain range. Koletsis et al. (2009) demonstrated the sensitivity of gap wind speeds in a mesoscale model to the changes in the elevation.
The variability in coastal flow around Crete depends highly on the wind direction. Two of the prevalent wind directions are of particular interest – namely northerly and westerly winds. Northerly winds over Crete are associated with the so-called Etesian wind often present in the region during summer and known to produce gap flows between the two large mountains in the east side of the island (Lefka Ori) and in the centre (Idi) (Koletsis et al., 2010). Westerly winds in this region have been associated with trapped lee waves (Miglietta et al., 2013).
As already stated, the goal of this case study is to demonstrate the interaction between large-scale flow and orography. It is necessary to choose situations where the upwind flow conditions are simple. This is to avoid wind conditions such as low wind speed with poorly defined direction, anti-cyclonic situations and local flows, e.g. sea breezes that could create a complicated wind field that would be difficult to interpret. Therefore, the wind speeds should be sufficiently high, and the wind direction should be representative for the entire domain.
To determine a representative flow direction, ERA5 wind speeds and directions extracted at the locations indicated in Fig. 8 are used. Figure 8 also shows the mean wind speed from the 549 coinciding ERA5 model simulations. ERA5 resolves the mean wind speed with much less spatial detail than WRF (compare Figs. 8 and 7b). The average wind speed at three points (A, C, E) is required to be above 3 m s−1. For the wind direction, the centre location upstream (B for northerly, D for westerly) should be within 30∘ of that direction. We further require that the neighbouring upstream points do not differ by more than 20∘ from the centre. Figure 9 illustrates the flow chart used for classification.
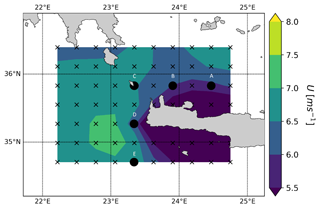
Figure 8Mean wind speed (m s−1) from ERA5 for 549 cases collocated with SAR scenes around Crete with points used for extracting wind speed and direction for classification. The five locations A to E are mentioned in the text.
The mean wind speed maps based on SAR and WRF for 59 cases of northerly and 57 cases of westerly flows are presented in Fig. 10. For northerly flow, notable differences exist between the SAR and WRF maps. The WRF winds show strong shadowing at 24∘ E and a pronounced jet-like structure at 24.5∘ E. These features are present in SAR as well, but much less pronounced. For westerly flow, good agreement between SAR and WRF is noted. Areas of increased wind speed to the south and the north are visible in both maps. A stagnation point area of low wind speed is located on the western side of Crete in both SAR and WRF maps. Stronger winds may increase surface water mixing, causing colder sea surface temperature (not shown).
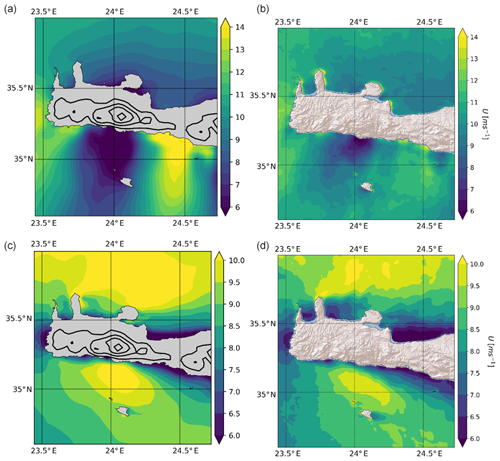
Figure 10Mean wind speeds (m s−1) from WRF (a, c) and SAR (b, d) at 10 m. (a, b) Northerly flow based on 59 collocated cases from 2002 to 2018. (c, d) Westerly flow based on 57 collocated cases from 2002 to 2018.
To clarify further similarities and discrepancies between SAR and WRF, two individual examples are chosen and compared to WRF (see Fig. 11): one case of northerly flow from 5 July 2017 at 04:24 UTC and one case of westerly flow from 6 May 2017 at 04:24 UTC.
The northerly flow SAR case (Fig. 11b) contains significant atmospheric waves. Although some evidence for atmospheric wave activity is also identified in the WRF data, namely periodic changes of flow over time (not pictured), no wave structure similar in wavelength to the one visible in SAR is identified in WRF. This could be because topography of the appropriate resolution is not present in the WRF model and thus cannot be resolved in the mode solution. In addition, the WRF model grid is too coarse to resolve the scale of the observed waves. The wind speed maxima in SAR and WRF compare well, as do the minima for the northerly flow case (Fig. 11a, b). The much weaker jet identified in the average of northerly flows in SAR vs. WRF (in Fig. 11a, b) can, in part, be explained by the gravity wave amplitude maxima not occurring at the same location. The averaging of out-of-phase waves can lead to destructive interference and result in the average sum of lower values in SAR. In contrast, WRF appears to simulate only the maxima of the unresolved waves.
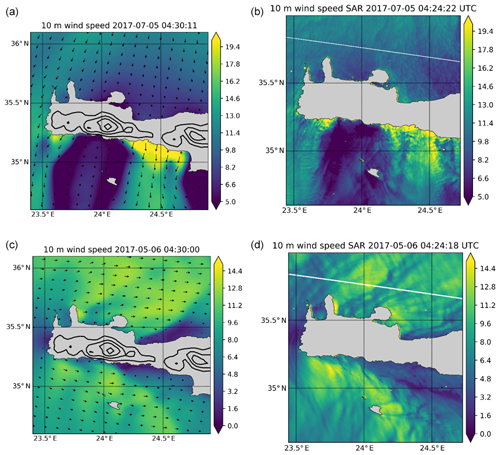
Figure 11Wind speed (m s−1) from WRF data (a, c) and SAR data (b, d). (a, b) Northerly flow on 5 July 2017, 04:24 UTC. (c, d) Westerly flow on 6 May 2017, 04:24 UTC (the white lines in SAR panels are consecutive scene borders).
The westerly flow case for SAR and WRF (Fig. 11c, d) also shows atmospheric wave activity. However, in this case the waves seem to have a longer wavelength and therefore could – although imperfectly – be reproduced in WRF; WRF still shows significantly longer wavelengths than SAR.
In summary, the orography in WRF is somewhat simplified and therefore significant features playing critical role in complicated flows are omitted. Another direction of investigation is to assess whether the atmospheric stability in the flow before it arrives at the obstacle has the correct representation in WRF. Meteorological observations are unfortunately not available for comparison.
The atmospheric stability from WRF has been investigated. We use the stability classes derived from the Obukhov length following Gryning et al. (2007) and map the stability at the time of the two cases shown in Fig. 11. The results are presented in Fig. 12. For the case with winds from the north, the inflow is very unstable at the northern shore of Crete, but in the high-speed jet south of Crete the flow is neutral. In the low-speed zone south of Crete there is a complicated pattern of atmospheric stability – with a large zone of very unstable flow. For the case with winds from the west, the inflow (west of Crete) is again very unstable, and the wind speed maxima are again associated with neutral stability conditions, but this time in the low-wind-speed zones near the coastline north and south of Crete very stable stratification is found.
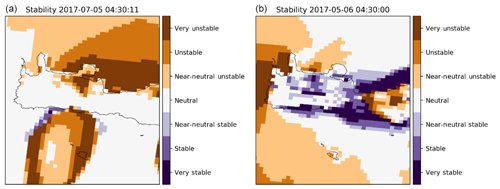
Figure 12Stability class following Gryning et al. (2007) in WRF for the cases with winds from the north (a) and from the west (b).
The results in Fig. 12 show a snapshot of stability in time; however, the focus on this paper is the climatological properties. Therefore, the next question arises – are these stability patterns typical? To answer this question, we analyse the stability patterns in the WRF dataset that coincides with SAR scenes (Fig. 10). The stability distribution for the 59 cases of northern winds (not shown) is characterized by a high probability of very unstable flow both in the inflow (north of Crete) and in the low-speed zone. A small increase in the frequency of neutral winds in the jet zone is also noted. This result allows us to argue that the conclusions from Fig. 12 can be generalized. The average stability for the 57 cases of westerly winds (not shown) has very unstable inflow to the west of Crete as in Fig. 12. The high-wind areas are associated with neutral or near-neutral stratification while the low-wind zones along the coastlines to the north and south have very unstable stratification. This leads us to conclude that the pattern in stability for westerly flow is similar between the single case (Fig. 11) and average (Fig. 10) except very close to the coastline for low wind speeds.
In wind resource mapping it is traditional to use hourly wind speed observations from one year (8760 samples) or ideally with higher temporal frequency and during more years from (tall) meteorological mast wind observations or wind profiling lidar. Offshore tall masts are few, and thus data are sparse. This stimulates research into atmospheric modelling and alternative observations, including satellite observations. At the onset of satellite data analysis for offshore wind resource mapping, few satellite scenes were available. Pioneering work (Barthelmie and Pryor, 2003; Pryor et al., 2004) focused on the number of samples relevant for assessing the mean wind speed, the Weibull scale and shape parameters, and the energy density.
Furthermore, the non-random sampling in time of sun-synchronous satellites (for ASCAT A/B these are local times around 09:30 and 21:30, for Envisat around 10:30 and 22:30, and for Sentinel-1 A/B around 06:00 and 18:00) may potentially bias the wind resource statistics, in the case of diurnal wind speed variations. The passive microwave wind observations with several more local observation times did not show much variation in diurnal cycle wind speeds in the central North Sea (Hasager et al., 2016), but near coastlines land–sea breezes prevail, causing systematic diurnal wind speed variations.
Methods to deal with few satellite samples include the hybrid method (Badger et al., 2010) and the gap-filling method during periods with a lack of data due to sea ice (Doubrawa et al., 2015). The adjustment for few samples and for uneven diurnal or seasonal sampling only makes sense to perform for local sites or regions (Ahsbahs et al., 2019) rather than for all the European seas. In the case meteorological observations are accessible, these can be useful for comparison and adjustment.
At the European scale, the SAR wind speed archive may be improved for future analysis, using the novel inter-calibration method proposed by Badger et al. (2019) and applied for SAR-based wind resource assessment along the US East Coast (Ahsbahs et al., 2019). The tendency in this inter-calibration is to decrease the SAR wind speeds. This obviously would make the comparison to both ASCAT and WRF agree better in the European seas. Further validation of the offshore WRF winds with masts and lidar observations at around 100 m a.m.s.l. in the North Sea shows smaller biases than those identified in Figs. 5 and 6 (Gonzalez-Rouco et al., 2019), which substantiates this hypothesis. It could furthermore be interesting to consider SAR and ASCAT inter-calibration such that coherent satellite datasets could be the foundation for further inter-comparison to WRF model results, for example. ASCAT and WRF test-run comparisons (Karagali et al., 2018a, b) as well as inter-comparison of WRF test runs and meteorological observations have proven valuable.
For planning of wind farms, statistics on wind speed and direction are crucial to agreeing on a central estimate of the long-term annual net energy production and for optimal design of turbine layout within the wind farm areas. ASCAT provides observations of wind speed and wind direction; thus wind roses based on ASCAT are fully independent observations (e.g. Karagali et al., 2018b). SAR only provides observations of wind speed and of direction based upon the interpolated wind directions from global models (e.g. Badger et al., 2010; Ahsbahs et al., 2019). Thus, wind roses from SAR are mixed from satellite data and modelling. WRF provides modelled wind speeds and wind directions. ERA5 is a valuable dataset, even though ERA5 resolves less spatial detail in offshore winds than the WRF production run, but ERA5 wind directions could be an alternative to CFSR and GFS wind directions as input for SAR wind retrieval. ERA5 input could potentially result in a more homogenous SAR-based wind dataset for the European seas.
The opportunities for further investigations and analysis based on the New European Wind Atlas offshore are numerous. They include long-term wind speed and wind direction trends, future wind climate, comparison to various new wind data sources, high-fidelity modelling of winds, extreme winds, seasonal dependencies in winds, wind farm cluster effects between large offshore wind farms, wind energy production variability, new perspectives on marine boundary layer flow physics, processes and meteorological parameters, and air–sea interactions, among other topics. It is the beginning of a new era in offshore wind energy research and applications.
The hitherto most comprehensive wind atlas for the European seas was published based on Envisat ASAR and Sentinel-1 A/B SAR satellite scenes, ASCAT A/B scatterometer satellite scenes, and WRF mesoscale model production run results.
The WRF model covers 1989–2018 (30 years) with spatial grid spacing of 3 km and results every 30 min (in total 525 912 samples). The SAR wind archive covers, from 2002 to 2018 with spatial resolution of 2 km, in total around 500 to 2500 samples during the years. The ASCAT wind archive covers, from 2007 to 2018 with spatial resolution 12.5 km, in total around 5000 to 12 000 samples during the years.
Comparison results between SAR and WRF for the Crete case study reveal fine-scale flow structures in SAR not fully captured in WRF. However, overall ASCAT and WRF produce similar results of the mean wind speed across the European seas at 100 m height while SAR is positively biased. It is expected this bias may be diminished or removed using the inter-calibration method for SAR.
SAR wind maps from DTU Wind Energy can be found at https://satwinds.windenergy.dtu.dk (DTU Wind Energy, 2020a). ASCAT wind maps from Copernicus Marine Environmental Monitoring Service are the coastal stress equivalent wind product (WIND_GLO_WIND_L3_NRT_OBSERVATIONS_012_002). NEWA WRF model results are available at https://map.neweuropeanwindatlas.eu/ (DTU Wind Energy, 2020b). ERA5 ECMWF can be found at https://www.ecmwf.int/en/forecasts/datasets/reanalysis-datasets/era5 (ECMWF, 2020).
CBH wrote the article and coordinated offshore wind atlas. ANH coordinated the WRF modelling, and TS assisted in WRF modelling and comparison. TA and TS analysed the Crete case study. TS performed the stability analysis. IK analysed ASCAT. TS prepared the graphics. MB analysed SAR. JM coordinated NEWA experiments and projects. All authors contributed to discussion of and writing of the article.
The authors declare that they have no conflict of interest.
The authors acknowledge the funding provided to the New European Wind Atlas project, in part funded by the European Commission's ERANET+, the Danish Energy Agency, and grants for the supercomputers PRACE and EDDY. ASCAT data are from the EUMETSAT, KNMI and CMEMS service. Envisat ASAR data are from ESA. Sentinel-1 data are from EC Copernicus. The SAR processing is based on the SAROPS software from JHU APL and NOAA. Tija Sile acknowledges the financial support of the project “Mathematical modelling of weather processes – development of methodology and applications for Latvia (1.1.1.2/VIAA/2/18/261)”. The authors gratefully acknowledge the good collaboration with the WP3 partners of the NEWA project. Thank you to Rémi Gandoin and the two anonymous reviewers for their helpful assessment of the manuscript.
The European Commission (EC) partly funded NEWA (NEWA- New European Wind Atlas) through FP7 (topic FP7ENERGY.2013.10.1.2) The authors of this paper acknowledge the support the Danish Energy Authority (EUDP 14-II, 64014-0590, Denmark). Tija Sile acknowledges the financial support of the project Mathematical modelling of weather processes – development of methodology and applications for Latvia (1.1.1.2/VIAA/2/18/261).
This paper was edited by Rebecca Barthelmie and reviewed by two anonymous referees.
4C Offshore 2019: available at: https://www.4coffshore.com/, last access: 17 June 2019.
Ahsbahs, T., Badger, M., Karagali, I., and Larsén, X. G.: Validation of Sentinel-1A SAR Coastal Wind Speeds Against Scanning LiDAR, Remote Sens., 9, 552, https://doi.org/10.3390/rs9060552, 2017.
Ahsbahs, T., Badger, M., Volker, P., Hansen, K. S., and Hasager, C. B.: Applications of satellite winds for the offshore wind farm site Anholt, Wind Energ. Sci., 3, 573–588, https://doi.org/10.5194/wes-3-573-2018, 2018.
Ahsbahs, T., Maclaurin, G., Draxl, C., Jackson, C., Monaldo, F., and Badger, M.: US East Coast synthetic aperture radar wind atlas for offshore wind energy, Wind Energ. Sci. Discuss., https://doi.org/10.5194/wes-2019-16, in review, 2019.
Badger, M., Badger, J., Nielsen, M., Hasager, C. B., and Peña, A.: Wind class sampling of satellite SAR imagery for offshore wind resource mapping, J. Appl. Meteorol. Clim., 49, 2474–2491, https://doi.org/10.1175/2010JAMC2523.1, 2010.
Badger, M., Peña, A., Hahmann, A. N., Mouche, A., and Hasager, C. B.: Extrapolating satellite winds to turbine operating heights, J. Appl. Meteorol. Clim., 55, 975–991, https://doi.org/10.1175/JAMC-D-15-0197.1, 2016.
Badger, M., Ahsbahs, T., Maule, P., and Karagali, I.: Inter-calibration of SAR data series for offshore wind resource assessment, Remote Sens. Environ., 232, 111316, https://doi.org/10.1016/j.rse.2019.111316, 2019.
Barthelmie, R. J. and Pryor, S. C.: Can satellite sampling of offshore wind speeds realistically represent wind speed distributions, J. Appl. Meteorol., 42, 83–94, 2003.
Beal, R. C., Young, G. S., Monaldo, F., Thompson, D. R., Winstead, N. S., and Schott, C. A.: High Resolution Wind Monitoring with Wide Swath SAR: A User's Guide, U.S. Department of Commerce, Washington, DC, USA, 1–155, 2015.
Berge, E., Byrkjedal, O., Ydersbond, Y., and Kindler, D.: Modelling of offshore wind resources, Comparison of a meso-scale model and measurements from FINO1 and North Sea oil rigs, Scientific Proceedings EWEC'09, Marseille, France, 16–19 March 2009.
Bischoff, O., Yu, W., Gottschall, J., and Cheng, P. W.: Validating a simulation environment for floating lidar systems. The Science of Making Torque from Wind (TORQUE 2018) IOP Publishing, J. Phys. Conf. Ser., 1037, 052036, https://doi.org/10.1088/1742-6596/1037/5/052036, 2018.
Casale, C., Lembo, E., Serri, L., and Viani, S.: Italy's Wind Atlas:Offshore Resource Assessment Through On-The-Spot Measurements, Wind Engineering, 34, 17–28, 2010.
Christiansen, M. B., Koch, W., Horstmann, J., Hasager, C. B., and Nielsen, M.: Wind resource assessment from C-band SAR, Remote Sens. Environ., 105, 68–81, 2016.
CMEMS: Copernicus Marine Environmental Monitoring Service, available at: http://marine.copernicus.eu/, last access: 17 June 2019.
CMEMS-OSI-PUM-012-002: Product User Manual for Wind-Global Ocean L3 Wind WIND_GLO_WIND_L3_NRT_OBSERVATIONS_012_002, Issue 2.9, EU Copernicus Marine Service, 2016.
Copernicus Land Monitoring Service: CORINE Land Cover, available at: https://land.copernicus.eu/pan-european/corine-land-cover, last access: 26 October 2019.
De Kloe, J., Stoffelen, A., and Verhoef, A.: Improved Use of Scatterometer Measurements by Using Stress-Equivalent Reference Winds, IEEE J. Sel. Top. Appl., 10, 2340–2347, 2017.
Donlon, C. J., Martin, M., Stark, J. D., Roberts-Jones, J., Fiedler, E., and Wimmer, W.: The operational sea surface temperature and sea ice analysis (OSTIA), Remote Sens. Environ., 116, 140–158, https://doi.org/10.1016/j.rse.2010.10.017, 2012.
Doubrawa, P., Barthelmie, R. J., Pryor, S. C., Hasager, C. B., Badger, M., and Karagali, I.: Satellite winds as a tool for offshore wind resource assessment: The Great Lakes Wind Atlas, Remote Sens. Environ., 168, 349–359, https://doi.org/10.1016/j.rse.2015.07.008, 2015.
DTU Wind Energy: SAR wind maps, available at: https://satwinds.windenergy.dtu.dk, last access: 24 March 2020a.
DTU Wind Energy: NEWA WRF model result, available at: https://map.neweuropeanwindatlas.eu/TS2, last access: 24 March 2020b.
ECMWF: ERA5, available at: https://www.ecmwf.int/en/forecasts/datasets/reanalysis-datasets/era5, last access: 24 March 2020.
EEA: Europe's onshore and offshore wind energy potential. An assessment of environmental and economic constrains, EEA Technical Report No 6/2009, 90 pp., 2009.
Emeis, S.: Wind Energy Meteorology: Atmospheric Physics for Wind Power Generation (Green Energy and Technology), Springer, 198 pp., 2012.
ERA5: Copernicus Climate Change Service (C3S): ERA5: Fifth generation of ECMWF atmospheric reanalyses of the global climate. Copernicus Climate Change Service Climate Data Store (CDS), available at: https://cds.climate.copernicus.eu/cdsapp#!/home (last access: 17 June 2019), 2017.
Farrugia, R. N. and Sant, T.: A wind resource assessment at Ahrax Point: A node for central Mediterranean offshore wind resource evaluation, Wind Engineering, 40, 438–446, 2016.
Floors, R., Peña, A., Lea, G., Vasiljević, N., Simon, E., and Courtney, M.: The RUNE Experiment – A Database of Remote-Sensing Observations of Near-Shore Winds, Remote Sens., 8, 884, https://doi.org/10.3390/rs8110884, 2016.
Floors, R. R., Hahmann, A. N., and Peña, A.: Evaluating Mesoscale Simulations of the Coastal Flow Using Lidar Measurements, J. Geophys. Res.-Atmos., 123, 2718–2736, https://doi.org/10.1002/2017JD027504, 2018.
Frehlich, R. and Sharman, R.: The use of structure functions and spectra from numerical model output to determine effective model resolution, Mon. Weather Rev., 136, 1537–1553, 2008.
Furevik, B. R., Sempreviva, A. M., Cavaleri, L., Lefèvre, J.-M., and Transerici, C.: Eight years of wind measurements from scatterometer for wind resource mapping in the Mediterranean Sea, Wind Energy, 14, 355–372, https://doi.org/10.1002/we.425, 2011.
Gonzalez-Rouco, F., García Bustamante, E., Hahmann, A. N., Karagali, I., Navarro, J., Olsen, B. T., Sile, T., and Witha, B.: Report on uncertainty quantification (Deliverable D4.4) (Version Final 19.08.2019), Zenodo, https://doi.org/10.5281/zenodo.3382572, 2019.
Gottschall, J., Catalano, E., Dörenkämper, M., and Witha, B.: The NEWA Ferry Lidar Experiment: Measuring Mesoscale Winds in the Southern Baltic Sea, Remote Sens., 10, 1620, https://doi.org/10.3390/rs10101620, 2018.
Gryning, S.-E., Batchvarova, E., Brümmer, B., Jørgensen, H. E., and Larsen, S. E.: On the extension of the wind profile over homogeneous terrain beyond the surface boundary layer, Bound.-Lay. Meteorol., 124, 251–268, https://doi.org/10.1007/s10546-007-9166-9, 2007.
Hahmann, A. N., Rostkier-Edelstein, D., Warner, T. T., Vandenberghe, F., Liu, Y., Babarsky, R., and Swerdlin, S. P.: A reanalysis system for the generation of mesoscale climatographies, J. Appl. Meteorol. Clim., 49, 954–972, 2010.
Hahmann, A. N., Vincent, C. L., Peña, A., Lange, J., and Hasager, C. B.: Wind climate estimation using WRF model output: Method and model sensitivities over the sea, Int. J. Climatol., 35, 3422–3439, https://doi.org/10.1002/joc.4217, 2015a.
Hahmann, A. N., Lennard, C., Badger, J., Vincent, C. L., Kelly, M. C., Volker, P. J. H., and Refslund, J.: Mesoscale modeling for the Wind Atlas of South Africa (WASA) project, DTU Wind Energy, DTU Wind Energy E, No. 0050, 2015b.
Hahmann, A. N., Sile, T., Witha, B., Davis, N. N., Dörenkämper, M., Ezber, Y., García-Bustamante, E., González Rouco, J. F., Navarro, J., Olsen, B. T., and Söderberg, S.: The Making of the New European Wind Atlas, Part 1: Model Sensitivity, Geosci. Model Dev. Discuss., https://doi.org/10.5194/gmd-2019-349, in review, 2020.
Hasager, C. B., Nielsen, M., Astrup, P., Barthelmie, R. J., Dellwik, E., Jensen, N. O., Jørgensen, B. H., Pryor, S. C., Rathmann, O., ad Furevik, B. R.: Offshore wind resource estimation from satellite SAR wind field maps, Wind Energy, 8, 403–419, 2005.
Hasager, C. B., Peña, A., Christiansen, M. B., Astrup, P-, Nielsen, M., Monaldo, F., Thompson, D., and Nielsen, P.: Remote sensing observation used in offshore wind energy, IEEE J. Sel. Top. Appl., 1, 67–79, 2008.
Hasager, C. B., Badger, M., Peña, A., and Larsén, X. G.: SAR–based wind resource statistics in the Baltic Sea, Remote Sens., 3, 117–144, https://doi.org/10.3390/rs3010117, 2011.
Hasager, C. B., Stein, D., Courtney, M., Peña, A., Mikkelsen, T., Stickland, M., and Oldroyd, A.: Hub height ocean winds over the North Sea observed by the NORSEWInD lidar array: Measuring techniques, quality control and data management, Remote Sens., 5, 4280–4303, https://doi.org/10.3390/rs5094280, 2013.
Hasager, C. B., Badger, M., Nawri, N., Furevik, B. R., Petersen, G. N., Björnsson, H., and Clausen, N.-E.: Mapping offshore winds around Iceland using satellite Synthetic Aperture Radar and mesoscale model simulations, IEEE J. Sel. Top. Appl., 8, https://doi.org/10.1109/JSTARS.2015.2443981, 2015a.
Hasager, C. B., Mouche, A., Badger, M., Bingöl, F., Karagali, I., Driesenaar, T., Stoffelen, A., Peña, A., and Longépé, N.: Offshore wind climatology based on synergetic use of Envisat ASAR, ASCAT and QuikSCAT, Remote Sens. Environ., 156, 247–263, https://doi.org/10.1016/j.rse.2014.09.030, 2015b.
Hasager, C. B., Astrup, P., Zhu, R., Chang, R., Badger, M., and Hahmann, A. N.: Quarter-Century Offshore Winds from SSM/I and WRF in the North Sea and South China Sea, Remote Sens., 8, 769, https://doi.org/10.3390/rs8090769, 2016.
Hersbach, H.: Comparison of C-Band Scatterometer CMOD5.N Equivalent Neutral Winds with ECMWF, J. Atmos. Ocean. Tech., 27, 721–736, 2010.
Jimenez, B., Durante, F., Lange, B., Kreutzer, T., and Tambke, J.: Offshore Wind Resource Assessment with WAsP and MM5: Comparative Study for the German Bight, Wind Energy, 10, 121–134, 2006.
Kara, A. B., Wallcraft, A. J., and Bourassa, M. A.: Optimizing surface winds using QuikSCAT measurements in the Mediterranean Sea during 2000–2006, J. Marine Syst., 8, S119–S131, https://doi.org/10.1016/j.jmarsys.2009.01.020, 2009.
Karagali, I., Badger, M., Hahmann, A. N., Peña, A., Hasager, C. B., and Sempreviva, A. M.: Spatial and temporal variability in winds in the Northern European Seas, Renew. Energ., 57, 200–210, https://doi.org/10.1016/j.renene.2013.01.017, 2013a.
Karagali, I., Larsén, X. G., Badger, M., Peña, A., and Hasager, C. B.: Spectral Properties of ENVISAT ASAR and QuikSCAT Surface Winds in the North Sea, Remote Sens., 5, 6096–6115, 2013b.
Karagali, I., Peña, A., Badger, M., and Hasager, C. B.: Wind characteristics in the North and Baltic Seas from the QuikSCAT satellite, Wind Energy, 17, 123–140, https://doi.org/10.1002/we.1565, 2014.
Karagali, I., Badger, M., and Hasager, C. B.: ASCAT winds used for offshore wind energy applications, Proceedings for the 2018 EUMETSAT Meteorological Satellite Conference, 17–21 September 2018, Tallinn, Estonia, 2018a.
Karagali, I., Hahmann, A. N, Badger, M., Hasager, C. B., and Mann, J.: New European Wind Atlas offshore, Proceedings for The Science of Making Torque from Wind (TORQUE 2018), IOP Conference Series: Journal of Physics: Conference Series 1037, 5, 2018b.
KNMI: The Royal Dutch Meteorological Institute, Dutch Offshore Wind Atlas (DOWA), available at: https://www.dutchoffshorewindatlas.nl/about-the-atlas, last access: 26 October 2019.
Koletsis, I., Lagouvardos, K., Kotroni, V., and Bartzokas, A.: The interaction of northern wind flow with the complex topography of Crete Island – Part 1: Observational study, Nat. Hazards Earth Syst. Sci., 9, 1845–1855, https://doi.org/10.5194/nhess-9-1845-2009, 2009.
Landberg, L.: Meteorology for wind energy: An introduction, Wiley, 224 pp., 2016.
Lavagnini A., Sempreviva, A. M., Transerici, C., Accadia, C., Casaioli, M., Mariani, S., and Speranza, A.: Offshore Wind Climatology over the Mediterranean Basin, Wind Energy, 9, 251–266, 2006.
Mann, J., Angelou, N., Arnqvist, J., Callies, D., Cantero, E., Arroyo, R. C., Courtney, M., Cuxart, J., Dellwik, E., Gottschall, J., Ivanell, S., Kuhn, P., Lea, G., Matos, C. J. S. D., Palma, J. M. L. M., Pauscher, L., Peña, A., Rodrigo, J. S., Söderberg, S., Vasiljevic, N., and Veiga Rodrigues, C.: Complex terrain experiments in the New European Wind Atlas, Philos. T. Roy. Soc. A, 375, https://doi.org/10.1098/rsta.2016.0101, 2017.
Miglietta, M. M., Zecchetto, S., and De Biasio, F.: A comparison of WRF model simulations with SAR wind data in two case studies of orographic lee waves over the Eastern Mediterranean Sea, Atmos. Res., 120, 127–146, 2013.
Monaldo, F. M., Li, X., Pichel, W. G., and Jackson, C. R.: Ocean wind speed climatology from spaceborne SAR imagery, B. Am. Meteorol. Soc., 95, 565–569, https://doi. org/10.1175/BAMS-D-12-00165.1, 2014.
Monin, A. S. and Obukhov, A. M.: Basic laws of turbulent mixing in the surface layer of the atmosphere, Contrib. Geophys. Inst. Acad. Sci. USSR, 151, 163–187, 1954.
Mouche, A., Hauser, D., J., Daloze, J., and Gueri, C.: Dual-polarization measurements at C-band over the ocean: Results from airborne radar observations and comparison with ENVISAT ASAR data, IEEE T. Geosci., 43, 753–769, 2005.
Nakanishi, M. and Niino, H.: Development of an improved turbulence closure model for the atmospheric boundary layer, J. Meteorol. Soc. Jpn., 87, 895–912, https://doi.org/10.2151/jmsj.87.895, 2009.
National Ice Center 2008: IMS daily Northern Hemisphere snow and ice analysis at 4 km and 24 km resolution. Boulder, Colorado USA, National Snow and Ice Data Center, https://doi.org/10.7265/N52R3PMC, 2008.
Nuño Martinez, E., Maule, P., Hahmann, A. N., Cutululis, N. A., Sørensen, P. E., and Karagali, I.: Simulation of transcontinental wind and solar PV generation time series, Renew. Energ., 118, 425–436, https://doi.org/10.1016/j.renene.2017.11.039, 2018.
Nygaard, N. G. and Newcombe, A. C.: Wake behind an offshore wind farm observed with Dual-Doppler radars. J. Phys. Conf. Ser., 1037, https://doi.org/10.1088/1742-6596/1037/7/072008, 2018.
OECD: The Ocean Economy, OECD Publishing, available at: http://www.oecd.org/sti/the-ocean-economy-in-2030-9789264251724-en.htm (last access: 17 June 2019), 2016.
OWA: Offshore Wind Accelerator: Floating LiDAR Roadmap Update Deployments of Floating LiDAR Systems, Carbon Trust, 99 pp., available at: https://www.carbontrust.com/media/677598/uflr_d04_ floatinglidarrepository_210318_final-feb19_2.pdf (last access: 17 June 2019), 2018.
Peña, A. and Hahmann, A. N.: Atmospheric stability and turbulence fluxes at Horns Rev – an intercomparison of sonic, bulk and WRF model data, Wind Energy, 15, 717–731, https://doi.org/10.1002/we.500, 2012.
Peña, A. and Hahmann, A. N.: 30-year mesoscale model simulations for the “Noise from wind turbines and risk of cardiovascular disease” project, DTU Wind Energy E, 0055, 2017.
Peña, A., Hahmann, A. N., Hasager, C. B., Bingöl, F., Karagali, I., Badger, J., Badger, M., and Clausen, N.-E.: South Baltic Wind Atlas: South Baltic Offshore Wind Energy Regions Project, Risø-R-1775(EN), Risø National Laboratory for Sustainable Energy, Technical University of Denmark, 1–66, 2011.
Peña, A., Floors, R. R., Sathe, A., Gryning, S.-E., Wagner, R., Courtney, M., Larsén, X. G., Hahmann, A. N., and Hasager, C. B.: Ten Years of Boundary-Layer and Wind-Power Meteorology at Høvsøre, Denmark, Bound.-Lay. Meteorol., 158, 1–27, https://doi.org/10.1007/s10546-015-0079-8, 2015.
Petersen, E. L.: Wind resources of Europe (the offshore and coastal resources), EWEA special topic conference '92, 8–11 September 1992, Herning, Denmark, 1992.
Petersen, E. L. and Troen, I.: Wind conditions and resource assessment, Wires Energy Environ., 1, 206–217, https://doi.org/10.1002/wene.4, 2012.
Petersen, E. L., Troen, I., Jørgensen, H. E., and Mann, J.: The new European wind atlas, Energy Bulletin, 17, 34–39, 2014.
Pryor, S. C., Nielsen, M., Barthelmie, R. J., and Mann, J.: Can satellite sampling of offshore wind speeds realistically represent wind speed distributions? Part II Quantifying uncertainties associated with sampling strategy and distribution fitting methods, J. Appl. Meteorol., 43, 739–750, 2004.
Sempreviva, A. M., Barthelmie, R. J., and Pryor, S. C.: Review of Methodologies for Offshore Wind Resource Assessment in European Seas, Surv. Geophys., 29, 471–497, https://doi.org/10.1007/s10712-008-9050-2, 2008.
Skamarock, W. C.: Evaluating mesoscale NWP models using kinetic energy spectra, Mon. Weather Rev., 132, 3019–3032, 2004.
Skamarock, W. C., Klemp, J. B., Dudhia, J., Gill, D. O., Barker, D., Duda, M. G., Huang, X.-Y., Wang, W., and Powers, J. G.: A Description of the Advanced Research WRF Version 3, NCAR Technical Note NCAR/TN-475+STR, https://doi.org/10.5065/D68S4MVH, 2008.
Soukissian, T., Karathanasi, F., and Axaopoulos, P.: Satellite-Based Offshore Wind Resource Assessment in the Mediterranean Sea, IEEE J. Oceanic Eng., 42, 73–86, 2017.
The Crowne Estate: available at: http://marinedataexchange.co.uk/ItemDetails.aspx?id=4385 (last access: 26 October 2019), 2015.
Troen, I. and Petersen, E. L.: European Wind Atlas, Risø National Laboratory, Roskilde, 1989.
UK Renewables Atlas: available at: https://www.renewables-atlas.info/explore-the-atlas/ (last access: 17 June 2019), 2008.
Valldecabres, L., Nygaard, N. G., Vera-Tudela, L., Von Bremen, L., and Kühn, M. On the Use of Dual-Doppler Radar Measurements for Very Short-Term Wind Power Forecasts, Remote Sens., 10, 1701, https://doi.org/10.3390/rs10111701, 2018.
Wind Europe: Offshore Wind in Europe, Key trends and statistics 2018, available at: https://windeurope.org/wp-content/uploads/files/about-wind/statistics/WindEurope-Annual-Offshore-Statistics-2018.pdf (last access: 17 June 2019), 2018.
Witha, B., Hahmann, A. N., Sile, T., Dörenkämper, M., Ezber, Y,, Bustamante, E. G., Gonzalez-Rouco, J. F., Leroy, G., and Navarro, J.: Report on WRF model sensitivity studies and specifications for the mesoscale wind atlas production runs: Deliverable D4.3, vol. D4.3, NEWA – New European Wind Atlas, https://doi.org/10.5281/zenodo.2682604, 2019.
Witze, A.: World's largest wind-mapping project spins up in Portugal, Nature, 542, 282–283, 2017.