the Creative Commons Attribution 4.0 License.
the Creative Commons Attribution 4.0 License.
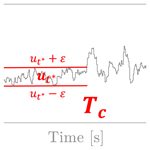
Periods of constant wind speed: how long do they last in the turbulent atmospheric boundary layer?
Daniela Moreno
Jan Friedrich
Matthias Wächter
Jörg Schwarte
Joachim Peinke
We perform a statistical analysis of the occurrence of periods of constant wind speed in atmospheric turbulence. We hypothesize that such periods of constant wind speed are related to characteristic wind field structures that, when interacting with a wind turbine, may induce particular dynamical responses. Therefore, this study focuses on characterizing the constant wind speed periods in terms of their lengths and probability of occurrence. Atmospheric offshore wind data are analyzed. Our findings reveal that long constant wind speed periods are an intrinsic feature of the marine atmospheric boundary layer (ABL). We confirm that the probability distribution of such periods of constant wind speeds follows a Pareto-like distribution, admitting power law behavior for periods exceeding the large-eddy-turnover time. The power law characteristics depend on the local conditions and the precise definition of wind speed thresholds. A comparison to wind time series generated with standard synthetic wind models and to time series from ideal stationary turbulence suggests that these structures are not characteristics of small-scale turbulence but seem to be consequences of larger-scale structures of the atmospheric boundary layer and thus are multi-scale. Given the results, we show that the continuous-time random walk (CTRW) model, as a non-standard wind model, can be adapted to generate time series of the wind speed whose statistics match the statistics of observed periods of constant wind speed.
- Article
(2153 KB) - Full-text XML
- BibTeX
- EndNote
Estimation of the loads experienced by a wind turbine (WT) is fundamental to decision-making processes during the design phase of various components of the machine, as well as for control strategies during its operation. Such estimation is performed through numerical modeling of the interaction between the WT and the incoming wind. Therefore, an accurate description of the wind within the atmospheric boundary layer (ABL) is essential to correctly calculate the loads acting on the WT. The International Electrotechnical Commission (IEC) has defined both the widely used standard parameters for the characterization of the atmospheric wind and the models for generating synthetic wind fields used for numerical estimation of loads on the WT (IEC, 2019). These IEC standards consider the spectral properties and coherence of the velocity components of the wind. Nevertheless, such guidelines are designed to mimic the atmospheric wind in a computationally efficient way. As a result, some flow features in the ABL are neglected or simplified in the characterization of atmospheric measured data, as well as in the generation of the synthetic wind fields. Furthermore, during the past decades, new challenges in the design process of WTs have emerged (Veers et al., 2019). On the one hand, trends in the design of modern WTs account for bigger rotor areas and less rigid structures (i.e., blades) to capture more energy from the available wind resources. On the other hand, the weight and material requirements of each component are being pushed to minimal levels. As a result, new WTs are becoming, in general, larger and less rigid. Therefore, some of the characteristics of the wind within the ABL that are not addressed in the IEC standard wind models might become relevant for the extra loads that were previously neglected within the design of smaller and stiffer WTs.
Based on cooperative research with a WT manufacturer, we hypothesized that one of these features, disregarded by the IEC guidelines, is the periods of constant wind speed (CWS) in atmospheric flows. Such periods are defined as the intervals of time over which the magnitude of the wind speed remains almost constant within a certain range, limited by a threshold value. In the following, we first contextualize the periods of CWS within the general characterization of turbulent features. Afterwards, we discuss the ways in which such CWS structures may be relevant for a WT.
Concerning the CWS periods as a general feature of the wind, we should mention that there are relevant and well-investigated turbulent quantities closely associated with our definition of CWS periods. This is the case for the persistence phenomenon, which characterizes how long the flow remains in a particular state before switching to another one. Persistence times can be inversely related to occurrence rates of extreme wind speeds or gusts. In this context, the exceedance statistics proposed by Rice (1944) have been applied to describe gusts as excursions at which certain thresholds of wind speed are exceeded (Kristensen et al., 1991; Young and Kristensen, 1992; Manshour et al., 2016). Another interpretation of persistence within turbulent flows is the zero-crossing analysis. In this case, for a zero-mean signal, the waiting times between two successive crossings of its zero level are evaluated. Statistical properties of zero crossings have been used to characterize intrinsic turbulent quantities such as the Taylor micro-scale (Narayanan et al., 1977; Sreenivasan et al., 1983; Kailasnath and Sreenivasan, 1993; Poggi and Katul, 2010) or the integral length scale (Mora and Obligado, 2020; Mazellier and Vassilicos, 2008). Analyses of zero crossings of velocity and temperature fluctuations in atmospheric turbulent data have been discussed (Cava and Katul, 2009; Cava et al., 2012; Chamecki, 2013; Chowdhuri et al., 2020). To summarize, the above-mentioned investigations showed that the statistical characteristics of the persistence for experimental and atmospheric data exhibit power law behavior up to a certain threshold, followed by log-normal or exponential cutoffs.
It is worth noting that even though the inter-arrival times of both excursions and zero crossings refer to structures between particular turbulent states, they do not correspond to the periods of reduced turbulent amplitudes in which we are interested. Further details of the differences between CWS periods and inter-arrival times between excursions and zero crossings are shown in Appendix A. Nevertheless, the method and statistics of such persistent events are relevant to the discussion. Of special interest are self-similar, critical, or fractal features of turbulence that propose power law behavior for the probability distribution of the time intervals with duration T, which can be formulated as (in particular for the limit of large T). A characteristic feature of a power law distribution is the absence of an intrinsic scale, i.e., the probability of observing a realization larger than ξT is times the probability of observing a realization larger than T, independent of the value of T. The long-tail regime of many distributions occurring in complex systems is assumed to exhibit power law behavior (Laherrere and Sornette, 1998). In the context of wind energy, for instance, a Pareto distribution has been tested as an extrapolation method to estimate extreme loads on a multi-megawatt wind turbine generator with a 1-month return period (Dimitrov, 2016).
Next, we discuss the potential relevance of an accurate description of the CWS periods for WT applications, which is directly linked to the increasing size and flexibility of the WTs. In the simplest case, such periods of CWS should imply relatively quiescent operating conditions for a WT when the CWS structure occurs homogeneously in the rotor area. A more entangled case might occur when resonant or near-resonant dynamics appear for specific periods of CWS over which the resonance can be strongly excited. In particular, for the larger WTs, the CWS periods may be restricted to a sub-area of the rotor plane. In this case, resonant dynamics exhibiting 3P oscillations may be amplified. Within this context, recent studies are devoted to interfaces between turbulent and non-turbulent states in atmospheric wind measured at typical WT heights (Neuhaus et al., 2024). Meanwhile, numerical and experimental investigations of the laminar–turbulent transition mechanisms on rotating wind turbine blades have shown changes in the transition characteristics over a single revolution, which affect the aerodynamic response of the WT (Lobo et al., 2023; Özçakmak et al., 2020).
As a last possible application for WTs, we want to mention that the statistical features of CWS periods may become of interest for probabilistic design methods. Although the methods proposed by the IEC for estimating WT loads are mostly deterministic (IEC, 2019), in recent years, probabilistic design methods have been introduced as surrogates for the design and load assessment of WTs (Abhinav et al., 2024; Kelma, 2024). Such probabilistic approaches produce more reliable estimations by considering the explicit calculation of the uncertainties from the operational conditions, aerodynamic models, materials, etc. (Sørensen and Toft, 2010). Characteristics of the wind are then defined as stochastic variables within the probabilistic model. Accordingly, broader and more accurate statistical descriptions of the intrinsic features of the wind inside the ABL account for a reduction in the uncertainty in the estimated loads and responses of the WTs.
In this paper, we focus on the periods of CWS as general features of turbulence; the discussion of possible impacts on a WT will be done only as side remarks. In particular, we characterize the statistics of periods of CWS (with a low level of turbulent fluctuations) from wind measurements in the ABL. In a preliminary investigation, the method for the assessment of such events from wind speed time series was presented, and the first results on the characterization of the periods of CWS in terms of their duration and probability distributions were also reported (Moreno et al., 2022). Special attention within the characterization was given to the tails of the distributions, which describe extremely long periods. Interestingly, we found that the probability distribution for very long periods shows a power law decay . Furthermore, a comparison with wind data generated by an IEC standard model revealed that the model underestimates the frequency of occurrence of the extremely long CWS periods measured in the ABL. In this study, we aim to address whether the CWS periods are induced by specific orographic perturbations, whether they are laminar or low-turbulence structures, and whether they are intrinsic features of a turbulent flow or rather result from large-scale interactions within the ABL. To characterize the CWS periods, we use data from offshore wind, as we expect them to have fewer special orographic effects compared to onshore data, and thus we can get more general insights into the CWS structure. This is also the motivation for the comparison of our results with ideal turbulent data from a free-jet experiment. Furthermore, a stochastic wind field model for WT simulations is presented as a surrogate approach to incorporate the statistics of long CWS periods from turbulence in the ABL.
The paper is structured as follows: Sect. 2 restates the method for measuring the periods and describes the atmospheric wind data to be analyzed. In Sect. 3, the results of the statistical characterization of the periods from the atmospheric data are shown. In Sect. 4, we compare the results from ABL data to those from two different data sets, i.e., the IEC standard wind model and experimental ideal turbulence. In Sect. 5, we present our conclusions and potential future work.
2.1 Definition of a period of CWS
Following Moreno et al. (2022), a CWS period (Tc) is defined as the time over which the magnitude of the wind speed u(t) exhibits low-amplitude fluctuations enclosed within certain thresholds. A period Tc is depicted in Fig. 1. Over the length of Tc, the wind speed remains inside the constant speed range (CSR). The CSR is defined as , where is the reference speed value at , and ε is the maximum acceptable magnitude of the fluctuations around . In Fig. 1, the horizontal red bars illustrate the thresholds that delineate the CSR. It should be noted that the CWS periods are not strictly laminar but are periods with a smaller amplitude of turbulence; see also the spectral analysis in Sect. 3.
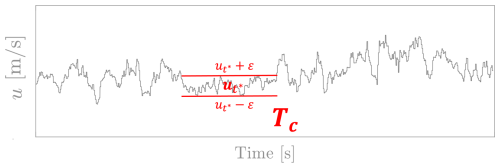
Figure 1 Schematic representation of a CWS period (Tc) measured from an exemplary wind speed time series u(t). The constant speed range (CSR), , specifies the limits for the accepted level of turbulence within a period Tc. The CSR is shown by the horizontal red bars.
In the following, the method for measuring the length of a period Tc at a given time step t* is described in detail. The goal is to count the number of N consecutive time steps, including t*, for which their wind velocity u(t) is contained inside the CSR. For that, the reference speed and the corresponding CSR, , are defined. Next, the velocities at the time step for …,∞) are evaluated and counted. The counter for the evaluation of is then defined as
Note that only consecutive points are counted in . The count is concluded once the value of exceeds either the bottom limit or the top limit of the CSR. So far, only points in the forward direction (+) from t* are evaluated. The same algorithm is subsequently applied to count the number of points in the backward direction from t*. In this case, values of are considered to evaluate in Eq. (1). Finally, the total number of consecutive points N measured at t* results from the sum of and , which are independently counted in their corresponding directions. The length of the period Tc at t* is then obtained by multiplying the total N by the size of the time step δt. A period Tc is estimated for every time step in the time series u(t). In the case of overlapping periods, only the longest period measured is recorded. By doing so, a recounting of events is avoided.
In Moreno et al. (2022), the threshold ε for fixing the CSR, , was randomly selected (e.g., 0.2–0.4 m s−1), and the method described in Eq. (1) was applied over the actual measurements u(t). However, limitations of the method appear when analyzing large data sets with very different mean wind speed and standard deviation σu, which are calculated over shorter time windows (i.e., 10 min) with respect to the length of the sample. To introduce a systematic approach, in this paper, the threshold ε is defined as proportional to the standard deviation of the wind speed σu. Then, ε to fix is calculated as
where A is a factor, typically A<1. The value of A can be chosen depending on the particular application. In the case of a WT, A might be related to the thresholds for the control system to operate within different turbulent regimes. In practice, such thresholds in the operating protocols are commonly defined as a function of the turbulence intensity TI . In Eq. (2) and through this paper, we refer to and σu as the mean and standard deviation values calculated over 10 min periods, unless a distinction is clearly stated.
2.2 Atmospheric wind data
Data from the offshore research platform FINO (Forschungsplattformen in Nord- und Ostsee) are investigated. We expect offshore wind to provide a better representation of undisturbed, or less disturbed, conditions within the ABL compared to onshore data. Therefore, the possible effects of onshore orographic conditions on the CWS structures are diminished.
Specifically, measurements at the FINO1 platform, located in the North Sea, are used. Records of the wind speed u(t) were taken by vertically aligned cup anemometers mounted at different heights H (FINO, 2025). The data correspond to measurements from January to December 2007, with a sampling frequency of 1 Hz. Measurements at heights H=[ 30, 50, 70, 90] m above the mean sea level are considered. Wind speed records have been limited to those 10 min periods with between 3 and 25 m s−1 due to their relevance for WT operation. Values of u(t) outside this range have been neglected. Moreover, to avoid disturbance from the met mast, data for wind directions between 275 and 350° are not considered. As an overview of the complete data set, Fig. 2 shows the mean and standard deviation σu calculated over individual 10 min periods at H=90 m.
3.1 Mean, standard deviation, and maximum value of Tc
As a starting point for the statistical characterization of the measured CWS periods Tc, we discuss their mean duration (), standard deviation (), and maximum value (Tc,max). We define Tc,max as a representative value from a set of the longest periods measured rather than the absolute and unique longest event. More details follow in Sect. 3.2. We compare the statistics of Tc at different heights H. A factor A=0.3 is chosen as an example to define the threshold ε=A σu for the CSR, . The results are summarized in Table 1.
Table 1Mean (), standard deviation (), and maximum length (Tc,max) of the calculated periods Tc at different heights H. A factor A=0.3 is assumed for the estimation of Tc.
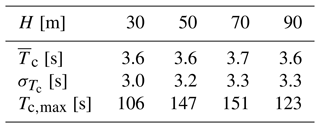
As a remark, special attention has to be devoted to the meaning of the statistical moments and calculated from the data. In certain cases, such as those presented in Moreno et al. (2022), the probability distribution p(Tc) may lead to non-converging moments, e.g., mean and variance. Further details are discussed in Appendix B and C. From the values in Table 1, comparable and are obtained for the four heights H. More interesting are the longest CWS periods measured Tc,max at each height H. Periods with lengths up to that correspond to more than 100 s are measured. The specific values of , , and Tc,max are expected to be dependent on the specific local conditions due to surface interactions. In particular, stronger differences in the lengths of CWS periods might arise under onshore conditions, as observed by Kelly (2024) when analyzing coastal flow accelerations at different heights.
3.2 Probability density function of Tc
Next, in the statistical characterization of the CWS periods, the probability density functions (PDFs) p(Tc) are discussed. Figure 3 shows p(Tc) for the data in Table 1 for different heights H. As mentioned before, we focus our attention on characterizing very long periods of Tc. Therefore we concentrate on the tails of p(Tc). For comparability, the values of Tc are normalized by the longest period measured at each H; more precisely, we use a representative value Tc,max of at least 10 of the longest periods to become more statistically robust. The values obtained for Tc,max are those summarized in Table 1.
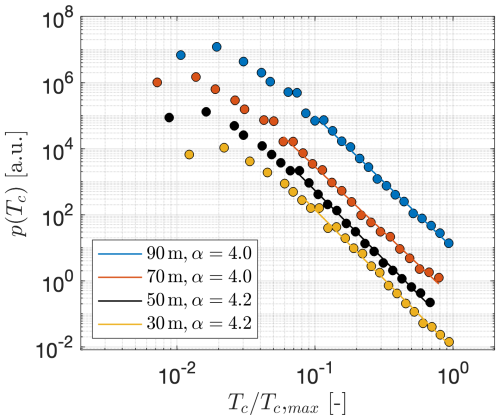
Figure 3Normalized probability density functions for FINO data at different heights H. The dots illustrate the results from the FINO data. The solid lines show the power law decay fitting . The value Tc,max for each height is defined as the bin center containing at least 10 of the longest periods measured after a binning process. The individual distributions are vertically shifted for better visualization.
The normalized PDFs p(Tc) in Fig. 3 are presented on a log–log scale. In such a representation, a straight line reveals power law behavior of the form , with α as the characteristic exponent. In Fig. 3, the power laws fitted over the tails of the distributions are shown by solid lines, with the same color used for the dots at each H. This indicates that the PDFs of CWS periods p(Tc) follow a Pareto-like distribution for large Tc (Laherrere and Sornette, 1998). We emphasize that the power laws extend over more than 1 decade. The corresponding exponents α are calculated following the procedure proposed by Clauset et al. (2009) and described in Appendix D. The values of α are given in the legends of the figure.
The variation in the exponent α for all H is within ±6 %.
This shows that the decay does not depend on the height. Moreover, since α≥3 for all H, the second-order statistical moments of Tc converge, and the results presented in Table 1 provide meaningful information about the characteristics of the periods Tc (see Appendix B and C).
The power law behavior observed in the distributions p(Tc) for the offshore data shown in Fig. 3 agrees with the data obtained for the two onshore sites investigated by Moreno et al. (2022), as well as for analyses performed on data from the mast Wettermast Hamburg (2025). This indicates that the CWS structures Tc are not due to the specific orographic conditions but rather represent general characteristics of the ABL. However, as the actual values of the statistics of CWS periods (i.e., , , Tc,max, α) vary significantly between data sets, they should be considered individually for each location.
3.3 Validity of the power law
To validate the universality of the power law distribution , we investigate the effect of the width of the CSR, . Different values of the factor A, such as ε=A σu, are evaluated. The results of , , Tc,max , and α for are summarized in Table 2. Figure 4 shows the normalized PDFs p(Tc) in an analogue representation, as shown previously in Fig. 3.
Table 2Mean (), standard deviation (), maximum length (Tc,max), and exponent α of the Tc periods calculated for different values of the factor A. FINO measurements at H=90 m are analyzed.
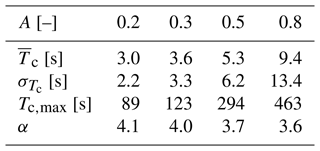
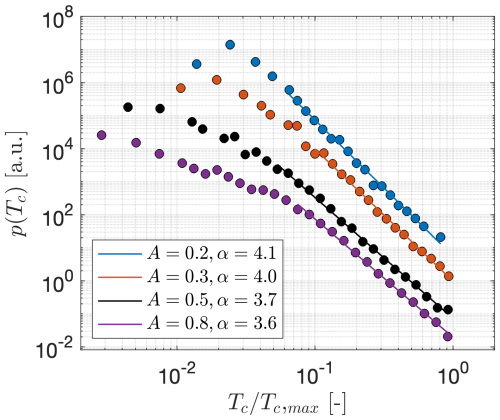
Figure 4Normalized probability density functions for FINO data for different values of A. The power law fittings are depicted by the solid lines. Measurements at H=90 m are considered. The value Tc,max for each value of A is defined as the bin center containing at least 10 of the longest periods measured after a binning process. The individual distributions are vertically shifted for better visualization.
The tails of the PDFs in Fig. 4 show a clear power law decay for all values of A. This confirms our hypothesis about the Pareto-like distributions of p(Tc) for large Tc, which has already been observed in Fig. 3. Interesting to note is that the exponent α decreases with increasing width of the CSR or of the factor A.
3.4 Power spectra of u(t) during periods Tc
Further in the characterization of the CWS periods, the spectral features of the wind speed u(t) during the CWS periods Tc address the question of whether the wind speed is strictly laminar or is instead turbulent with a low degree of turbulence. The turbulent nature of u(t) is now verified by the power spectra shown in Fig. 5. The spectra E(f) are calculated from the time series of u(t) extracted during CWS periods larger than 10 s. The time series u(t) are normalized by the standard deviation σu of their corresponding 10 min periods. A time window of roughly 5 d was considered to extract the definite time series u(t) during Tc>10 s. A decay of the form is obtained for all heights H. Accordingly, the wind data embedded along the periods Tc are not laminar flow sections but periods of turbulence with smaller amplitudes.
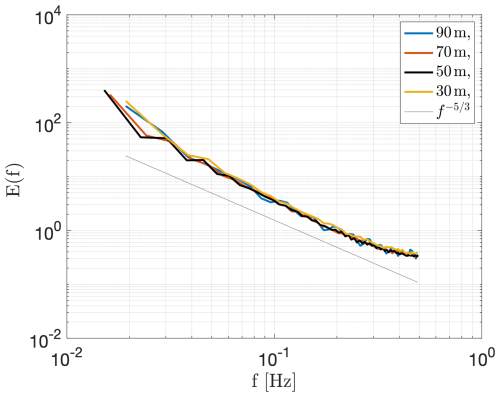
Figure 5Power spectra E(f) of normalized wind speed u(t) during the Tc periods measured at different heights H. The solid gray line shows a decay . The spectra are calculated for each period Tc and then averaged over all periods. A time window of roughly 5 d was considered to extract the definite time series u(t) during Tc>10 s.
4.1 Experimental wind-tunnel turbulence and IEC standard Gaussian Kaimal
In order to investigate whether the CWS periods are typical features of turbulent flow or are special features of the ABL, we investigate the statistics of the CWS periods Tc from experimental wind-tunnel turbulent data, as well as from synthetic data. The experimental data “Lab” were measured by Renner et al. (2001) in the central region of a free jet, which is approximately stationary, homogeneous, and isotropic. The synthetic data “Kaimal” correspond to IEC standard wind data based on the well-known Kaimal model, with normally distributed amplitudes (Kaimal et al., 1972). The Kaimal data are generated by the National Renewable Energy Laboratory (NREL) TurbSim package (Jonkman, 2016). Details about the parameters and characteristics of the two additional data sets, Lab and Kaimal, are given in Appendix E.
The analysis of the CWS periods from FINO and Kaimal can be easily compared, as the wind data sets u(t) have comparable IEC standard characteristics in terms of mean wind speed, standard deviation, sampling frequency, and integral length scale. However, such a match of parameters to atmospheric data is not possible with the experimental Lab data. To work out the intrinsic features of the periods of CWS from these different data, we used two different approaches to normalize the calculated Tc.
Firstly, the normalization is done by Tc,max, analogous to that in Figs. 3 and 4. The resulting normalized PDFs p(Tc) for the three wind data sets, FINO, Kaimal, and Lab, are shown in Fig. 6. For its interpretation, it is important to remark that the number of data points given by the sampling rate and measured time determines the lowest probability that can be resolved within the PDF. Accordingly, the minimum value of p(Tc) for Kaimal data in Fig. 6 is explained by the smaller amount of data in the sample. Oppositely, the high probability p(Tc) of shorter periods for Lab data is explained by a much higher sampling of the data.
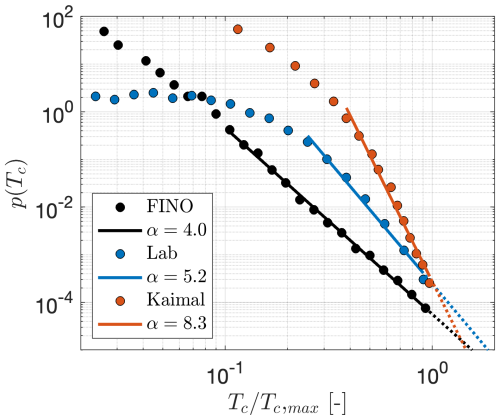
Figure 6Normalized probability density functions for the FINO, Kaimal, and Lab data sets. The power law fittings are depicted by the solid lines. The value Tc,max for each data set is defined after a binning process as the center of a bin containing at least 10 of the longest periods measured. Measurements at H=90 m are considered for FINO. The threshold ε=A σu for the CSR is calculated with A=0.3. The values of σu for Kaimal and Lab are 0.58 and 0.38 m s−1, respectively. In this particular case, as both data sets are expected to be steady, the standard deviation σu is calculated over the length of the time series.
Clearly different PDFs are observed for the three data sets in Fig. 6. The most prominent power law is found for the FINO data, with a smaller exponent α or more heavy-tailed probabilities. For the Kaimal and Lab data, a power law is questionable. We nevertheless show power laws as a reference for comparison between the three data sets. Interestingly, the range of periods Tc for which the power law holds for the FINO data extends over a decade, at least from to . In contrast, the power law range for Kaimal and Lab spreads only from to .
The normalization by Tc,max shown in Fig. 6 does not provide any information regarding the magnitude of the CWS periods Tc. Therefore, a comparison of absolute values Tc between the three data sets remains inconclusive. Accordingly, we chose a second approach to normalize the CWS periods so that their lengths are related to the intrinsic lengths of the flow. The integral length scale Lint is a measure of the longest correlations. For ideal turbulence, structures that are significantly larger than Lint are not to be expected. For meteorological wind data, the problem arises that at lower frequencies, no white noise (i.e., zero correlation) is present, so larger structures than Lint are expected (Sim et al., 2023; Larsén et al., 2016). Thus, we now normalize the periods Tc by the large-eddy-turnover time (Monin and Yaglom, 2007), where is calculated over the full time series for Kaimal and Lab data. The resulting PDFs p(Tc) after the second normalization approach are shown in Fig. 7.
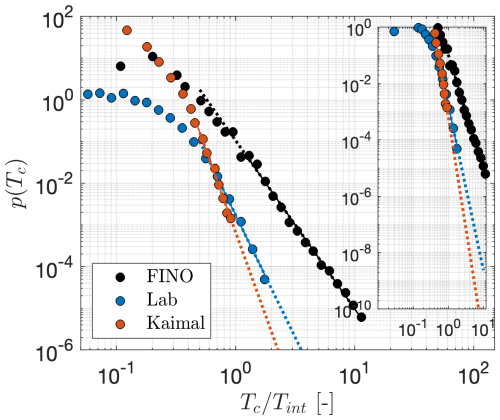
Figure 7Normalized probability density functions for the FINO, Kaimal, and Lab data sets. The values of Tint are 17 and 0.029 s for Kaimal and Lab, respectively (Fuchs et al., 2022). For FINO, Tint is set to 10 s, as a representative value of the atmospheric data. The power law fittings are depicted by the solid lines. The dotted lines show the power law fittings extended over a range of Tc larger than the range used for calculating the fitting parameters.
Figure 7 shows that the FINO data have significantly longer CWS periods Tc. It is observed that the maximal CWS event of the data from the Gaussian Kaimal model, Tc≈ Tint, is around 100 times more frequent for the FINO data than for the other two data sets. Assuming the extended power law tails for Kaimal and Lab depicted by the dotted lines and better visualized in the zoomed plot, a period Tc≈10 Tint would be around 104 times less probable in the Kaimal and Lab data compared to the measured FINO data. From the 1-year FINO data, we measured 15 events Tc≈10 Tint (with ). It means that there was an observation Tc≈10 Tint roughly every 24 d. Under the IEC Kaimal Gaussian assumption, this event will appear once every 66×104 d or 1808 years.
Furthermore, we calculate the standard deviation of the periods in units of integral lengths Lint. The resulting values are , , and . The estimated values of show in another way that FINO data tend to have remarkably longer periods compared to Kaimal and Lab data.
4.2 CTRW wind model
We have shown the results of the distributions of CWS periods p(Tc) in the ABL and their underestimation by the IEC standard Gaussian Kaimal wind model. Consequently, we finally show how the observed features of the atmospheric turbulent data can be included in a numeric wind field model. As a surrogate for the IEC standard Kaimal model, we investigate non-standard wind velocity time series generated by the continuous-time random walk (CTRW) model (Kleinhans, 2008; Ehrich, 2022; Schwarz et al., 2019; Mücke et al., 2011). The CTRW model generates either Gaussian CTRW-G ( with G as an abbreviation for Gaussian) or non-Gaussian CTRW-NG ( with NG as the abbreviation for non-Gaussian) wind velocity time series. For the CTRW-G, the statistics of u(t) are entirely Gaussian. On the contrary, the statistics of u(t) for the CTRW-NG deviate from Gaussianity towards distributions with heavy tails or higher probabilities of rare or extreme events.
The CTRW model is based on a skewed Lévy-distributed stochastic process parameterized by the characteristic exponent αL. The stochastic process defines a time transformation from the intrinsic scale of the model s to the physical time t. Such time-scaling transformation allows the generation of non-Gaussian time series u(t). The characteristic exponent αL, with 0 , specifies the asymptotic behavior of the skewed Lévy distribution. For αL = 1, the resulting process u(t) is entirely Gaussian. Values of αL→0 generate processes with more pronounced non-Gaussian characteristics. In this case, non-Gaussianity is related to extremely long waiting times between two successive time steps s. A very long waiting time in u(s) would then be translated into a period over which the process u(t) remains constant.
Figure 8 shows an excerpt of u(t) for the Gaussian CTRW-G and non-Gaussian CTRW-NG realizations. Values of αL=1 and αL=0.9 are considered for CTRW-G and CTRW-NG, respectively. Along the interval between t=875 and t=895 s, a period of almost constant wind speed is observed for the CTRW-NG. For better visualization, a zoomed version of the time series is presented in the sub-panel in the bottom-right corner. Such a structure of the wind, indicated by the horizontal blue line, agrees with our definition of a CWS period Tc. The small fluctuations observed within the CWS period result from the interpolation process between the intrinsic and the physical times s→t (Ehrich, 2022).
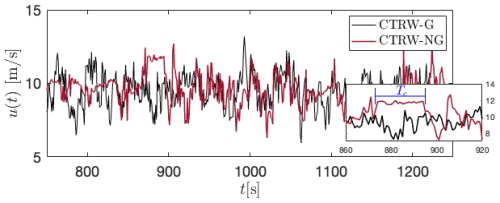
Figure 8Excerpt of the wind speed time series u(t) for CTRW-G and CTRW-NG. A CWS period Tc is visible between 875 and 895 s in the CTRW-NG. The exponent for the Lévy distribution of the CTRW-NG is αL=0.9.
The fundamentals of the CTRW model as well as further details on the method for achieving such non-Gaussian features are given in Appendix F. The parameters for generating the time series are provided in Appendix E.
Figure 9a shows the PDFs p(Tc) for the CTRW realizations and the FINO data. The individual distributions are vertically shifted for better visualization. The dotted lines show the Gaussian distributions, with the mean and standard deviation of the corresponding p(Tc). The gray-shadowed area illustrates the range of the decay of p(Tc) or slopes α enclosed by CTRW-G (triangles) with αL=1 and CTRW-NG (squares) with αL=0.9. The distribution of the CTRW-NG realization shows an overestimation compared to the FINO data; there is a deviation from Gaussianity towards a higher probability of very long periods of Tc. This deviation is visible in . On the contrary, the decay of the CTRW-G is much more pronounced, and the divergence from the Gaussian distribution is visible only for events . A third realization, CTRW-NG* (black circles), with αL=0.995 is included. The resulting p(Tc) distribution for CTRW-NG* shows better agreement with the FINO data. Both distributions, FINO and CTRW-NG*, lie inside the gray-shadowed area depicting the slopes enclosed between the Gaussian CTRW-G and extremely non-Gaussian CTRW-NG.
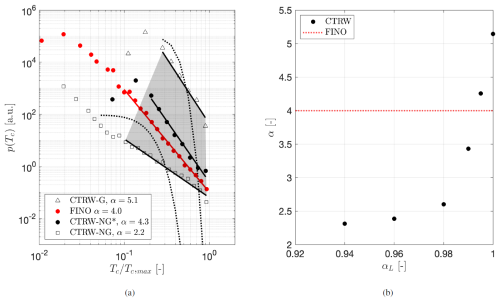
Figure 9(a) Normalized probability density functions for the CTRW-G, CTRW-NG, and CTRW-NG* and for the FINO data. The gray area depicts the range of the slopes covered between CTRW-G and CTRW-NG. Measurements at H=90 m are considered for FINO. The individual distributions are shifted vertically for better visualization. Dotted lines depict Gaussian distributions. (b) Power law exponents α from as a function of the characteristic exponent αL from the Lévy distribution of the CTRW model. The horizontal red line depicts the value of α for the FINO data shown in (a).
Figure 9b shows the resulting exponents α from the decay versus the exponent αL from the Lévy distribution of the CTRW model. The dotted horizontal line depicts the value of α for FINO in Fig. 9a. As observed, by tuning the αL parameter of the CTRW model, non-Gaussian realizations of u(t) can reproduce the statistics of p(Tc) from turbulent wind in the ABL. Since the resulting distributions p(Tc) are quite sensitive to the Lévy exponent αL, a careful selection of the exponent is required.
We present measurements of the CWS periods (Tc) (periods with turbulence of a reduced amplitude) from offshore wind data within the ABL. It is shown that the probability distributions p(Tc) for offshore data exhibit a power law decay for very long events (i.e., hundreds of seconds). This agrees with Moreno et al. (2022), where preliminary results from onshore cases were reported. However, significant differences in the values of the exponent α between offshore and onshore conditions suggest that the lengths of Tc are indeed influenced by interactions with the surroundings. Therefore, the estimated statistics of Tc must be considered locally for the specific location of interest. Given that offshore conditions maintain a more unperturbed ABL compared to those onshore, we demonstrated that the periods Tc are intrinsic features of the ABL rather than structures resulting from specific external factors (i.e., mountains, obstacles). Moreover, the exponent α seems to be quite independent of the height but changes significantly with the threshold ε. Less pronounced decays of p(Tc) are obtained with wider thresholds when considering the wind speed to be constant. We found examples of Tc significantly larger than 100 s, which correspond to spatially extended structures over sizes larger than 1 km, using Taylor's hypothesis of frozen turbulence. Such large structures in turbulent wind may be related to the current hot topic of “turbulent superstructures” (Pandey et al., 2018; Krug et al., 2020; Käufer et al., 2023).
Based on the spectral properties, we proved the turbulent nature of the wind speed u(t) during the CWS periods Tc. This relates our results to the case of the turbulent–turbulent interfaces (Kankanwadi and Buxton, 2022). However, the statistics of Tc deviate significantly when comparing different turbulent data. Results from experimental homogeneous isotropic turbulence data suggest that the nature of the periods Tc is attributed to special structures developing in the wind inside the ABL. It is still an open question whether they are caused by special effects of the small-scale turbulence (such as turbulence with or without shear) or whether they are indeed consequences of larger-scale interactions of the atmospheric boundary layer, such as phenomena related to the spectral gap (Larsén et al., 2016).
The frequency of very long events Tc in the ABL is significantly underestimated by the Gaussian assumptions in the IEC models. Therefore, the need for an improved wind model is justified. The continuous-time random walk (CTRW) model, with its characteristic time mapping (see Appendix F), is particularly suitable for the incorporation of the periods Tc measured in the turbulent atmospheric wind. By tuning the exponent of the intrinsic Lévy distribution, different statistics of very long CWS periods can be obtained. This surrogate wind model represents an improvement towards more realistic atmospheric wind fields for numerical simulations. Consequently, results of the WT on the wind when interacting with such disregarded structures might be better predicted.
From an engineering perspective, very long CWS periods might be undesirable for the operation of WTs if phenomena such as resonance or critical loading are induced. On the other hand, they also might be beneficial if conditions such as constant power production are achieved. Further research is needed on the detailed effects of CWS periods on loads by investigating specific WT models.
A very long CWS period might have an increased impact on a WT depending on its spatial location in the plane of the rotor. The effect of such an event happening in the outer region of the rotor plane might be higher compared to the case when it reaches the turbine at the region near the hub. Accordingly, preliminary investigations (detailed in Appendix G) suggest that the periods of CWS show a tendency to be localized at different measurement heights and, therefore, may become of particular interest for turbines with larger diameters. Future work has to be devoted to assessing the relevance of the empirically observed power law behavior of periods of CWS on turbine loading. For that, the complete statistical parameterization of periods of CWS, in both the temporal and spatial domains, should be assessed and improved for the synthetic wind field models such as the proposed CTRW model (Kleinhans, 2008); the recently introduced time-mapped Mann model (Yassin et al., 2023), which can generate long waiting times of u(t) as in the CTRW model; or the super-statistical model (Friedrich et al., 2021, 2022) that follows the K62 model of turbulence. Another interesting aspect for future work would be the statistical analysis of CWS periods from weather-modeled data (e.g., the European Center for Medium-Range Weather Forecasts (ECMWF) and Weather Research and Forecasting (WRF) models). The results would reveal whether such larger-scale models can reproduce the CWS structures within atmospheric forecasting.
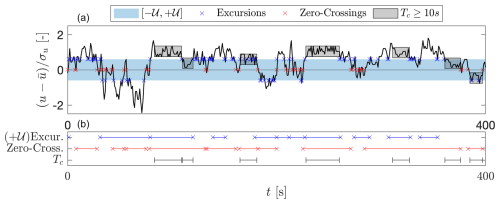
Figure A1Illustration of excursions, zero crossings, and CWS periods (Tc). Panel (a) is a normalized signal . The blue crosses depict the excursions, considering ±𝒰 as thresholds. The red crosses correspond to the zero crossings. The gray rectangles mark periods of CWS Tc>10 s. The blue and red lines in (b) depict a selection of the resulting inter-arrival times for the excursion measured at the upper limit +𝒰 and the zero crossings, respectively. Only the inter-arrival periods longer than 10 s are shown. For comparison, the periods Tc>10 s are re-plotted as black lines.
In the Introduction (Sect. 1), we referred to the inter-arrival times of excursions and zero crossings as two general turbulent characteristics within the context of persistence phenomena. Those inter-arrival times might wrongly be assumed to be intrinsically related to our periods of CWS. As shown in Fig. A1, fundamental differences arise when comparing the three events within a turbulent signal. In Fig. A1, a zero-mean and normalized-by-standard-deviation signal is plotted. The thresholds ±𝒰 are fixed for considering the excursions of the signal. The blue area depicts the range contained inside these thresholds. The excursion events are depicted by blue crosses. Similarly, the zero crossings are depicted by red crosses. The gray rectangles depict the measured CWS periods Tc>10 s. We assume ε=0.3 for measuring Tc.
In Fig. A1, the length of selected inter-arrival times between the excursion and zero-crossing events and the periods Tc are plotted. The lines follow the color code in panel (a). The selected inter-arrival times are only those longer than 10 s, as was assumed for the periods Tc. As an additional criterion for the excursions (blue lines), only inter-arrival times between successive excursions at the upper limit +𝒰 are considered (i.e., events at −𝒰 are neglected). Note that if all the inter-arrival times were plotted without any distinction, then the individual blue and red lines would overlap, covering the entire length of the time series.
As observed, there is no direct correlation between the occurrence or the length of the CWS periods and the inter-arrival times, neither between excursions nor between zero crossings. A CWS period might enclose several inter-arrival times, and several CWS structures might be embedded inside an interval between consecutive zero crossings or excursions.
A general quantity x with a probability distribution p(x) follows a power law if
for x≥xmin, with the characteristic exponent α and a constant C=ec. The minimum value xmin holds for the lowest limit of the power law. The exponent α>1, otherwise , does not converge. The estimation of α from empirical data has been extensively discussed in the analysis of the distributions of a very wide range of applications (Newman, 2005; Clauset et al., 2009). Since Eq. (B1) is equivalent to , the most simple approach for the calculation of α comes from a linear regression on the log–log plot of the histogram of x. However, this procedure introduces significant errors due to the binning of the data and the resulting distributions. Such distributions are usually dominated by a few bins at lower values of x with very high values of p(x) and several bins in the higher range of x with very low probabilities of p(x) (Newman, 2005; Dorval, 2008). Instead of such a linear regression, a logarithmic binning process of the data is recommended. Within this approach, the histogram of x is constructed for k number of bins with variable widths. More specifically, the bin edges B are proportional to successive powers of a constant a. Then,
where b1>0, k>1, and xmin is the minimum value of x to consider the power law behavior. Thus, the ith bin encloses the interval , and the larger edge of the kth is assumed to be +∞.
The value of the lower bound xmin affects the estimation of the exponent α in . Analogously, for binned data, bmin is defined as the minimum bin taken into consideration for the calculation of α. We follow the algorithm proposed by Clauset et al. (2009) and Virkar and Clauset (2014) to choose bmin from binned empirical data. This method is based on a Kolmogorov–Smirnov (KS) statistic test (Massey, 1951) to minimize the distance between the distributions of the fitted model and the empirical model S(b) above bmin. Then, the optimized value of minimizes
Further details about the method for calculating bmin and α are provided in Appendix D.
A power law distribution of a continuous variable x is defined in Eq. (B1), where α>1 is the power law exponent, C is a normalization constant, and xmin is the minimum value at which the power law holds. Then, the kth statistical moment of a power law distribution is given by
Then, a quantity x with may have divergent moments. Its general kth moment exists only if . The mean value of p(x) or 〈x1〉 becomes infinite for α≤2. Furthermore, if α≤3, p(x) has no finite variance, 〈x2〉. In such a case, x can take values of . Many phenomena, varying from biological to economical, are characterized by such critical distributions. A few examples are the frequency of the use of words, the income among individuals, and the magnitude of earthquakes (Newman, 2005; Marquet et al., 2005; Powers, 1998).
Here we describe the method introduced in Appendix B for estimating the minimum bin bmin, above which the power law is valid. The method was proposed by Virkar and Clauset (2014).
For each possible , we
-
calculate the cumulative binned empirical distribution S(b) for bins b≥bmin,
-
estimate the characteristic exponent considering b≥bmin,
-
calculate the cumulative density function (CDF) for of the binned power law,
-
calculate the Kolmogorov–Smirnov (KS) test statistic D defined in Eq. (B3), and
-
select the optimal value as the value of bmin with the minimum test statistic D.
The bins b are defined according to Eq. (B2). For the estimation of in step (2), a least-squares linear regression method is considered.
-
Kaimal. The data set contains 4×105 data points with a frequency of 1 Hz. The implementation of the Kaimal spectrum for the longitudinal component u of the wind in TurbSim (Jonkman, 2016) follows
where σu is the standard deviation, is the mean at the hub height, and f is the frequency. The integral scale Lu is defined as Lu=8.10Λu, with Λu being the turbulence scale. Λu is calculated as , where HH is the hub height. The parameters are chosen to be comparable to the averaged values of FINO data (see Sect. 2.2). We assume a hub height of HH=90 m, a mean wind speed of 10 m s−1, and a standard deviation σu of 0.58 m s−1. Then, the integral length scale is set to 170 m.
-
CTRW. Both realizations, CTRW-G and CTRW-NG, have 4×105 data points, with a frequency of 1 Hz. The mean wind speed and standard deviation are 9.5 and 1.1 m s−1 for both cases. Extended parameters for the model are ωc=1.8 Hz, , and . Details about the definition of the parameters are given in Appendix F and by Ehrich (2022). The values of the parameters are chosen to generate data comparable to FINO measurements (see Sect. 2.2).
-
Lab. The velocity in the direction of the flow was measured by a hot-wire anemometer. The data set consists of 8.48×106 data points, with a sampling frequency of 8 kHz. The measured integral length scale is reported as 0.067 m (Fuchs et al., 2022). Details of the experiment are found in Renner et al. (2001).
More detailed descriptions of the model are provided by Kleinhans (2008), Yassin et al. (2023), Mücke et al. (2011), and Schwarz et al. (2019). Time series of the wind speed at each point i of a defined grid are based on two coupled Ornstein–Uhlenbeck (OU) stochastic processes, and . Both processes are first generated in an intrinsic scale s. The super index κ accounts for the three directions of the wind . In our case, we generate wind speed time series only in the longitudinal direction u(x) such that κ=(x). The two processes are defined as
and
where γ and γr are damping constants, D and Dr are diffusion constants, and Γ(s) and Γr(s) are Gaussian-distributed white noise. Next, the resulting Gaussian velocity signals are mapped to the physical timescale t by means of an additional stochastic process as
where is a Lévy-distributed process with a characteristic exponent αL and a cutoff value . In the case of αL=1, the intrinsic scale s is equivalent to the physical time t such that = . The time-mapping process described in Eq. (F3) allows the key feature of the model, which accounts for the intermittent behavior of the wind speed time series. The intermittency is introduced by the Lévy-distributed sizes of the waiting times for the transformation from s to t.
In Sect. 4, we investigated two CTRW data sets: CTRW-G and CTRW-NG. For the CTRW-G time series shown in Figs. 8 and 9a, the Lévy exponent αL is equal to 1 such that the waiting times of the intrinsic scale s are constant and the statistics of u(t) are Gaussian. For the CTRW-NG time series, we assumed αL=0.9. By doing so, we introduce non-Gaussian features into the probability distributions. Further values of the parameters for generating the fields are given in Appendix E.
The spatial coherence of the CWS periods has been preliminarily investigated. Figure G1 shows the results of evaluating the simultaneity of events Tc>Tmin occurring at different heights of the FINO data and conditioned on a reference height . As an example, Fig. G1 shows the case when considering the reference height m and Tmin=30 s. Then, for each event Tc>30 s at 90 m, the occurrence of simultaneous events Tc at the remaining heights H is evaluated. A black line is drawn when an event Tc is measured at the corresponding H.
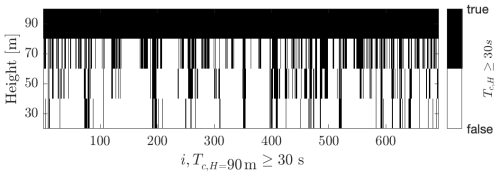
Figure G1Events Tc>Tmin at different heights, conditioned on m. First, the reference height is defined. Next, for each i event at , the occurrence of Tc at the remaining heights m is evaluated. Black lines depict the occurrence of an event. The Tc at all heights H is conditioned so that Tc>Tmin. For the example in this figure, Tmin=30 s and m.
The results show that most of the events are not coherent over the four heights H and confirm the appearance of localized structures. In fact, for the example shown, 37 % of the events at H=90 m are happening simultaneously at H=70 m. This number decreases to 11 % when comparing the CWS periods between H=90 m and H=30 m. The same evaluation for coherent events has been performed for different values of Tmin and reference heights .
The code for the algorithm described in Sect. 2.1 to measure the CWS periods from wind speed data can be provided upon request.
The FINO and Lab measurements, as well as the generated Kaimal and CTRW time series, can be obtained upon request.
DM – development of the code to measure the periods of constant wind speed from different data sets, generation of the synthetic wind data, analysis of the data, and writing the core of the paper. JF and MW – review, analysis, discussion of the results, and contributions to the text. JS – discussion of the results from the manufacturer/operator perspective. JP – extensive understanding of the method, analysis of the results, supervision, and reviewing and editing of the text.
At least one of the (co-)authors is a member of the editorial board of Wind Energy Science. The peer-review process was guided by an independent editor, and the authors also have no other competing interests to declare.
Publisher’s note: Copernicus Publications remains neutral with regard to jurisdictional claims made in the text, published maps, institutional affiliations, or any other geographical representation in this paper. While Copernicus Publications makes every effort to include appropriate place names, the final responsibility lies with the authors.
We gratefully appreciate the valuable discussions with our partners, the Institute for Mechanical and Industrial Engineering Chemnitz and Nordex Energy SE, who are involved in the PASTA project (precise design methods of complex coupled vibration systems of modern wind turbines in turbulent conditions). The current paper was initiated to address the challenges discussed within the project.
This research has been supported by the Bundesministerium für Wirtschaft und Klimaschutz (grant no. 03EE2024) and the European Union (grant no. 101084205).
This paper was edited by Alfredo Peña and reviewed by two anonymous referees.
Abhinav, K. A., Sørensen, J. D., Hammerum, K., and Nielsen, J. S.: Probabilistic Design Methods for Gust-Based Loads on Wind Turbines, Energies, 17, 1518, https://doi.org/10.3390/en17071518, 2024. a
Cava, D. and Katul, G.: The Effects of Thermal Stratification on Clustering Properties of Canopy Turbulence, Bound.-Lay. Meteorol., 130, 307–325, https://doi.org/10.1007/s10546-008-9342-6, 2009. a
Cava, D., Katul, G., Molini, A., and Elefante, C.: The role of surface characteristics on intermittency and zero-crossing properties of atmospheric turbulence, J. Geophys. Res.-Atmos., 117, D01104, https://doi.org/10.1029/2011JD016167, 2012. a
Chamecki, M.: Persistence of velocity fluctuations in non-Gaussian turbulence within and above plant canopies, Phys. Fluids, 25, 115110, https://doi.org/10.1063/1.4832955, 2013. a
Chowdhuri, S., Kalmár-Nagy, T., and Banerjee, T.: Persistence analysis of velocity and temperature fluctuations in convective surface layer turbulence, Phys. Fluids, 32, 076601, https://doi.org/10.1063/5.0013911, 2020. a
Clauset, A., Shalizi, C. R., and Newman, M. E. J.: Power-Law Distributions in Empirical Data, SIAM Rev., 51, 661–703, 2009. a, b, c
Dimitrov, N.: Comparative analysis of methods for modelling the short-term probability distribution of extreme wind turbine loads, Wind Energy, 19, 717–737, 2016. a
Dorval, A.: Probability distributions of the logarithm of inter-spike intervals yield accurate entropy estimates from small datasets, J. Neurosci. Meth., 173, 129–139, https://doi.org/10.1016/j.jneumeth.2008.05.013, 2008. a
Ehrich, S.: Analysis of the effect of intermittent wind on wind turbines by means of CFD, PhD thesis, Carl von Ossietzky Universität Oldenburg, http://oops.uni-oldenburg.de/5650/1/ehrana22.pdf (last access: 29 January 2025), 2022. a, b, c
FINO: FINO1: Forschungsplattformen in Nord- und Ostsee, https://www.fino1.de/en/, last access: 29 January 2025. a
Friedrich, J., Peinke, J., Pumir, A., and Grauer, R.: Explicit construction of joint multipoint statistics in complex systems, J. Phys. Complexity, 2, 045006, https://doi.org/10.1088/2632-072x/ac2cda, 2021. a
Friedrich, J., Moreno, D., Sinhuber, M., Wächter, M., and Peinke, J.: Superstatistical Wind Fields from Pointwise Atmospheric Turbulence Measurements, PRX Energy, 1, 023006, https://doi.org/10.1103/PRXEnergy.1.023006, 2022. a
Fuchs, A., Kharche, S., Patil, A., Friedrich, J., Wächter, M., and Peinke, J.: An open source package to perform basic and advanced statistical analysis of turbulence data and other complex systems, Phys. Fluids, 34, 101801, https://doi.org/10.1063/5.0107974, 2022. a, b
IEC: 61400-1 Wind energy generation systems, Standard, International Electrotechnical Commission, ISBN 978-2-8322-6253-5, 2019. a, b
Jonkman, B.: TurbSim User's Guide V2.00.00, Tech. rep., National Renewable Energy Laboratory, https://www.nrel.gov/wind/nwtc/assets/downloads/TurbSim/TurbSim_v2.00.pdf (last access: 29 January 2025), 2016. a, b
Kailasnath, P. and Sreenivasan, K. R.: Zero crossings of velocity fluctuations in turbulent boundary layers, Phys. Fluids A-Fluid, 5, 2879–2885, https://doi.org/10.1063/1.858697, 1993. a
Kaimal, J., Wyngaard, J., Izumi, Y., and Coté, O.: Spectral characteristics of surface-layer turbulence, Q. J. Roy. Meteor. Soc., 98, 563–589, 1972. a
Kankanwadi, K. S. and Buxton, O. R.: On the physical nature of the turbulent/turbulent interface, J. Fluid Mech., 942, A31, https://doi.org/10.1017/jfm.2022.388, 2022. a
Käufer, T., Vieweg, P. P., Schumacher, J., and Cierpka, C.: Thermal boundary condition studies in large aspect ratio Rayleigh–Bénard convection, Eur. J. Mech. B-Fluid., 101, 283–293, 2023. a
Kelly, M.: Flow acceleration statistics: a new paradigm for wind-driven loads, towards probabilistic turbine design, Wind Energ. Sci. Discuss. [preprint], https://doi.org/10.5194/wes-2024-69, in review, 2024. a
Kelma, S.: Probabilistic design of support structures for offshore wind turbines by means of non-Gaussian spectral analysis, PhD thesis, Gottfried Wilhelm Leibniz Universität, Hannover, https://doi.org/10.15488/15784, 2024. a
Kleinhans, D.: Stochastische Modellierung komplexer Systeme, PhD thesis, Westfälischen Wilhelms-Universität Münster, https://miami.uni-muenster.de/Record/2af675c2-594f-4e38-b206-aaf8e800c4d5 (last access: 29 January 2025), 2008. a, b, c
Kristensen, L., Csanova, M., Courtney, M. S., and Troen I.: In search of a gust definition, Bound.-Lay. Meteorol., 55, 91–107, https://doi.org/10.1007/BF00119328, 1991. a
Krug, D., Lohse, D., and Stevens, R. J. A. M.: Coherence of temperature and velocity superstructures in turbulent Rayleigh–Bénard flow, J. Fluid Mech., 887, A2, https://doi.org/10.1017/jfm.2019.1054, 2020. a
Laherrere, J. and Sornette, D.: Stretched exponential distributions in nature and economy: “fat tails” with characteristic scales, Eur. Phys. J. B, 2, 525–539, 1998. a, b
Larsén, X. G., Larsen, S. E., and Petersen, E. L.: Full-Scale Spectrum of Boundary-Layer Winds, Bound.-Lay. Meteorol., 159, 349–371, https://doi.org/10.1007/s10546-016-0129-x, 2016. a, b
Lobo, B. A., Özçakmak, Ö. S., Madsen, H. A., Schaffarczyk, A. P., Breuer, M., and Sørensen, N. N.: On the laminar–turbulent transition mechanism on megawatt wind turbine blades operating in atmospheric flow, Wind Energ. Sci., 8, 303–326, https://doi.org/10.5194/wes-8-303-2023, 2023. a
Manshour, P., Anvari, M., Reinke, N., Sahimi, M., and Tabar, M. R.: Interoccurrence time statistics in fully-developed turbulence, Sci. Rep., 6, 27452, https://doi.org/10.1038/srep27452, 2016. a
Marquet, P., Quiñones, R. A., Abades, S., Labra, F., Tognelli, M., Arim, M., and Rivadeneira, M.: Scaling and power-laws in ecological systems, J. Exp. Biol., 208, 1749–1769, https://doi.org/10.1242/jeb.01588, 2005. a
Massey, F. J.: The Kolmogorov-Smirnov Test for Goodness of Fit, J. Am. Stat. Assoc., 46, 68–78, https://doi.org/10.2307/2280095, 1951. a
Mazellier, N. and Vassilicos, J.: The turbulence dissipation constant is not universal because of its universal dependence on large-scale flow topology, Phys. Fluids, 20, 015101, https://doi.org/10.1063/1.2832778, 2008. a
Monin, A. S. and Jaglom, A. M.: Statistical fluid dynamics: mechanics of turbulence, edited by: Lumley, J. L., The MIT Press, Cambridge, Mass, ISBN 0262130629, 1979. a
Mora, D. O. and Obligado, M.: Estimating the integral length scale on turbulent flows from the zero crossings of the longitudinal velocity fluctuation, Exp. Fluids, 61, 199, https://doi.org/10.1007/s00348-020-03033-2, 2020. a
Moreno, D., Friedrich, J., Wächter, M., Peinke, J., and Schwarte, J.: How long can constant wind speed periods last in the turbulent atmospheric boundary layer?, J. Phys. Conf. Ser., 2265, 022036, https://doi.org/10.1088/1742-6596/2265/2/022036, 2022. a, b, c, d, e, f
Mücke, T., Kleinhans, D., and Peinke, J.: Atmospheric turbulence and its influence on the alternating loads on wind turbines, Wind Energy, 14, 301–316, https://doi.org/10.1002/we.422, 2011. a, b
Narayanan, M. A. B., Rajagopalan, S., and Narasimha, R.: Experiments on the fine structure of turbulence, J. Fluid Mech., 80, 237–257, https://doi.org/10.1017/S0022112077001657, 1977. a
Neuhaus, L., Wächter, M., and Peinke, J.: The fractal turbulent–non-turbulent interface in the atmosphere, Wind Energ. Sci., 9, 439–452, https://doi.org/10.5194/wes-9-439-2024, 2024. a
Newman, M.: Power laws, Pareto distributions and Zipf's law, Contemp. Phys., 46, 323–351, https://doi.org/10.1080/00107510500052444, 2005. a, b, c
Özçakmak, Ö. S., Madsen, H. A., Sørensen, N. N., and Sørensen, J. N.: Laminar-turbulent transition characteristics of a 3-D wind turbine rotor blade based on experiments and computations, Wind Energ. Sci., 5, 1487–1505, https://doi.org/10.5194/wes-5-1487-2020, 2020. a
Pandey, A., Scheel, J. D., and Schumacher, J.: Turbulent superstructures in Rayleigh-Bénard convection, Nat. Commun., 9, 2118, https://doi.org/10.1038/s41467-018-04478-0, 2018. a
Poggi, D. and Katul, G.: Evaluation of the Turbulent Kinetic Energy Dissipation Rate Inside Canopies by Zero- and Level-Crossing Density Methods, Bound.-Lay. Meteorol., 136, 219–233, https://doi.org/10.1007/s10546-010-9503-2, 2010. a
Powers, D. M. W.: Applications and Explanations of Zipf's Law, in: New Methods in Language Processing and Computational Natural Language Learning, ISBN 0725806346, 1998. a
Renner, C., Peinke, J., and Friedrich, R.: Experimental indications for Markov properties of small-scale turbulence, J. Fluid Mech., 433, 383–409, https://doi.org/10.1017/S0022112001003597, 2001. a, b
Rice, S. O.: Mathematical analysis of random noise, AT&T Tech. J., 23, 282–332, https://doi.org/10.1002/j.1538-7305.1944.tb00874.x, 1944. a
Schwarz, C. M., Ehrich, S., and Peinke, J.: Wind turbine load dynamics in the context of turbulence intermittency, Wind Energ. Sci., 4, 581–594, https://doi.org/10.5194/wes-4-581-2019, 2019. a, b
Sim, S., Peinke, J., and Maass, P.: Signatures of geostrophic turbulence in power spectra and third-order structure function of offshore wind speed fluctuations, Sci. Rep., 13, 13411, https://doi.org/10.1038/s41598-023-40450-9, 2023. a
Sørensen, J. D. and Toft, H. S.: Probabilistic Design of Wind Turbines, Energies, 3, 241–257, https://doi.org/10.3390/en3020241, 2010. a
Sreenivasan, K. R., Prabhu, A., and Narasimha, R.: Zero-crossings in turbulent signals, J. Fluid Mech., 137, 251–272, https://doi.org/10.1017/S0022112083002396, 1983. a
Veers, P., Dykes, K., Lantz, E., Barth, S., Bottasso, C. L., Carlson, O., Clifton, A., Green, J., Green, P., Holttinen, H., Laird, D., Lehtomäki, V., Lundquist, J. K., Manwell, J., Marquis, M., Meneveau, C., Moriarty, P., Munduate, X., Muskulus, M., Naughton, J., Pao, L., Paquette, J., Peinke, J., Robertson, A., Sanz Rodrigo, J., Sempreviva, A. M., Smith, J. C., Tuohy, A., and Wiser, R.: Grand challenges in the science of wind energy, Science, 366, eaau2027, https://doi.org/10.1126/science.aau2027, 2019. a
Virkar, Y. and Clauset, A.: Power-Law distributions in binned empirical data, Ann. Appl. Stat., 8, 89–119, 2014. a, b
Wettermast Hamburg: https://wettermast.uni-hamburg.de/frame.php?doc=Home.htm, last access: 29 January 2025). a
Yassin, K., Helms, A., Moreno, D., Kassem, H., Höning, L., and Lukassen, L. J.: Applying a random time mapping to Mann-modeled turbulence for the generation of intermittent wind fields, Wind Energ. Sci., 8, 1133–1152, https://doi.org/10.5194/wes-8-1133-2023, 2023. a, b
Young, G. S. and Kristensen, L.: Surface-layer gusts for aircraft operation, Bound.-Lay. Meteorol., 59, 231–242, https://doi.org/10.1007/BF00119814, 1992. a
- Abstract
- Introduction
- Methodology and data
- Statistics of Tc for atmospheric turbulent data
- Comparison to pure turbulent and synthetic wind data
- Conclusions and outlook
- Appendix A: CWS periods vs. persistence events
- Appendix B: Power law distributions
- Appendix C: Statistical moments of power laws
- Appendix D: Estimation of bmin
- Appendix E: Further details of experimental wind-tunnel and synthetic IEC standard wind data
- Appendix F: CTRW model for the generation of wind fields
- Appendix G: Spatial coherence of Tc
- Code availability
- Data availability
- Author contributions
- Competing interests
- Disclaimer
- Acknowledgements
- Financial support
- Review statement
- References
- Abstract
- Introduction
- Methodology and data
- Statistics of Tc for atmospheric turbulent data
- Comparison to pure turbulent and synthetic wind data
- Conclusions and outlook
- Appendix A: CWS periods vs. persistence events
- Appendix B: Power law distributions
- Appendix C: Statistical moments of power laws
- Appendix D: Estimation of bmin
- Appendix E: Further details of experimental wind-tunnel and synthetic IEC standard wind data
- Appendix F: CTRW model for the generation of wind fields
- Appendix G: Spatial coherence of Tc
- Code availability
- Data availability
- Author contributions
- Competing interests
- Disclaimer
- Acknowledgements
- Financial support
- Review statement
- References