the Creative Commons Attribution 4.0 License.
the Creative Commons Attribution 4.0 License.
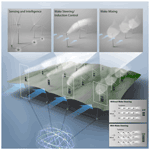
Wind farm flow control: prospects and challenges
Carlo Bottasso
Katherine Dykes
Paul Fleming
Pieter Gebraad
Gregor Giebel
Tuhfe Göçmen
Jan-Willem van Wingerden
Wind farm control has been a topic of research for more than two decades. It has been identified as a core component of grand challenges in wind energy science to support accelerated wind energy deployment and to transition to a clean and sustainable energy system for the 21st century. The prospect of collective control of wind turbines in an array, to increase energy extraction, reduce structural loads, improve the balance of systems, reduce operation and maintenance costs, etc. has inspired many researchers over the years to propose innovative ideas and solutions. However, practical demonstration and commercialization of some of the more advanced concepts has been limited by a wide range of challenges, which include the complex physics of turbulent flows in wind farms and the atmosphere, uncertainties related to predicting structural load and failure statistics, and the highly multi-disciplinary nature of the overall design optimization problem, among others. In the current work, we aim at providing a comprehensive overview of the state of the art and outstanding challenges, thus identifying the key research areas that could further enable commercial uptake and success of wind farm control solutions. To this end, we have structured the discussion on challenges and opportunities into four main areas: (1) insight in control flow physics, (2) algorithms and AI, (3) validation and industry implementation, and (4) integrating control with system design (co-design).
Wind farms today consist of tens to hundreds of multi-megawatt turbines working together to provide low-cost energy to electricity grids across the world. Operating for multiple decades, these machines constantly interact with turbulent flows from the atmosphere, influenced by local orography and further affected by the wakes of upstream turbines. To ensure reliable and low-cost operation and the best possible performance in energy production, control solutions are critical to optimize the turbine power production while balancing structural loading through the turbine components. Advancements in wind turbine control solutions, collective and individual pitch control, yaw control, and more have enabled higher energy capture while, at the same time, allowing for lighter weight and lower cost machines (Bossanyi, 2003b).
However, the operation of wind turbines that are clustered together in a farm is coupled through the flow. Thus, research and development on control for wind energy applications has increasingly shifted to the farm level – where the overall performance, reliability, and cost of the fleet of turbines is considered. Addressing complexities and uncertainties in the physics of wind farm phenomena – from the flow to the machine dynamics to the interaction with the grid – has proved challenging and has ultimately limited widespread commercial adoption of more advanced wind farm control solutions. Due to this, wind farm control has been identified as a key component of one of the three grand challenges in wind energy science (Veers et al., 2019) that must be resolved to unleash the full potential of wind energy in our future clean and sustainable global energy system.
Core to the challenge of wind farm control are wakes. Wake effects have long been recognized as influencing the efficiency of wind turbine clusters, reducing the total energy capture compared to turbines that operate in isolation. With the development of large wind farms in the 1980s in California, early work looked into the formulation of wake models in wind farms for use in the optimization of layout (Lissaman, 1979; Vermeulen and Builtjes, 1981; Jensen, 1983). The first attempt to control wake effects – using axial induction control – was proposed by Steinbuch et al. (1988) with the aim of increasing energy capture. Since then, many studies have looked into wind farm flow control (WFFC), aiming not only for improved energy extraction but also for reduced structural loads, improved balance of the power grid, and combinations thereof (see, e.g., Spruce, 1993; Sørensen et al., 2005, and many more since then).
The focus of this manuscript is on wind farm flow control. We define it as the coordinated control of the turbines in the farm, with the aim of influencing the flow (wakes, turbulence) in such a way that it improves the overall figure of merit of the farm. The latter can be, e.g., overall power extraction, total lifetime, levelized cost of energy, or simply the lifetime profit. A graphical impression of WFFC is provided in Fig. 1, highlighting in particular some of the physics that can be leveraged for influencing the flow (see also Sect. 1.2 for more details). Note that, in the literature, the term wind farm or wind power plant control includes WFFC but is often more broadly used. For instance, early work on wind plant control focused on grid stability under increased penetration of wind energy, using collective set-point strategies to balance the power grid, lumping all turbines together into one power plant model (Schlueter et al., 1983; Javid et al., 1985; Sørensen et al., 2002; Kristoffersen and Christiansen, 2003). Similarly, the term supervisory control, while sometimes encompassing WFFC, also refers to safe operation, start up and shut down, etc., for which flow interaction effects are usually not important. Next to this, the term wind farm control is sometimes also used in the context of the control of active and reactive power in the local wind farm power grid (Hansen et al., 2002). We refer the reader to these and similar works for broader discussions on wind farm control.
Finally, we note that some earlier reviews and perspective articles on wind farm control already exist. For instance, Kheirabadi and Nagamune (2019) provides a comprehensive review on increasing energy extraction with control. Later, Andersson et al. (2021) and Shapiro et al. (2022) extend the analysis to include the provision of grid services objectives, presenting a review of the corresponding studies. Houck (2022) provides a review of the studies per investigated flow control strategy with respect to power maximization, structural load alleviation, and ancillary services objectives. A recent work of Eguinoa et al. (2021) discusses WFFC in the context of electricity markets and grid integration, providing an initial overview of the capabilities and prospects of the technology from a large-scale systems perspective. Earlier reviews can, e.g., be found in Johnson and Thomas (2009), Knudsen et al. (2015), and Boersma et al. (2017). In this work, we extend past review efforts by focusing more broadly on outstanding research challenges in wind farm control in terms of (1) insight in control flow physics, (2) algorithms and AI, (3) validation and industry implementation, and (4) integrating control with system design (co-design).
In the next subsections, we first briefly review the main control objectives that are relevant for WFFC (Sect. 1.1) and the main control approaches (Sect. 1.2) before we further detail the scope of the current manuscript in Sect. 1.3.
1.1 Main control objectives studied to date
Very often, the main target of WFFC is to improve the levelized cost of energy (LCoE). This corresponds to the sum of all expenses (capital, operation and maintenance (O & M), and end-of-life costs) discounted to a fixed point in time, divided by the power production along the lifetime of the wind power plant (Riva et al., 2019). Beyond improving LCoE and other profitability objectives, wind farm control objectives may include service provision to the electric grid and/or mitigation of adverse social and/or environmental impacts. We briefly review the different control objectives that are commonly considered relevant for wind farm control.
Increasing energy extraction. A recent expert elicitation (van Wingerden et al., 2020) involving academic and industrial participants reports that a clear majority of the wind farm (flow) control community considers increased energy production to be the most important benefit of the technology. Its potential value is directly quantifiable for a gain in annual energy production (AEP) and increased revenue through producing more power at a given electricity price. Accordingly, it is the most studied objective of the wind farm flow controllers, with several examples of multi-fidelity model implementations, wind tunnel experiments, and field tests reported in the literature. Recent reviews with detailed quantitative comparisons can be found in Andersson et al. (2021) and in Kheirabadi and Nagamune (2019). The level of predicted gains varies substantially based on the specifics of a particular case, including the turbine technology, site and resource conditions, and more. Moreover, the confidence in the predicted gains in energy capture depends critically on the physical complexity of the wind farm flow represented in the models used as well as on the accuracy of the measurement sensors and the degree of sophistication of the considered data analysis methods.
Reducing structural loading conditions on the turbine(s). The higher levels of turbulence in the wake and the effects of asymmetric inflows from partially waked conditions result in additional structural loading on wake-impinged turbines. This additional structural loading can affect various components, such as the blades, hub, tower, bearings, transmission, and various actuation systems. Via the reduction of local wake-added turbulence and/or wake redirection, structural loading on the wind turbines can be mitigated through farm flow control. Compared to the increased energy capture objective, fewer studies investigate the dependencies of structural loading of wind turbines with regard to wake control concepts (Kanev et al., 2018; Vali et al., 2019b, 2022). Overall, the potential for structural load mitigation may be quite significant, and confidence in the predictions may be less sensitive to model fidelity and uncertainty (see, e.g., Campagnolo et al., 2020). However, the capitalization of structural load mitigation is harder to assess than changes in the power production, since the connection among structural loading, component reliability, remaining component lifetime, and ultimately O & M costs is challenging to assess (Clark et al., 2022; Réthoré et al., 2014). Still, some clear benefits may be realized both in the pre-construction and project development phases in terms of wind farm layout and balance of systems costs as well as during the operational phase (see Sect. 5 for a more detailed discussion). Furthermore, the business case for installing structural load-reducing farm flow concepts may also be of interest for the realization of lifetime extensions of existing turbines and farms.
Power tracking for ancillary services and balancing market participation. As stated earlier, to support the grid stability, a simple approach is to lump the farm together into one power plant model and to use this to optimize the grid support. Simple set-point distribution schemes, which distribute the farm set-point to the different turbines in the farm, are also commonly available (see, e.g., Kristoffersen and Christiansen, 2003; Hansen et al., 2006; van Wingerden et al., 2017). However, for the ancillary services that involve regulation of the active wind farm power output over time spans that surpass the turbine-to-turbine flow time, the ability to control wake interactions may lead to improvements in the way these services are provided. For example, when a reduction in total power output is demanded by the transmission system operators (TSOs), WFFC can be implemented to maximize the (potential) energy extraction and to mitigate structural loading while sustaining the aggregated power level within the quality of the TSO requirements (power tracking) (Ela et al., 2014; Shapiro et al., 2017a; Boersma et al., 2019). Accordingly, flow control can be implemented to maximize the reserve power for higher compensation during mandatory down-regulation (see, e.g., Siniscalchi-Minna et al., 2019) as well as for higher value in the balancing market and/or to minimize fatigue loads at all the selected turbines (see, e.g., Vali et al., 2019b). Similarly, flow control can support better asset management under flexible and dynamic electricity prices (Kölle et al., 2020; Eguinoa et al., 2021). In the zero-subsidy era, maximizing the revenue is likely to be prioritized over AEP gains, i.e., reducing the active power during times of low electricity prices (optimization of structural loads for a potential increase in lifetime instead), up-regulation (boosting), and/or active power maximization during higher electricity prices, maximization of the reserve power for the higher prices in the reserve market, etc.
Other O & M improvements. WFFC concepts can additionally be applied for protection of the power system as well as of several turbine components. The former is typically delivered as other types of ancillary services, such as reactive power and voltage control, and is addressed in the broader definition of wind farm control (Hansen et al., 2006). The latter can be in the form of protection against leading edge erosion, icing, overheating of power electronics, etc. In order to mitigate leading edge erosion, typically driven by extreme or aggregated rain events, turbine curtailment via reduction of tip speed is the state-of-the art approach (Bech et al., 2018). Similarly, active pitching is considered to be one of the mitigation techniques for icing on the blades (Sundén and Wu, 2015). For power electronics, avoiding overheating of components can be addressed through induction control (Ma et al., 2019). While these types of control are focused on the turbine and its components, and while turbine–turbine interactions through the flow may not play an important role, these objectives may nevertheless be a relevant contributor to an overall multi-objective wind farm control optimization that includes flow coupling. For example, (leading edge) erosion-safe mode via reduced tip speed at the upstream turbines can be combined with the objective of structural load mitigation at the downstream turbines, e.g., through axial induction wake control strategy, potentially adding to the relative profit increases recently reported by Hasager et al. (2020).
Mitigation of environmental and/or societal impacts. Variations in the rotational speed and pitch are commonly adopted as aerodynamic noise reduction techniques at wind farms (see, e.g., Jianu et al., 2012). Additionally, restricted turbine operation, typically in the form of curtailment or turbine shutdown on demand, is used as a mitigation technique for bird and bat collisions or for wildlife fatalities in general (Marques et al., 2014). Again, mitigating control actions are related to turbine control rather than to wind farm control. However, inclusion in a multi-objective framework, either as part of the objective function or as a constraint, may also lead to interesting interactions with the modes of operation of WFFC. This can further incentivize the implementation of the technology at certain sites and support the transition towards fully integrated WFFC.
1.2 Control approaches
We briefly review current WFFC approaches in terms of their physical actuation of the wake. The most common mechanisms considered to date include axial induction control and yaw control, and these are the main focus of the current work. However, tilt control is also of interest – particularly for downwind and floating offshore wind turbines – and will be briefly discussed. In the future, additional actuators in turbine systems may provide other forms of control, e.g., the use of blade flaps (Barlas and van Kuik, 2007; van Wingerden et al., 2008), but these are excluded from the current discussion.
In reality, wind farm control relies on actuation at the turbine level. This includes control of the torque set-point of the generator, the blade pitch angle, and the turbine yaw setting. However, when discussing wind farm control, very often, collective effects on the flow physics that result from turbine actuation are directly considered as a control input without considering the precise actuation at the turbine level. The most common example is induction control, in which the axial induction set-point of the turbine is changed to affect the wake and its downstream interactions. This may be achieved in various ways, i.e., by changing the generator torque set-point (thus changing the tip-speed ratio), the collective blade pitch angles, or combinations thereof. We should note that, although these details do not matter much for the effective wake flow development, they do matter in terms of loads and power and should be included in the overall control optimization. For instance, derating the turbine without pitching the blades is suboptimal in terms of power extraction, i.e., given a thrust set-point, there is a unique pitch to tip-speed ratio combination that maximizes power. When considering yaw control, changes in the yaw set-point can lead to changes in the thrust set-point as well, which need to be properly captured for correct wake behavior. Again, these changes can include changes in generator torque or blade pitch set-points, and precise details can matter a lot for the effective power output and turbine structural loads. Finally, we note that, given a selected thrust and yaw set-point, the effective turbine torque (and related power set-point) will have a subtle effect on the amount of wake rotation induced by the turbine, but these effects are small, given that modern turbines operate at high tip-speed ratios.
Before discussing wind farm control strategies in more detail, we distinguish between two main categories, i.e., quasi-static WFFC and dynamic WFFC. The former approach changes turbine set-points at a relatively slow pace, adapting them to background meteorological variations (changes in wind direction and/or wind speed over the day) but does not react to physical details that happen at time scales that are significantly faster than the farm flow through time. Dynamic wind farm control aims at including faster flow physics, e.g., accounting for wind gusts traveling through the farm or for changes of turbine set-points traveling through wakes. More advanced dynamic approaches aim at directly influencing the wake mixing and turbulence. We briefly discuss six combinations of strategies that have received a lot of attention in the literature: static induction control, static yaw control, static yaw and induction control, dynamic induction control, dynamic yaw (and induction) control, and dynamic individual pitch control. In addition, a short treatment of tilt control (from both a static and dynamic perspective) is introduced. As previously discussed, increased energy extraction has been the control objective most commonly investigated so far, but other objectives around structural loading, reliability, and more have also been considered.
Static induction control is the earliest control strategy proposed for wind farm control (Steinbuch et al., 1988). The main idea is to down-rate upstream turbines, reducing their axial induction set-point and wake strength in the hope of increasing energy extraction of waked turbines. Individual turbine induction set-points can then be optimized at farm level as a function of wind direction, wind speed, etc. and can be used in an open-loop scheme to control the farm. However, although initial results based on simplified wake models (e.g., Corten and Schaak, 2003; Schepers and van der Pijl, 2007; Horvat et al., 2012; Tian et al., 2014) were very promising, in recent years, it was convincingly shown that potential gains in energy extraction are smaller than originally predicted (Gebraad et al., 2015; Bartl and Sætran, 2016; Bartl et al., 2017; Annoni et al., 2016b; van der Hoek et al., 2019). Among other results, wind tunnel experiments conducted with small-scale turbines in a neutral boundary layer have shown that, while down-rating does energize the wake, it also results in a slower recovery, thereby achieving only modest power gains downstream (Bottasso and Campagnolo, 2020). Similarly, small gains observed in a recent full-scale field experiment Bossanyi and Ruisi (2021) remain within statistical uncertainty. Nevertheless, recently, there has been some renewed interest in static induction control, e.g., in tightly spaced farms – see Sect. 2.1.1 for further discussion.
Static yaw control exploits the fact that wind turbine wakes are redirected when the turbine is misaligned with the incoming wind direction (Clayton and Filby, 1982; Atkinson and Wilson, 1986a), which is a simple result from Newton's action–reaction principle. An illustration of the effect observed in large-eddy simulation (LES) is shown in Fig. 2 (middle panel). Atkinson and Wilson (1986b) and Parkin et al. (2001) were among the first to suggest using this mechanism to influence operational conditions (energy extraction, loads) in downstream turbines. Since then, this approach has received significant attention, with strong potential for energy gains observed in simulations (Jiménez et al., 2010; Gebraad et al., 2016), wind tunnels – which provided the first experimental evidence of this method in Campagnolo et al. (2016a) and later in Bastankhah and Porté-Agel (2019) and Campagnolo et al. (2020) – and in the field (Fleming et al., 2017, 2019; Howland et al., 2019; Ahmad et al., 2019; Fleming et al., 2020; Doekemeijer et al., 2021; Simley et al., 2021). Overall, similar to static induction control, wind turbine yaw set-points in a farm can be optimized offline as a function of wind direction, speed, etc. and applied in an open-loop control scheme to the farm. The first commercial products that employ yaw control are currently on the market (Siemens Gamesa Renewable Energy, 2019). Nevertheless, a number of challenges remain, as discussed in Sect. 2.1.2.
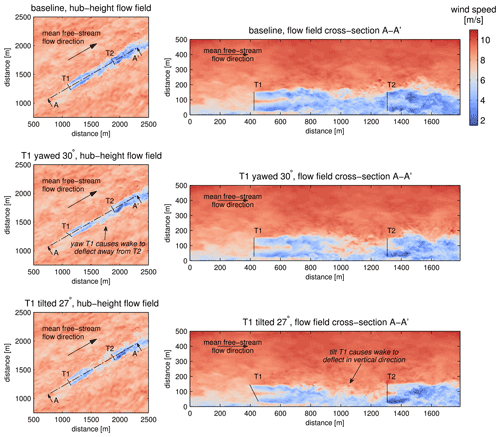
Figure 2Large-eddy simulation comparing yaw and tilt control versus a baseline setup. Figure from Fleming et al. (2015). Reproduced with permission.
Static yaw and induction control can potentially be combined. Yawing a turbine to redirect its wake increases the turbine structural loading. Combining yaw control with derating (under-induction) of the turbine can be used to find a trade-off between energy extraction and structural load reduction at the level of the farm (Bossanyi, 2018; Debusscher et al., 2022). Another track that has received some attention is the combination of yaw control and over-induction. In a detailed LES-based optimization study of wind farm controls, Munters and Meyers (2018a) noted that energy extraction by (static) yaw control can be further enhanced by combining it with over-inductive induction control (under high thrust coefficient). More recently, the idea was investigated more thoroughly by Cossu (2021b), who noted that stronger induction enhances wake deflection while also reducing the losses in the upstream yawed turbine.
Dynamic induction control. In dynamic induction control, turbine thrust set-points are changed much faster, with the aim of potentially increasing energy extraction over the static situation. Goit and Meyers (2015) and Goit et al. (2016) were the first to investigate this approach in an LES-based optimization setting using a receding horizon control approach; they found theoretical gains that can be quite high. However, the complexity of LES-based receding horizon control remains too large for practical application. A more practical approach, based on simple sinusoidal variations of the thrust set-point, was proposed by Munters and Meyers (2018c) and was shown to increase wake mixing, thus increasing the energy extraction at downstream turbines. Later, Frederik et al. (2020b) provided a first proof of concept in a wind tunnel setting, validating the potential of dynamic induction control. Nevertheless, many issues need further investigation (see Sect. 2.2). Finally, we should mention that some studies, using LES, have also focused on power tracking, dynamically changing individual turbine induction set-points (Shapiro et al., 2017a, 2018b). In this case, the dynamic aspect was not so much related to trying to influence turbulent mixing but rather to dynamically responding to changing wake characteristics due to turbulent wind gusts and changing turbine set-points that travel through the wakes.
Dynamic yaw (and induction) control. Studies on dynamic yaw (possibly in combination with dynamic induction) are more scarce. Munters and Meyers (2018a) used LES-based optimization to study the potential of various combinations of yaw and induction control. They found that sinusoidal dynamic yaw control can be used to trigger wake meandering but that the approach is less effective than static yaw control and further loses its effectiveness with higher turbulence intensities. They further found when combining dynamic yaw and induction control that most of the effectiveness comes from the baseline static yaw and over-induction set-points and that the additional dynamic response to the turbulent background field only brings smaller improvements. Howland et al. (2020), also using LES, reported similar conclusions for a neutral atmospheric boundary layer. However, results may depend, to a large extent, on wind farm layout and spacing (Munters and Meyers, 2018b).
Dynamic individual pitch control. Cyclic individual pitch control has been used to control turbine structural loads by reducing the 1P and 3P blade-passing excitation frequencies (Bossanyi, 2003a). The use of cyclic pitch for WFFC was first explored by Fleming et al. (2014) to redirect the wake in lieu of yawing or tilting the rotor (an approach that could technically be categorized as “static” wake redirection) and was then experimentally tested in a wind tunnel by Campagnolo et al. (2016b). More recently, in an LES study, Frederik et al. (2020a) used a modulation version of cyclic pitch control to actuate helical wake modes that improve mixing, and thus power extraction, at downstream turbines. The method has the advantage of not increasing structural loading as much as dynamic induction control. See more in Sect. 2.2.
Tilt control can be used to provide vertical steering of wakes (see also Fig. 2, bottom panel). While most turbines do not include actuators or degrees of freedom that would enable vertical wake steering, such steering may also be achieved for floating turbines using differential ballast control (Nanos et al., 2020). Simulation studies (Cossu, 2020, 2021a) and experimental studies with scaled turbines (Scott et al., 2020; Nanos et al., 2020; Bossuyt et al., 2021) indicate that deflecting the wake towards the ground results in larger power boosting than when the wake is deflected upward towards the sky.
1.3 Outline
The rest of the paper is organized around four major areas with important fundamental challenges but also significant potential for improvement. In Sect. 2, we review state of the art and knowledge gaps from the wind farm control perspective in the physics of turbulence, wakes, and the atmospheric boundary layer as well as what physics can be influenced. Then, in Sect. 3, we explore opportunities for improving algorithms, including state estimation and AI or machine learning. Section 4 addresses outstanding needs in experimental validation and demonstration at different scales from simulation to field experiments. Finally, in Sect. 5, we look at opportunities to integrate wind farm control in the broader context of wind turbines and farm design (i.e., co-optimization or co-design of control strategies with the physical system).
In the current section we first discuss challenges and opportunities with respect to quasi-steady flow physics in Sect. 2.1. Subsequently, we discuss aspects of wake dynamics and turbulence in Sect. 2.2. Finally, in Sect. 2.3, the relevance of the control of mesoscale phenomena, such as wind farm blockage, are discussed.
2.1 Quasi-steady flow control physics
Static wake control concepts rely on affecting the wake through relatively slow changes of the control degrees of freedom of the turbine in a way that affect the time-averaged properties of the wake. Over the years, this type of control has been extensively studied, so the response of the wake to control actions is relatively well documented. Nevertheless, open questions remain when considering, e.g., near-wake behavior, impact of atmospheric conditions, effects of wake shape, and deficit on structural loads, as further discussed in Sect. 2.1.1 for steady axial induction control and in Sect. 2.1.2 for steady yaw control.
2.1.1 Axial-induction-based control
In the past, most research on axial induction has been on increasing energy extraction. However, more recently, several studies have demonstrated the potential for increased energy extraction from static induction control to be rather low (see discussion in Sect. 1.2). Gebraad et al. (2015) showed that the possible kinetic energy gains in the wake resulting from under-induction are mainly concentrated at the outer part of the wake. Since the wake expands as it flows downstream, this kinetic energy may not be captured by a turbine standing downstream. However, overall gains in power production may still be attainable in situations with partial wake overlap. There are also some indications that under-induction may lead to increased energy extraction in tightly spaced wind farms (spacing less than 4 rotor diameters), where the effect of reducing the initial velocity deficit in the near wake is dominant (van der Hoek et al., 2019). Also full-scale experiments by Duc et al. (2019) show indications that gains may be more significant in a tightly spaced pair of wind turbines in fully waked conditions. Furthermore, recent efforts have shown some opportunities for increasing power production depending on the implementation of the control strategy (Pedersen and Larsen, 2020). Nevertheless, demonstrating in field experiments effective gains that are statistically significant remains challenging (Bossanyi and Ruisi, 2021).
The use of over-induction, in contrast to the under-induction that is usually considered in axial induction control, is a relatively new research area. In below-rated regimes, it is possible to reduce the power set-point of a turbine while increasing the turbine thrust set-point. This can be achieved by increasing the tip-speed ratio while optionally also adapting the pitch angle (Goit and Meyers, 2015). Such an operational regime is normally never considered for down-rating, as it increases turbine structural loading. Over-induction was first considered in a dynamic induction control context by Goit and Meyers (2015) (see below). Munters and Meyers (2016) found that, when reducing the response time of dynamic control towards a static approach, significant power increases may still be realized using over-induction, whereas this is not the case for under-induction. Martínez-Tossas et al. (2022) investigated the wake behavior under over-induction through LES, showing that it leads to faster wake breakup as the turbine starts to behave more as a bluff body, and they derived an empirical model to capture this enhanced recovery mechanism. Additional research is needed in this area, among others, showing the benefit of this mechanism for wake mitigation and investigating the effects of increased structural loading associated with the higher thrust set-points.
Finally, it is well documented that, in turbine arrays, multiple set-point combinations that yield approximately the same energy output exist (see, e.g., the wind tunnel experiments of Bartl and Sætran (2016); Bartl et al. (2017)). This can be leveraged to minimize overall turbine structural loads while providing power output according to a schedule for the full farm. Recent research in this area can be found in Vali et al. (2019b), Baros and Annaswamy (2019), Galinos et al. (2020), and Stock et al. (2020). In order to successfully apply these concepts, further research and possible test campaigns are needed to validate wind farm structural load models in relation to the effect that axial-induction-based wind farm control concepts have on the structural loads on specific components of the downstream turbines. This direction of research may be very relevant for LCoE reductions of wind farms in the future, particularly if included as part of an overall co-optimization framework – see Sect. 5 for further discussion.
2.1.2 Wake steering using yaw offsets
At present, wake steering through yaw is probably the most advanced control approach in terms of commercial realization (see, e.g., Siemens Gamesa Renewable Energy, 2019). Nevertheless, a number of research challenges remain in this area, mostly related to the complex response of the wake to yaw set-points and its strong dependence on atmospheric conditions.
First of all, when the wake is deflected using a yaw offset, it gradually deforms into a curled shape when moving downstream, deforming into a kidney-like cross section (Howland et al., 2016; Bastankhah and Porté-Agel, 2016; Bartl et al., 2018; Fleming et al., 2018). As a result of this and the natural wake rotation induced by the turbine torque, the wake deflection is also not symmetric with respect to the yaw offset (Bastankhah and Porté-Agel, 2016; Bartl et al., 2018; Fleming et al., 2018). However, it is well known that the wake shape can depend strongly on atmospheric conditions. In particular, stratification (Magnusson and Smedman, 1994; Chamorro and Porté-Agel, 2010) and wind veer (Abkar and Porté-Agel, 2016; Bromm et al., 2017) are known to have significant effects on wake behavior. The effect of these parameters in the presence of yaw is much less studied to date. Also, turbine control can have an effect on the wake of a yawed turbine: e.g., the effect of load-reducing individual pitch control on the behavior of the wake of a wake-steering wind turbine was investigated using LES in Wang et al. (2020a).
Secondly, the structure of a deflected wake includes the presence of a “transverse wake” (Atkinson and Wilson, 1985, 1986a), i.e., a lateral flow component (or side-wash) that results in the lateral displacement of the streamwise velocity deficit. In turbines that are sufficiently close downstream of a yawed turbine, this effect (called “secondary steering”) generates a change in inflow direction, as first observed in LES simulations by Fleming et al. (2018); Wang et al. (2018) and also confirmed by wind tunnel experiments in the latter reference. Similarly to above, this phenomenon is expected to depend on atmospheric conditions such as stratification and wind veer.
In recent years, significant research has focused on developing wake models that include wake steering, changing wake shape, and possibly also secondary steering (see, e.g., Bastankhah and Porté-Agel, 2016; Shapiro et al., 2018a; Martínez-Tossas et al., 2019; Howland and Dabiri, 2021). Additional parameterization of atmospheric conditions and testing in actual control settings are still largely ongoing research topics.
Finally, in terms of loading, static yaw control has mixed implications that need to be carefully considered. Yaw control can be used to reduce loading in downstream turbines by slightly yawing the upstream turbine to reduce partial waking of downstream machines. At the same time, however, yaw control that improves energy production may increase partial waking and structural loading of downstream machines (Herges et al., 2018). Furthermore, the yawed turbine may also see some increase in structural loading under certain operational conditions (Damiani et al., 2018). Thus, the application of static yaw control in practice seeks to balance or avoid negative structural loading impacts while still achieving benefits in increased power and energy production (Fleming et al., 2019).
2.2 Dynamic flow control physics
Here, we focus on the challenges related to triggering and interacting dynamically with wakes and turbulence as a means to control wind farm flow and to speed up wake breakup or to increase mixing and entrainment into the wakes. Recently, some first studies suggest that this effect may be leveraged to significantly increase the energy extraction of wind farms (Goit and Meyers, 2015; Munters and Meyers, 2018c; Frederik et al., 2020a).
2.2.1 Wake dynamics
From a phenomenological point of view, two different physical mechanisms that lead to wake breakup have received significant attention: tip- and/or root-vortex instabilities and wake meandering. Next to this, vortex rings triggered by the dynamic modulation of turbine thrust (Munters and Meyers, 2018c) have also received some attention recently. We briefly discuss these topics here, highlighting possible connections to control and discussing limitations and challenges.
Tip-vortex and root-vortex instabilities have been investigated as an explanation for wake breakup and mixing (Sørensen, 2011; Iungo et al., 2013; Viola et al., 2014; Quaranta et al., 2015). These studies mostly looked at linear stability analysis of single wakes without effectively studying wind farm control. Nevertheless, e.g., Quaranta et al. (2015) suggest that carefully selected modulation of the blade rotation leads to faster wake breakup triggered by tip-vortex instability. However, all of these studies are at low turbulence intensities, for which the wake remains very stable, and dynamics are dominated by the helicoidal tip-vortex system. At higher turbulence intensities, the tip-vortex system is dissipated relatively quickly, and other nonlinear effects play a dominant role in wake mixing.
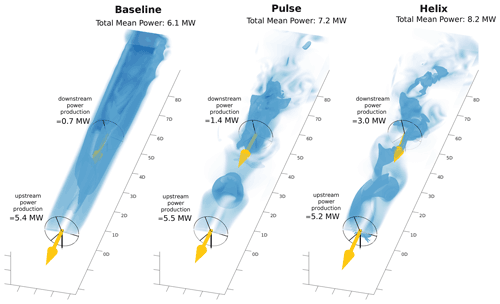
Figure 3Illustration from an LES study of a stable, normal-operation wake (left panel), wake resulting from (periodic) dynamic induction control (Munters and Meyers, 2018c) (middle panel), and wake resulting from the helix approach (Frederik et al., 2020a) (right panel) along downstream distances normalized in turbine diameter (D). Dark blue shading corresponds to an isosurface of the velocity; light blue shading in the horizontal plane corresponds to velocity magnitude. For further details on simulation setup, etc., see Frederik et al. (2020a).
A second phenomenon that has been studied extensively is wake meandering (Taylor et al., 1985; Medici and Alfredsson, 2008; Larsen et al., 2008; España et al., 2011; Kang et al., 2014; Foti et al., 2018). This type of instability is mostly triggered by large-scale motions in the background turbulence and leads to increased dynamic loading on downstream turbines. Munters and Meyers (2018a) investigated sinusoidally varying yaw control for increased mixing, observing the triggering of wake meandering. However, the effectiveness of this approach decreased for higher turbulence intensities, as wake meandering in this case is already triggered (and saturated) by ambient turbulence. Moreover, at low turbulence intensity, static yaw control yielded better results for the cases studied by Munters and Meyers (2018a), and, although wake meandering will improve power production, the increased loading may be more of a disadvantage. Nevertheless, whenever wake meandering is present, it is quite relevant to include it in control models so that turbines properly respond to time-varying inflow conditions caused by meandering. To date, wind farm control studies that explicitly include wake meandering in their models remain scarce (Yang et al., 2015; Munters and Meyers, 2018a; Doekemeijer et al., 2020b).
Munters and Meyers (2018c) proposed a sinusoidally varying thrust coefficient to induce additional mixing in the wake. This type of actuation leads to the excitation of a train of large annular vortex rings surrounding the wake that entrain high-speed fluid into the core of the wake (Munters and Meyers, 2018c; Yılmaz and Meyers, 2018) (see also Fig. 3). While Munters and Meyers (2018c) used a simulation setup based on an actuator disk model, Yılmaz and Meyers (2018) investigated the same type of control using an actuator line model, using a combination of pitch and torque control. Later, dynamic induction control was also tested in a wind tunnel by Frederik et al. (2020b) and by Brown et al. (2021), while a combined actuator line simulation and experimental study addressing both power and structural loading is reported by Wang et al. (2020c). However, Munters and Meyers (2018c) did not find evidence that sinusoidal dynamic induction control remains effective when used for anything other than first-row turbines or when used in situations with high turbulence intensities (for which wake mixing is already very high). When considering a large farm or large turbulence intensities, to date, no simple dynamic schemes that can lead to increased energy extraction were identified (see also Sect. 2.2.2 below).
Recently, Frederik et al. (2020a) proposed a different type of dynamic control that is based on a modulation of classical cyclic pitch control to trigger helicoidal breakup of the wake. A visualization of the typical breakup pattern is shown in Fig. 3. The major advantage of this approach over collective dynamic induction control is that actuation amplitudes are much smaller and that added turbine structural loading is much lower, while wake breakup may even be slightly better. However, to date, this work has remained limited to inflow with low turbulence intensity and a few turbines only. Extension to more complex inflow conditions and large farms is still under investigation.
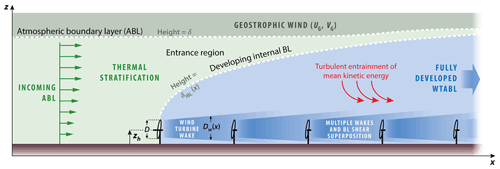
Figure 4Illustration of the interaction between the atmospheric boundary layer and large wind farms. From Stevens and Meneveau (2017). Reproduced with permission.
The field of dynamic control to trigger faster wake breakup is still very young. Theoretical connections to the field of linear stability analysis may be of interest but have not been fully explored. Next to that, methods have only been investigated for rather simple inflow conditions and small turbine arrays. The effects of stratification, wind shear, veer, etc. have not yet been studied.
Finally, apart from representing a possible excitation mechanism for active control, wake dynamics may also play an important role in the breakup of wakes when externally forced. For instance, Abraham and Hong (2020) show that dynamic wake modulation by wind gusts or direction changes plays an important role in wake recovery. As another example, offshore-floating wind turbines and their movement (heave, surge, roll, and platform yaw and pitch) may be another cause of external excitation of the wake (Wise and Bachynski, 2020) that will gain relevance in the future. Incorporating these effects into improved wake models for control (e.g., in the context of ancillary service power tracking) is another interesting avenue of future research.
2.2.2 Boundary-layer turbulence
The atmospheric boundary layer is the lower part of the atmosphere that is directly influenced by drag and convective heat exchange with the surface. Its height can vary from tens of meters to several kilometers, depending on atmospheric conditions, stratification, surface roughness, etc. Depending on the stratification regime, the boundary layer can be very turbulent (unstable conditions) or nearly laminar and intermittent (very stable conditions). Also, the size of coherent turbulent motions in the boundary layer can change significantly, with a cascade of scales ranging from kilometers down to millimeters in unstable and neutral conditions, whereas the largest coherent scales in stable conditions tend to be much smaller. For a classical review of the most important aspects that play a role, we refer to Stull (1988) and Garratt (1994).
Given their size, large wind farms interact in complex ways with the atmospheric boundary layer. The size of modern turbines places them in the middle of the turbulent spectrum (for neutral and unstable conditions), which is important for turbine structural loading but also for power variability and interactions of atmospheric turbulence with wakes. The wind farm will also lead to the development of an internal boundary layer, akin to a classical roughness change. An illustration of the important interactions that play a role is shown in Fig. 4. We refer to Stevens and Meneveau (2017) and Porté-Agel et al. (2020) for recent reviews on the topic of wind farm–boundary-layer interaction.
From a control perspective, the interactions between wind farms and the atmospheric boundary layer may lead to interesting opportunities. The wind turbines can be seen as flow actuators and can be potentially controlled to influence wake mixing, entrainment of high-momentum flow into the farm, etc., possibly leading to increased energy extraction or reduced structural loading. To date, research in this area is scarce and has been limited to neutral atmospheric conditions only. Goit and Meyers (2015) were the first to consider wind farm–boundary-layer interaction in an optimal control setting governed by large-eddy simulations. Later, Goit et al. (2016) and Munters and Meyers (2017, 2018a, b) followed up on this work, all focusing on increasing energy extraction in the wind farm using dynamic induction control. However, from a control implementation point of view, this approach is hindered by the large cost associated with using large-eddy simulations as a control model. Moreover, strong simplifying assumptions – i.e., perfect knowledge of the state – and a control model that exactly matches the (virtual) plant were used (Munters and Meyers, 2017). Unfortunately, physical insight into the dynamic controls that can be distilled from this framework into simple and robust control laws remains elusive. Munters and Meyers (2018c) performed a detailed analysis of LES-based optimal control to try and identify such laws, uncovering sinusoidal induction control as a mechanism to increase wake mixing (see Sect. 2.2). However, this mechanism only works for first-row turbines and explains only a fraction of the energy gains observed in the full LES-based dynamic controls. Whether the remaining part of the gain is related to the control of turbulence and entrainment in the atmospheric boundary layer or rather to the physics of wake mixing in more downstream turbines remains unknown.
Without insights into the control physics, devising a simple controller that leverages wind farm–boundary-layer interactions may not be feasible. An alternative would be to build a real-time controller that directly uses turbulence-resolving simulations, such as large-eddy simulations, as a control model. Whether it is feasible to arrive at a controller that can be used in real time will depend on the minimum resolution (Bauweraerts and Meyers, 2019) or possible model simplifications (Boersma et al., 2018) that still represent the relevant physics while making the control model sufficiently fast. Additional challenges are related to the correct estimation of the full three-dimensional turbulent state (Bauweraerts and Meyers, 2021) and associated uncertainties (see also Sect. 3). With further advances in computer architecture and flow sensors, such a brute-force optimal control approach may become feasible in the long term but will most probably remain out of reach for the next decade.
2.3 Mesoscale effects, blockage, and wind farm wakes
With wind farms growing in size, effects that extend beyond the scale of the atmospheric boundary layer are becoming visible. Two effects that have received significant attention are wind farm wakes and blockage. We hypothesize that wind farms may interact in other ways with mesoscale systems, such as land–sea breeze systems, convective cells, etc. However, to date, these interactions have not been studied in much detail. Given that wind farms can influence the system on this scale, there may also be opportunities for control by either mitigating effects (e.g., power loss) or by incorporating mesoscale coupling in a more general optimal control setting.
Wind farm blockage was first observed in field experiments by Bleeg et al. (2018), and they reported possible wind speed reductions between 2 % and 4 % because of wind flowing around the farm (instead of through it). Schneemann et al. (2021) performed lidar measurements of the Global Tech I offshore wind farm in Germany, showing wind speed reductions of up to 6 %. Furthermore, extensive work has studied blockage in simulations and in wind tunnel experiments, with research following two main working hypotheses for the root cause of blockage. Currently, various new full-scale experiments are underway to further identify wind farm blockage (see, e.g., Moriarty et al., 2020; RWE Renewables, 2021).
To date, the working hypothesis that has received most attention relates wind farm blockage to classical hydrodynamic blockage. Segalini and Dahlberg (2020) performed detailed wind tunnel experiments in neutral conditions, showing blockage effects in the order of 2 % to 3 %. Earlier wind tunnel studies have shown similar effects for a row of wind turbines, while in side-by-side arrangements, so-called in-field blockage may even increase energy extraction (McTavish et al., 2014). Several simulation studies have also investigated hydrodynamic blockage, including Meyer Forsting et al. (2017), Wu and Porté-Agel (2017), and Bleeg et al. (2018). When simplifying the flow physics to potential flow and a single axisymmetric open rotor, we arrive at the Betz limit as a direct expression of hydrodynamic blockage. From this perspective, hydrodynamic blockage in wind farms would simply be a result of the difference between the expected Betz optimal point for each turbine and the effective power extraction in a wind farm setup that is not anymore axisymmetric (i.e., when jointly considering all rotor surfaces). From a controls or optimization perspective, including these additional physics may lead to different operational set-points (and/or designs) when turbines are clustered together. However, while the effect of turbulent mixing of the wake can be neglected in the Betz theory, it will play a significant role in wind farms, typically reducing wake deficits, and therefore also influencing blockage. In lidar experiments, Schneemann et al. (2021) observed that the effects of blockage are fully absent in unstable (highly turbulent) atmospheric conditions but are prominently present in stable atmospheric conditions. Better understanding these effects and including them in control and design models will be an important step to improving wind farm set-point optimization.
A second working hypothesis that has been suggested as an important root cause for blockage is the excitation of atmospheric gravity waves by wind farms. It is well understood that gravity waves are excited in the stably stratified free atmosphere by orography, such as mountains or hills (see, e.g., Teixeira, 2014, for a review). Smith (2010) was the first to suggest that large wind farms may also excite gravity waves, with a potential impact on power production because of pressure feedback in the wind farm boundary layer. Allaerts and Meyers (2017) and Allaerts and Meyers (2018) performed large-eddy simulations of neutral and stable boundary layers, also showing that gravity waves may reduce wind speeds upstream of the farm, with the effects in stable boundary layers being significantly larger. Allaerts and Meyers (2019) proposed a fast engineering model to incorporate the effect of gravity waves in wind farm wake models, partly inspired by a simpler model proposed by Smith (2010). Using this model, Lanzilao and Meyers (2021) performed a set-point optimization study that tried to mitigate energy losses, finding possible power gains of 4 % to 14 %, depending on atmospheric conditions. To date, experimental data that confirm the existence of gravity waves excited by wind farms are lacking, and additional research is necessary. Unfortunately, the effects of gravity waves are very difficult to reproduce in wind tunnels, so more full-scale data are needed. Finally, we note that blockage may be a combination of classical hydrodynamic blockage and wave effects, depending on atmospheric conditions. A first attempt to disentangle both effects in simulations was recently discussed by Centurelli et al. (2021).
Apart from blockage, wind farm wakes have also received significant attention in recent years. First observations using satellite imaging already date back to Christiansen and Hasager (2005) for the Nysted and Horns Rev I farm, showing that farm wakes can persist over long distances in stable atmospheric conditions. Since then, several other studies have looked into wind farm wakes (Nygaard and Hansen, 2016; Nygaard and Newcombe, 2018; Platis et al., 2018; Cañadillas et al., 2020), with wakes extending more than 50 km in some cases (Cañadillas et al., 2020). Compared to turbine wakes, wind farm wakes decay much slower. Whether it is possible to improve vertical mixing or to redirect farm wakes by control has not yet been established but may lead to interesting new research challenges. Whether such mitigation strategies would be economically viable is another open question, particularly since interacting farms are not necessarily owned by the same company.
Finally, we remark that many more mesoscale effects that influence wind farm operation exist: weather fronts, thunderstorms, coastal gradients, gravity waves originating from orography, etc. However, many of these mesoscale systems are too large to be directly affected by wind farms. In these situations, wind farm control boils down to optimally responding to larger-scale phenomena, e.g., when they are advected through the farm, while keeping track of flow-feedback effects at smaller scales, such as turbine wakes. When considering optimal control, an important challenge in this context is the identification of these large-scale systems, as they are not straightforwardly accessible through local measurements within the wind farm.
Understanding the control physics that can potentially be leveraged for WFFC (see Sect. 2) does not suffice to arrive at an effective and robust wind farm controller. At the core of WFFC, we have the operational logic and algorithms that make the decision regarding how to operate the individual turbines based on the actual conditions to maximize a certain objective (e.g., power maximization, structural load distribution, or the provision of ancillary services). There are many ways to categorize the algorithms, but in general, we can talk about open-loop control and closed-loop control. We start this section by explaining these different concepts and by giving an overview of the state of the art. Later, in Sect. 3.3, we indicate the synergies with the field of machine learning and AI.
3.1 Current practice – open-loop control
The current baseline for WFFC is defined by many recently performed field experiments in which induction and wake steering are tested (Fleming et al., 2017; Ahmad et al., 2019; Howland et al., 2019; Duc et al., 2019; Fleming et al., 2020; Doekemeijer et al., 2021; Bossanyi and Ruisi, 2021; Simley et al., 2021). In all these experiments, the control algorithm is based on offline-calibrated, steady-state engineering models. These models are optimized offline for different atmospheric conditions, and look-up tables (LUTs), which are used online in combination with external inputs, are generated (Doekemeijer et al., 2021). These external inputs – such as mean wind direction, wind speed, and possibly other atmospheric conditions such as turbulence intensity, and stability – are fed into the LUT to determine the optimal control settings. In traditional control terminology, this architecture can be labeled as “open-loop” control, and the performance strongly depends on the accuracy of the model and is prone to disturbances. The information flows as demonstrated in Fig. 5 are followed within the “open-loop” control scheme.
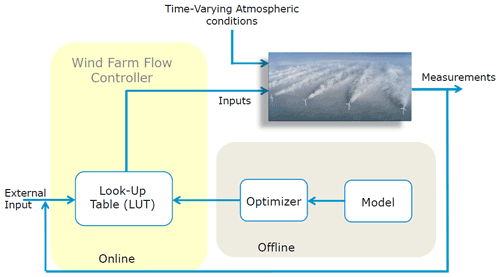
Figure 5Open-loop control scheme: current practice in wind farm flow control (Horns Rev-I wake picture courtesy: Vattenfall. Photographer is Christian Steiness).
Although this open-loop approach has been successful in controlled testing environments to date, it requires human check-ins of small test sites to confirm correct operation (Bossanyi and Ruisi, 2021; Fleming et al., 2020; Howland et al., 2019). To apply WFFC at the scale of whole wind farms and higher temporal resolutions and to better manage uncertainties and model errors, robust feedback control is an important feature which can directly accommodate the majority of the inherent uncertainties in wind energy production.
3.2 The closed-loop paradigm
For wind farm control, the feedback information flows are demonstrated in Fig. 6. In this feedback control setting, measurements are used in a real-time optimization framework to determine the next control policy. This framework can deal with, among other factors, model uncertainty and unknown disturbances. For example, in Doekemeijer et al. (2019), the benefits and challenges for closed-loop control are discussed using the engineering model FLORIS. However, the level of complexity and detail embedded in closed-loop control can differ significantly, e.g., steady-state-model-based closed-loop control (acting on quasi-static wake behavior; see Sect. 2.1), quasi-dynamic-model-based closed-loop control (including wake delays and responding to mesoscale variability in the flow field; see Sect. 2.3), and fully dynamic closed-loop control (predictive control with fully dynamic feedback to the turbulence and updated states; see Sect. 2.2). It is evident that the external input, measurement, communication, and computational requirements necessary to execute these different types of feedback control can vary greatly (see also Sect. 3.4).
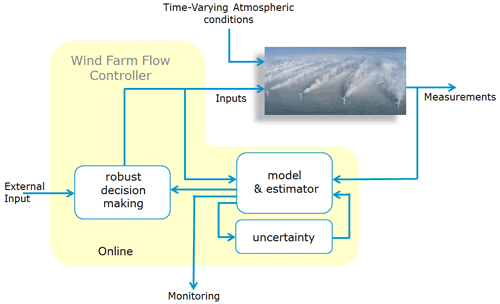
Figure 6Closed-loop control scheme: envisioned framework for future wind farm flow control (Horns Rev-I wake picture courtesy: Vattenfall. Photographer is Christian Steiness).
In academia, little attention has been paid to the practicality of implementing such closed-loop control schemes, as focus has been on proving the essential concepts, e.g., maximum power point tracking (Gebraad and van Wingerden, 2015; Gebraad et al., 2013), game theory (Marden et al., 2013; Park et al., 2013), extremum seeking control (Johnson and Fritsch, 2012; Creaby et al., 2009; Ciri et al., 2017), and dynamic programming (Rotea, 2014; Guo et al., 2020). This is especially evident, as few of the efforts consider how to handle sensor uncertainties, actuator uncertainties, and modeling errors and instead focus on maximizing utility under near-perfect conditions. In the foreseen framework, however, real-time data will be used continuously to update a dynamic wind farm model, and in combination with an uncertainty description, it will be used to make cautious (with a safety margin) or robust decisions about the control settings of the individual turbines in a receding-horizon framework. Through that framework, the turbine's wake can be controlled, minimizing its impact on neighboring wind turbines for realistic conditions. At the core of this model control paradigm is the fusion of prior knowledge and measurement data (data assimilation), resulting in data-driven calibrated models that can be used for real-time decision making. These data-driven calibrated models or digital twins can also be used for monitoring wind farms. In the remainder of this section, we will elaborate on the different building blocks of the closed-loop control paradigm.
Internal model. The internal model contains the essential first principles to extrapolate and predict the future behavior of the wind farm as a function of the possible control actions. There are many steady-state models proposed that can serve as an internal model, such as Jensen (Jensen, 1983), Frandsen (Frandsen et al., 2006), Ainslie (Ainslie, 1988), FUGA (Ott and Nielsen, 2014), or Porté-Agel and Bastankah's Gaussian wake formulation (Bastankhah and Porté-Agel, 2016). The main difference among these various methods lies in the characterization of the steady-state wake and the wake control effects they include. Several of these models have recently been assembled in open-source wake model libraries such as FLORIS (National Renewable Energy Laboratory, 2021) or PyWake (DTU Wind Energy, 2021). Also, in recent years, many dynamic engineering models with the capability to predict the dynamic behavior of the wakes as function of a time-varying control input(s) have been proposed. For example, WFSIM (Boersma et al., 2018, 2016; Torres et al., 2011) uses a two-dimensional computational fluid dynamics (CFD) model to predict the dynamic behavior of the different wakes as a function of the time-varying yaw actions. There are many other (quasi-)dynamic models, with different fidelities (representing the flow, possibly the turbines, etc.) that can take a similar role, such as FLORIdyn (Becker et al., 2022), FRED (Van Den Broek and van Wingerden, 2020), PossPOW (Göçmen et al., 2019), FastFarm (Jonkman and Shaler, 2021), HAWC2Farm (Liew et al., 2022), and the DWM model by Larsen et al. (2008). Another modeling approach uses data from field measurements and/or high-fidelity CFD to generate low-dimensional surrogate models. This approach, also referred to as data-driven modeling, uses techniques such as dynamic mode decomposition (DMD) to generate models (e.g., Liew et al., 2022; Chen et al., 2020; Annoni et al., 2016a; Cassamo and van Wingerden, 2020) and/or machine learning approaches (see also Sect. 3.3). While dynamic or static first-principles models easily generalize for different wind directions and speeds, these data-driven models face several computational challenges, since they need to be trained on representative data. Schreiber et al. (2020a) proposed a hybrid approach that tries to circumvent the limitations of purely model-based and purely data-driven methods by augmenting a baseline engineering model with correction terms that are learned from data. Depending on the application, main challenges regarding the internal model include accuracy, computational load, programming language, included and represented physics, disturbance modeling, and fidelity.
State estimation or data-assimilation, model calibration, model adaptation. WFFC depends on the awareness of the current situation of the flow field within the wind farm, the state of the turbines, and the ability to predict based on the current situation or state. In this estimation step, all the available measurement data are combined with an internal model to estimate the current situation or state within the wind farm. This is classically called state estimation or data assimilation and was recently also referred to as situation awareness. With an updated state, the data-augmented model, or digital twin, can be used to predict the future. In control engineering, several types of state estimation techniques – such as the family of Kalman Filters (Doekemeijer et al., 2018; Shapiro et al., 2017b; Doekemeijer et al., 2016, 2017) and direct model inversion techniques (Schreiber et al., 2020a; van Der Hoek et al., 2021) – are commonly implemented. Before the state-estimation step (as also shown in Doekemeijer et al., 2019), a model calibration step is made – see e.g., Schreiber et al. (2017) and Doekemeijer et al. (2020a) for the FLORIS model and Sørensen and Nielsen (2006) for the Jensen model. More recently, model adaptation techniques (Andersson et al., 2020; Schreiber et al., 2020a) have been proposed to update the first-principles model using data which clearly tie in with the field of machine learning and AI. Besides using these data-augmented models for decision making, they can also be used to provide important information for monitoring purposes, since indirectly measured states (also labeled as virtual sensors) can also be reconstructed. When choosing an estimation strategy, the main challenges can be listed as follows: model and/or desired output accuracy, sampling time, sensors required, type of model knowledge required, and convergence speed. Depending on the time scales, it is expected that different solutions will be optimal.
Robust decision making. The (mixed) objective function (e.g., power maximization, structural load minimization, or power reference tracking) conditioned by the data-calibrated internal model will be used to make decisions regarding how actuators are employed over time. In general, the concept of receding-horizon control is used. Based on the current state of the wind farm, the control variables are optimized over a control horizon. The first computed control action will be implemented, and at the next time step, a new optimization problem is solved. The optimization problem can be solved with many different algorithms, such as gradient descent and adjoint methods (Vali et al., 2019a, 2017). The important challenges in such decision-making processes are reliability, adaptability, robustness, the ability of the algorithms to work with uncertainty, the convergence and convexity of the optimization problem, and the overall computational complexity.
In summary, given its building blocks, the overall challenge for the closed-loop paradigm is to provide the theoretical foundations, robust integrated designs, and novel optimization routines that will enable the next generation of wind farm flow estimators and controllers. This envisioned framework is at the crossroads of systems and control engineering, optimization, and machine learning and has strong synergies with artificial intelligence.
3.3 Synergies with artificial intelligence and other digitalization concepts
With the increased availability and accessibility of the data, the implementation of artificial intelligence (AI) algorithms has been continuously expanding in the field of wind energy. This is particularly the case for machine learning, which is a subset of AI that includes complex statistical techniques (including multilayered neural networks, also referred to as deep learning) to enable machines or models to improve at tasks with experience. Machine learning has been increasingly applied to WFFC workflows over the last few years. Currently, the main focus is improving the models and estimators (see Fig. 6) to reduce uncertainties in the predictions, typically without an explicit representation of the external input uncertainties. This data-informed modeling can include model adaptations via active learning from operational data to correct physical model inadequacies (Schreiber et al., 2020a; Andersson and Imsland, 2020) or can be purely data driven via, e.g., surrogate modeling (Hulsman et al., 2020).
A central challenge for the machine learning applications within WFFC today is related to the fundamental limitations of the applied algorithms, mainly due to their dependencies on data and computational resources. The first problem is represented by the insufficient data in terms of its size (e.g., lack of available data), its rate of growth (e.g., delays in data streams, malfunctioning data storage), its variety (e.g., data is accessible only for limited turbine types), and overall uncertainties associated with it. Additionally, observability of all the necessary features that are to be included to predict all the relevant states of the wind farm flow is restrained by the capabilities of the sensors and/or availability of the signals and channels. Additionally, complex high-dimensional WFFC problems with many features require even more observations to achieve statistical significance and to avoid data sparsity and overfitting (generally referred to as the “curse of dimensionality”; Bellman, 1961).
With broader use of machine learning, the cost of hosting and processing the data and the training, updating, and inference of the models is growing rapidly. High performance computing (HPC) plays an increasingly important role in addressing such demands, enabling their implementation in WFFC to grow significantly. Examples of such growth could be extensive surrogate modeling based on high-fidelity turbine and flow representation in a wind farm environment (e.g., LES with fully resolved blades; Mittal et al., 2016), with high spatial and temporal resolutions and long(er) simulation times (Andersen et al., 2020). With all the control hierarchies included, such analyses would be an essential building block for comprehensive digital twins (Grieves, 2014) of the entire power system (e.g., of a region) to help better advise future planning and operation of renewables.
The knowledge gap in utilizing data-driven workflows (based on observations) for a WFFC framework is far greater than physics-based workflows (based on a set of rules or physical representation) in terms of the model development and logical sequence of decisions taken to reach a prediction for the stakeholders of the technology (Feng, 2019). Accordingly, another important challenge for implementing modern machine learning tools in WFFC is the complexity of the algorithms (Marugán et al., 2018). Typically, the models are employed (by domain scientists) in a black-box manner, where the accuracy is prioritized over interpretability or explainability. Although essential, validation in this perspective is not enough to ensure reliable operation of wind farm(s) as safety-critical systems under strict constraints. A probabilistic framework and the representation of uncertainty is as crucial for AI approaches as it is for physics-based modeling for WFFC, as recently shown in, e.g., Rott et al. (2018) and Quick et al. (2020). The transition from deterministic to probabilistic approaches for AI in wind energy started with Kalman filters (Bossanyi, 1985) and recently continued for deep learning with Bayesian neural networks (Liu et al., 2020; Mbuvha et al., 2021) and with mixture models (Vallejo and Chaer, 2020; Zhang et al., 2020) capturing the parameter and output uncertainties. Together with other probabilistic architectures, their application to flow control problems is a growing research interest.
It is also relevant to investigate which features are important in the model predictions and how they are combined (Díaz et al., 2020). Additionally, decision-level explanations can be provided by breaking down a single or global model into smaller sub-models and analyzing the information flow and series of decisions throughout (Chatterjee and Dethlefs, 2020, 2021). Such efforts are crucial, as the lack of transparency induces additional risks for large-scale deployment of AI approaches in WFFC. This prevents the transition from data-supported and data-driven workflows (humans as the central processor) to AI-driven workflows (AI as the central processor) for robust decision making. The advantage of AI-driven workflows, with AI at the center of the decision-making process, in a WFFC framework is their capability to process much higher levels of information much faster and to capture very detailed trends and variances. They are also capable of mapping very nonlinear relationships within the operation to define optima that could otherwise be hidden (see, e.g., Marden et al., 2013; Ahmad et al., 2016; Zhao et al., 2020). Overall, the AI transformation has great potential to increase the efficiency and reliability of the WFFC technology towards the new-generation, fully autonomous wind power plants that go beyond the “foreseeable” future. It remains to be seen to what extent such transformation will take place and if AI applications will replace the current model-based WFFC implementation entirely.
3.4 Controllability, observability, and sensors
When moving from the current open-loop paradigm to closed-loop WFFC, including the use of advanced data-assimilation methods, a number of additional theoretical and practical challenges arise related to the controllability and observability of the three-dimensional turbulent flow in the wind farm. Controllability refers to the extent to which flow states and outputs can be influenced by a limited set of control actuators, e.g., the turbine blades in WFFC. From a practical perspective, it is reasonably well documented how the turbine can influence the quasi-steady flow (see Sect. 2.1), but much less is known about dynamic control. Although theoretical controllability results exist for various types of systems (see, e.g., Kalman, 1963; Lin, 1974), these methods become intangible for high-dimensional nonlinear systems, such as wind farm flows.
Observability is the dual of controllability. It identifies how well flow states and inputs can be determined by a limited set of measurements and thus provides an upper bound for the level of flow awareness that can be attained. With more sophisticated control models and control dynamics, more sensors will be necessary to provide statistically relevant state and input estimations. In modern wind farms, sensors usually provide local or point measurements, either located at turbines (including virtual sensors for wind speed, shear, etc. – see below), at meteorological masts, or at the substation. To what extent they suffice as an input to more sophisticated dynamic control algorithms with feedback is still largely unexplored. Nowadays, various remote flow sensing devices (lidar, sodar, radar, drones) promise to improve flow awareness. However, both cost and robustness aspects should be considered when adding more complex sensors into the mix, which should be more than offset by control gains, essentially leading to an integrated cost optimization problem (see Sect. 5). Moreover, although extensive work has already been performed on flow reconstruction from, e.g., lidar measurements (see, e.g., Simley et al., 2011; Mikkelsen et al., 2013; Schlipf et al., 2013; Simley et al., 2014a, b; Lundquist et al., 2015; Raach et al., 2017), the more sophisticated three-dimensional reconstruction of turbulence still faces many challenges (see, e.g., Lin et al., 2001; Chai et al., 2004; Raach et al., 2014; Bauweraerts and Meyers, 2021).
When considering sensors for WFFC, a lot of them provide flow data directly. These include local sensors such as wind speed anemometers (cup, ultrasonic), air temperature measurements, wind vanes, and also remote techniques such as lidar, sodar, and radar. However, with advances in monitoring of the turbine itself, indirect flow measurements that use a model of the wind turbine to invert the structural responses to wind input are also possible. These include the measurement of rotor-equivalent wind speed (see, e.g., Soltani et al., 2013), the use of blade-root load sensors (strain gauges) to detect wakes (Bottasso et al., 2018; Bottasso and Schreiber, 2018) or, in general, to estimate sector-equivalent wind speeds and hence horizontal and vertical wind shears (Schreiber et al., 2020b), or the use of load harmonic components to estimate shears as well as inflow angles (Bertelè et al., 2021). Given the low cost and relative robustness of the sensors involved, the use of these indirect wind measurements (and their time series) for more advanced reconstruction of the flow field downstream of the rotor is an interesting avenue of future research.
Finally, as highlighted throughout this manuscript, WFFC is part of a multi-objective optimization problem that also includes turbine structural loading, cost of O & M, etc. (see also Sect. 5). Thus, for proper feedback control, the flow state should be identified, and the turbine state is essential. For instance, using load sensors, structural loading can be monitored at crucial spots in turbine components by using digital twin technology to generate a continuous evaluation of the accumulated fatigue damage (Pimenta et al., 2020). This type of turbine self-awareness requires a well-considered sensing strategy at the level of the turbine and its controller. A further discussion of these elements is, however, outside the scope of the current paper.
The final ambition of research in wind farm control is commercial implementation. However, next to a commercial cost–benefit analysis and various other aspects (for example, certification; see Manjock et al., 2020), it is necessary to develop a thorough validation and proof of concept of each new control idea and of its implementation. A recent expert elicitation on wind farm control identified validation as the key priority for advancing wind farm control (van Wingerden et al., 2020).
Several wind farm control concepts have been shown in the past few years to have the potential to increase wind farm production. Some concepts have made it to commercial application: for example, wake steering is now available as a commercial product (Siemens Gamesa Renewable Energy, 2019). However, the realization of such commercial opportunities is invariably supported by comprehensive research and development programs across many organizations and countries over several years.
A wind farm represents a high-value asset in which many parties are involved (one or more wind farm owners, a wind farm operator, an original equipment manufacturer, and possibly insurers, financiers, and certification bodies). One or more stakeholders also share in the project revenue generation as well as in the costs and risks. Thus, demonstration should not only address the potential of a new innovation (such as a WFFC strategy) but also the quantification of the risks of that innovation that are critical to industrial adoption. Such demonstrations must be grounded in well-designed simulation experiments and test campaigns that generate data and results that are realistic, transparent, and reliable.
In recent years, this has led towards a four-stage approach to developing confidence and trust in control concepts, ordered by increasing cost and effort: (1) proof of concept in a sufficiently advanced high-fidelity simulation environment, such as large-eddy simulations; (2) validation of the control concepts in a (boundary layer) wind tunnel; (3) field campaign on research turbines or older assets, with a limited scope (e.g., involving a small number of turbines); and (4) full-scale field campaign. Initially, a high-fidelity simulation or wind tunnel experiment is a key enabler for further R & D investment decisions and for further effort being put into testing at full scale. In recent years, the sequence of simulation to scaled testing to field demonstration has proven to be a model for the commercialization of WFFC solutions. However, each of these stages faces specific challenges, with significant room for improvement. These are further addressed in detail in Sect. 4.1–4.3 below. Finally, additional aspects with respect to full-scale industrial implementation are discussed in Sect. 4.4.
4.1 Proof-of-concept studies in high-fidelity simulation tools
In recent years, LES has emerged as a virtual environment capable of representing significant features of wind farm flows (see, e.g., Calaf et al., 2010; Porté-Agel et al., 2011; Churchfield et al., 2012; Wu and Porté-Agel, 2013; Fleming et al., 2014; Witha et al., 2014; Wang et al., 2017; Martïnez-Tossas et al., 2018), making it an ideal choice for the testing of wind farm control strategies (Goit and Meyers, 2015; Munters and Meyers, 2018c; Vali et al., 2019b; Wang et al., 2019; Doekemeijer et al., 2020b). The advantages of wind farm LES include a high degree of control over the simulation and the ability to run counter-factual simulations. For instance, running a case with and without controls while using the exact same inflow provides a very clear means to compare performance, something that is not possible in full-scale field experiments. Additionally, the full flow field can be readily accessed and analyzed in space and time, contrary to the experimental scaled and full-scale cases, where synchronous and high-resolution measurements of the complete field are not (yet) possible. High-fidelity CFD simulation tools have become more widely available and are used by an increasing number of research teams (for example, LES codes like SOWFA by NREL, SP-Wind by KU Leuven, EllipSys3D LES by DTU, PALM by Leibniz University Hannover, and TUM.LES by TU Munich, which has specialized in the CFD replication of wind tunnel experiments). Nevertheless, many challenges and opportunities remain.
First of all, LESs of wind farms are computationally expensive, usually requiring high-performance computing. Simplifying assumptions are often necessary to keep computational resources manageable. Although fully blade-resolved simulations of wind turbines are becoming feasible (see, e.g., Kirby et al., 2019; Sprague et al., 2020), these simulations are currently too expensive to perform over the sufficiently large spatial and temporal domains that are necessary for wind farm control testing. Therefore, a major simplification that is usually made is related to the turbine representation, which is either based on actuator disk (Sørensen et al., 1998; Jiménez et al., 2007), actuator line (Sørensen and Shen, 2002; Troldborg et al., 2007), or actuator sector models (Storey et al., 2015; Vitsas and Meyers, 2016). For wind farm control proof-of-concept studies, an actuator line or sector model coupled to an aeroelastic turbine representation and its controller is usually preferred, allowing the evaluation not only of power output but also of turbine structural loading. However, the accurate representation of some flow characteristics remains a challenge, including the correct representation of dynamic features related to the blade boundary layer (dynamic stall, rotational augmentation, three-dimensional effects) as well as near-wake features related to the tip-vortex system (Martínez-Tossas et al., 2017; Meyer Forsting et al., 2019).
Secondly, a representative parametrization of inflow conditions in LES remains an important area of research, with a large potential impact on the quality of the results. One aspect of particular importance is the generation of realistic inflow turbulence. In the past, research has focused on the development of synthetic turbulence approaches (see, e.g., Wu, 2017, for a review), but nowadays it is well established that precursor methods yield more realistic spatio-temporal correlations in the flow (Stevens et al., 2014; Munters et al., 2016). Another aspect that needs attention is that simulations are often performed using idealized background conditions, whereas the atmospheric inflow is governed by mesoscale weather systems that usually display a complex amalgamation of larger-scale flow features. In recent years, research has focused on coupling mesoscale models to microscale LES (Muñoz-Esparza et al., 2014; Muñoz-Esparza and Kosović, 2018; Haupt et al., 2020), but issues remain with respect to the characterization of representative turbulence at the LES inflow, which is not directly represented in mesoscale models.
Finally, although LES is quite a mature simulation technology that has been developed over more than three decades, some open problems remain with respect to subgrid-scale modeling, particularly in stably stratified conditions (see, e.g., Couvreux et al., 2020), and with respect to wall-stress models (required for the simulations of the atmospheric boundary layer) and the representation of complex terrains.
4.2 Validation in wind tunnel experiments
Wind tunnel testing with scaled models has long supported scientific research in various engineering fields, and wind energy is no exception. In addition to the classical characterization of the aerodynamic performance of airfoils (which, for wind energy applications, poses its own challenges due to the combination of high Reynolds numbers and unsteady inflows), wind tunnel testing has recently moved to the simulation of clusters of scaled wind turbine models exposed to flows that mimic the characteristics of the atmospheric boundary layer. Working hand in hand with simulations and full-scale experiments, wind tunnel testing contributes to the understanding of wake physics and the validation of wake models (Chamorro and Porté-Agel, 2009, 2010; Hu et al., 2012; Iungo et al., 2013; Viola et al., 2014; Bastankhah and Porté-Agel, 2015; Howard et al., 2015; Yang et al., 2016; Bastankhah and Porté-Agel, 2017b; Schreiber et al., 2017) and, in turn, to the development of wind farm control technology (Campagnolo et al., 2016a; Bastankhah and Porté-Agel, 2016; Wang et al., 2017; Campagnolo et al., 2020; Frederik et al., 2020b; Wang et al., 2020c). Although it is clear that any wind farm control method has to be demonstrated in the field before it can achieve full credibility, wind tunnel testing of control concepts has been gaining increasing popularity in recent years for proof-of-concept demonstration.
The success of wind tunnel testing is due to some of its distinguishing characteristics. First, differently from the field, the ambient conditions in a wind tunnel are precisely measurable, repeatable, and – at least to some extent – controllable. A number of boundary layer wind tunnels worldwide are capable of generating realistic scaled turbulent flows. Among these, the large facility at Politecnico di Milano, which features a 3.84 m (height) by 13.84 m (width) by 36 m (length) test section, has been used for some of the most complex wind farm control experiments to date (Campagnolo et al., 2016a; Bottasso and Campagnolo, 2020; Campagnolo et al., 2020; Frederik et al., 2020b; Wang et al., 2020c). In this facility, in a wake-steering experiment, dynamic changes in wind direction were simulated using the facility's large actuated turntable (Campagnolo et al., 2020) – see Fig. 7. Apart from size, which is important to limit blockage and to avoid excessive miniaturization of the models (Bottasso and Campagnolo, 2020), some facilities have been designed to generate specific flow conditions that are particularly relevant to wind energy applications. For example, the wind tunnels at the University of Surrey (Hancock et al., 2014) and at the University of Minnesota (Chamorro and Porté-Agel, 2010) can generate stratified flows, such that not only neutral but also stable and unstable boundary layers can be investigated; the WindLab wind tunnel (University of Oldenburg, DE) features a large active grid (Neuhaus et al., 2021), which gives more freedom in the generation of turbulent flows than the classical passive means (Zasso et al., 2005); the WindEEE facility (Western University, London, Ontario, CA) (WindEEE, 2020) can generate tornadoes and downbursts, which are relevant for the simulation of extreme operating conditions.
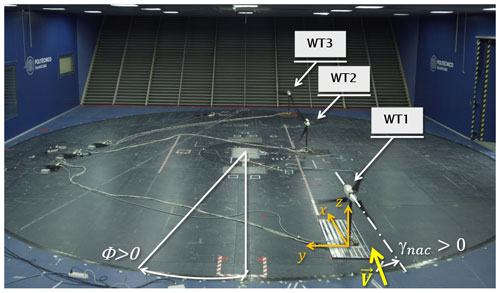
Figure 7Wind tunnel facility of the Politecnico di Milano, with a three-turbine setup for testing yaw control. Picture reproduced from Campagnolo et al. (2020). Reproduced with permission.
A second distinguishing feature of wind tunnel testing is that measuring in a laboratory environment is usually easier, more accurate, and cheaper than in the field. In fact, detailed characterizations of the inflow and of the wakes can be obtained with a variety of techniques and equipment that differ in cost, accuracy, spatial and temporal resolution, intrusiveness, and setup complexity. These include pressure and hot-wire probes (Lomas, 1986), particle image velocimetry (PIV) and its derived techniques (Adrian, 2005), and also – more recently – scanning lidars (van Dooren et al., 2017). Finally, when properly scaling a setup to smaller spatial dimensions while keeping velocity roughly at the same magnitude (as typically done for wind tunnel testing), the time scales will also decrease (time flows faster) (Bottasso and Campagnolo, 2020; Canet et al., 2021; Campagnolo et al., 2020) so that more statistical information can be accumulated over shorter experimentation times.
A third useful characteristic of scaled testing is that models can be designed ad hoc to achieve specific goals (Schottler et al., 2016; Lanfazame et al., 2016; Winslow et al., 2018; Kelley et al., 2016; Bastankhah and Porté-Agel, 2017a, b; Campagnolo et al., 2016a; Bottasso and Campagnolo, 2020; Nanos et al., 2022), and can be extensively instrumented (Bottasso and Campagnolo, 2020). Advances in 3D printing (Zhu, 2019), improvements in precision manufacturing and in the realization of complex composite structures (Campagnolo et al., 2014; Bottasso et al., 2014; Parandoush and Lin, 2017), advanced sensors (Qiu et al., 2020), miniaturized actuators, wireless transmission, and other technological improvements are being actively exploited to improve model building (Bottasso and Campagnolo, 2020). While the small dimensions often pose constraints, the flexibility offered by the design of scaled turbine models is hard to match when compared to full-scale machines. Sophisticated laboratory characterizations of crucial model components are also possible (Campagnolo et al., 2014; Wang et al., 2020b). Furthermore, farm layouts and scenarios can be readily changed to explore different conditions of interest by simply repositioning the scaled model turbines on the wind tunnel floor.
In parallel to these useful characteristics of wind tunnel testing, it is important to realize that, in general, a scaled model cannot exactly represent all physical processes that take place at full scale. Dimensional analysis, through the Buckingham Π theorem (Buckingham, 1914), lays the foundations for the systematic development of scaling laws for the design of model turbines. Depending on the application and focus of the research, scaled designs can differ significantly. For instance, in a wind tunnel of the same size, a focus on rotor aerodynamics may lead to a larger rotor design than when a study of wake behavior is the sole focus. Other aspects that can have a decisive impact on design are a possible focus on reproducing correct (scaled) aeroelastic behavior (Bottasso et al., 2014) or the inclusion of realistic turbine actuators. Moreover, the wind tunnel facility that is used will impose some additional constraints; thus, the design of a scaled model is an exercise in finding a best compromise, as is often the case in many complex engineering applications.
Canet et al. (2021) reviewed the laws that govern steady and transient gravo-aeroelastic scaling of wind turbine rotors, while Bottasso and Campagnolo (2020) applied the results of the scaling analysis to the design of wind turbine models for wind tunnel testing; Wang et al. (2021) looked specifically at the realism of the wakes generated by scaled models for wind farm control applications. The main findings of these studies show that, notwithstanding the existence of some mismatched effects mostly caused by the chord-based Reynolds number, with proper design choices, scaled wakes generated in wind tunnel experiments appear to be remarkably similar to their full-scale counterparts, except in the immediate proximity of the rotor.
A final aspect that is important when considering scaling is that a reduction of length scales leads to a reduction of time scales, i.e., an acceleration of time in the scaled experiment (Bottasso and Campagnolo, 2020; Canet et al., 2021; Campagnolo et al., 2020). Thus, control algorithms and actuations need to be executed much faster in a wind tunnel than at full scale. This poses constraints on the turbine hardware in terms of actuation rates and on the computing hardware and software in terms of execution speed (Campagnolo et al., 2020). Therefore, depending on the setup, the testing of complex control algorithms (e.g., model-predictive control) may not be feasible or may imply considerable challenges.
Notwithstanding the indisputable success of wind tunnel testing in recent years, much remains to be done on many fronts. For example, PIV and lidars should become more widely available, because accurate, non-intrusive flow measurements are indispensable for a better understanding of the flow physics as well as for model validation. The effects of floating motions on wake behavior and control are not well understood; however, the scaled testing of floating wind turbines is still in its infancy because of the challenge of replicating at scale (or by hardware-in-the-loop co-simulation) aerodynamic and hydrodynamic interactions. Improvements are also necessary to more faithfully replicate at scale the inflows that very large modern machines face in various types of atmospheric conditions together with the effects that terrain orography and vegetation cause on the flow and on wake behavior. The development of complete high-fidelity digital simulation-based replicas of experimental setups – including the wind tunnel, the turbine models, and the turbine- and farm-level control laws – can greatly increase the value of experimental measurements (Wang et al., 2019).
4.3 Validation via field tests
Full-scale validations implement wind farm controls on industrial wind turbines and seek to validate wind farm control models and performance. Some example studies at this scale include tests at the Summerview wind farm (Alberta, Canada) (Howland et al., 2019), the Peetz Table Wind Energy Center (Colorado, United States) (Fleming et al., 2019, 2020), the Sole du Moulin Vieux farm (Ablaincourt-Pressoir, France) (Ahmad et al., 2019), and the Sedini wind farm (Sedini, Italy) (Doekemeijer et al., 2021). The advantage of these tests are that they represent the realized concept in near-final form, with full-sized turbines in fully realistic atmospheric conditions. However, the challenge is the much-reduced controllability and observability of inflow conditions. Since identical conditions can not be guaranteed to be applied to the plant with and without wind farm controls, statistical methods must be used to try to quantify changes in performance. A common approach – for example, in Fleming et al. (2020) and Doekemeijer et al. (2021) – is to test the wind farm controller on only some of the turbines in the farm (putting all other turbines in a reference group) and then to toggle on and off the control group, producing a baseline and test dataset. By comparing the relative performance of the turbines being controlled to the reference turbines (through power ratio or energy ratio comparisons, for example) when the turbine is off versus on, the effect on power or energy production can be estimated. Toggle testing has been an established practice in testing new controllers for wind turbines (see Bossanyi et al., 2013). However, wind farm wake control strategies, unlike turbine control strategies, have a dependence on wind direction that complicates the comparison. Additionally, previous tests of turbine control strategies, based on pitch or torque control, could toggle quickly, making 10 min intervals a standard choice. However, in wake control, the yaw control changes much more slowly, and the changes in the wake need time to propagate downstream. These influences have made approximately 1 h toggling typical of early tests. The establishment of best practices in performing field validation is actively researched and is a focus point of the new IEA Wind Task 44: Wind Farm Flow Control (https://iea-wind.org/task44/, last access: 17 November 2022).
To date, there are many opportunities for advances in full-scale validation. First, collecting data in field campaigns using more turbines within farms, or even whole farms, and for longer periods than currently reported will provide much more certainty in terms of performance. Another opportunity is to employ more sophisticated measurements to learn about the fundamental properties and performance of flow control (for example, lidars, radars, and drones).
There are also opportunities for testing more sophisticated control models. To date, many of the wind farm controllers tested in public field trials of wake steering are based primarily on the open-loop paradigm and precomputed look-up tables. Future field tests should incorporate more recently developed control strategies, such as the consensus for collectively identifying wind directions (Annoni et al., 2019), or use online estimation (Doekemeijer et al., 2020b) or robust design (Rott et al., 2018). Furthermore, consideration of the underlying turbine yaw control optimized to implement wind farm control is an important area of ongoing work (Kanev, 2020).
Finally, there are many opportunities to measure and quantify impacts on structural loads in addition to confirming energy uplifts. While there is some research in the area of field trials of wind farm controls measuring impact on structural loads (Damiani et al., 2018), this is another critical area of ongoing research.
4.4 Industrial implementation
Once a wake control concept has been proven, several steps need to be taken in industry to achieve commercial application in operating wind farms. This is illustrated in Table 1, where we suggest definitions of technology readiness level (TRL) for wind farm control concepts, from the identification of basic principles (TRL 1) to commercial application (TRL 9). Challenges in transitioning from TRL 5 (first field test) to commercial implementation TRL 9 are further discussed in this section.
(Clayton and Filby, 1982)Atkinson and Wilson, 1986bMedici and Dahlberg, 2003Jiménez et al. (2010)Fleming et al. (2015)(Gebraad et al., 2016)(Campagnolo et al., 2016a)(Howland et al., 2019; Fleming et al., 2019)(Doekemeijer et al., 2021)(see e.g., Siemens Gamesa Renewable Energy, 2019)Table 1Definition of technology readiness levels (TRLs) for wind farm control concepts, and example references.
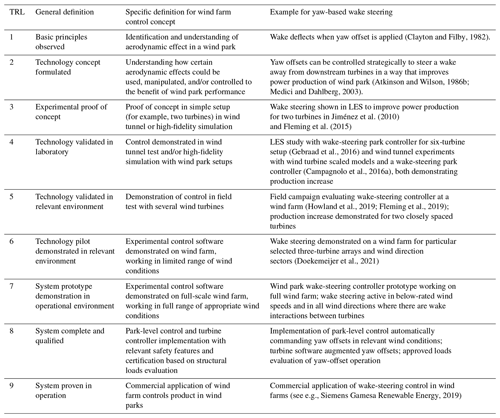
First, the effects of wake control need to be shown to be reproducible and measurable from sensor data that is readily available on a wind turbine rather than only seen in an experimental setup with special remote sensing equipment. This ensures that the performance of a wind farm control concept can be shown to work at several operating wind farms and that it is therefore scalable as a product. This step often requires adjustment of the available measurement equipment on commercial wind turbines. The adjustments may include calibration of the measurements for the alternative operational modes needed for the flow control, which is a requirement that could already be considered early on in the formulation of research questions and the setup of simulations or wind tunnel tests. Further, a typical practical issue to be solved is the accurate calibration of wind direction or nacelle yaw position relative to north at each site, which makes sure that wake control is done in the appropriate wind directions. Secondly, if a flow control mode is expected to increase certain structural loads acting on a wind turbine, an evaluation needs to be performed that shows whether the loads are still within the envelope that the turbine is designed for. Moreover, special care needs to be taken in the evaluation of actuators if they are used in a different way than originally designed for. For new wind turbine models, the design load cases could be adjusted to allow for more aggressive wind farm control strategies. This requires an integrated co-optimization of design and control. See Sect. 5 for further discussion. Thirdly, the park-level control and turbine controller needs to be implemented with the relevant safety features. This includes cyber-security measures allowing safe communication between the wind turbine and wind park-level control.
The three steps mentioned above allow for the transition from a field test (TRL 5) to the safe implementation of the park-level control on a wind park without special human supervision (TRL 8). For transitioning to TRL 9 (commercial application), not only does the control implementation need to be deployable on several sites in order to be able to scale the product, but the commercial value of the control also needs to be proven. If the objective of the wind farm control is to improve the wind farm AEP, this may entail validating model predictions using data from one or more farms and then using the model to project a longer-term benefit for specific sites. Alternatively, a more data-driven, site-specific approach can be used, where the AEP benefit is measured from a toggle test at a specific site in order to measure the performance of the wind farm control on that site. Such a toggle test may take several months to measure the park control benefit in a large range of wind conditions or even up to a year to take into account seasonal variations. An example approach for such a toggle test was presented in Boccolini et al. (2021). The wind industry further needs to establish relevant certifications and standards related to the above-mentioned topics of structural loads evaluation, safety, and wind park production benefit predictions and measurements. A position paper on certification practices for wind farm control was recently presented by Manjock et al. (2020).
It is also important to note that the advancement through the TRL stages also depends on specific technologies and site characteristics. Thus, a given control strategy may advance at different rates for different applications. For example, while wake steering is advanced to TRL 9 for land-based and fixed-bottom offshore wind farms, this is currently not the case for floating wind energy applications. In addition, on land, conditions related to complex terrain or other features of the wind farm design have yet to be fully validated from a yaw-based wake-steering perspective. The variation of performance and the risks of a control strategy for different technologies, site designs, and site environmental conditions require ongoing R & D well beyond the first instance of commercialization.
So far, industry has shown more interest in wind farm control concepts that result in AEP increase; however, in a subsidy-free market, more interest may be shown to other kinds of concepts that allow operators extra flexibility in optimizing revenue. A study of market showcases is discussed by Kölle et al. (2020) and Eguinoa et al. (2021). Furthermore, structural load reductions, providing grid services, and more may become of interest to industry. For any new control strategy application, it will be necessary to follow the R & D processes – from simulation to scaled testing to field demonstration – described in this section. Moreover, to accelerate the development of new wind farm control products, the industry could benefit from standardization of the processes and of the measurement and controls interfaces, such as the communications interface between WFFC and the turbine controller.
As with other technological systems, the up-front design and the resulting control strategies that are available are not independent. Improved WFFC can inform design processes and affect design heuristics. For instance, the ability to increase energy extraction and to reduce structural loads may enable the ability to build wind farms with increased power density (with higher AEPs per square kilometer).
Integrated design of the system hardware and software (or controls) is an emerging research area in wind energy, with the potential to enable significant innovation (Garcia-Sanz, 2019). Control co-design (CCD), which focuses on concurrent design of all relevant disciplines, including controls, has been recognized as a promising research and technology development pathway for wind turbines and, in particular, floating wind turbines (Garcia-Sanz, 2019). However, the community also recognizes the need to look at holistic design and control of full wind farms due to the significant couplings of the flow and performance at the wind farm level. Thus, there is a need to elevate the application of CCD to the farm level. Essentially, since the location and types of wind turbines, sensing equipment, and other control-related technologies in the farm determine the availability and magnitude of the wind farm control opportunity, there is a coupling of the up-front design of the farm and the downstream execution of wind farm control. First, we briefly discuss the state of the art in wind farm design before elaborating on recent efforts towards integrated wind farm design and control.
5.1 Progress in wind farm design optimization research
Research in pre-construction wind farm design spans multiple decades (Herbert-Acero et al., 2014). As discussed in Sect. 1.1, there are a number of objectives of interest for operation and control, including energy production and cost of energy. These same metrics are used in the pre-construction wind farm design process. Historically, AEP was the main objective, and cost elements have been increasingly incorporated to enable full LCoE (as a metric to represent a farm's overall economic profitability) (Ning et al., 2019).
As discussed in Dykes et al. (2021), the key ingredients of wind farm multidisciplinary design optimization correspond to the following:
-
Annual energy production. In addition to the size (e.g., rotor diameter) and performance features (e.g., rated power) of the turbines, AEP of a wind farm is also influenced by wake effects. With the objective of maximizing AEP, the turbine selection, the number of turbines, and the layout have all been considered in the optimization problem.
-
Capital expenditure for the balance of systems. CAPEX for BOS optimization is frequently considered as a key cost component within the sub-optimization problem of the electrical or power system (Perez-Moreno et al., 2018). Alternatively, its own detailed optimization is performed once a layout has been decided (Pérez-Rúa et al., 2020). Other costs for BOS, such as the roads for land-based farms, including the installation and logistics strategies, have also been explored.
-
CAPEX for turbines and foundations. Recent research has investigated the coupled optimization of wind farm and turbine design and selection (Stanley et al., 2018, 2019; Stanley and Ning, 2019; Graf et al., 2016) as well as coupling with the design of offshore support structures (Perez-Moreno et al., 2018).
-
Operational expenditure. Originally, site suitability for structural loads was considered after a farm layout was mostly fixed. More recently, surrogate models have allowed the inclusion of structural load models directly in the wind farm optimization (Riva et al., 2020).
For design, LCoE has served the wind energy community as a complex but straightforward metric, which allowed wind farm developers to largely ignore time-varying aspects of system performance and cost. Through statistical models, the time-varying nature of the wind resource was simplified into a wind rose, and the annual energy production for a given design was calculated once per design iteration.
Looking towards future electricity systems with large levels of variable sources of energy generation and a shift toward subsidy-free wind energy systems, the need to increase wind farm profitability will shift the focus from LCoE towards objectives that also account for time-varying revenues and costs. Initial work in this direction has shown that the current level of generation of wind energy in a system can be parameterized to give an indication of the value of new wind generation as a function of statistical correlations between wind and market conditions (Simpson et al., 2020). Other metrics account for the time-varying revenue a wind farm would see over its lifetime when participating in merchant markets (Beiter et al., 2021). Such metrics could also be used to evaluate the potential of control strategies as well as the co-design of the wind farm and control strategy together. This area of research is still largely unexplored but promises many new challenges for wind farm design, control, and the interaction of the two.
From a wind farm design perspective, there are various potential impacts of using value-based metrics over LCoE. We expect impacts on the following:
-
Wind turbine design. A recent trend has explored the potential of low-wind-speed machines or machines with low specific power that produce more electricity at lower wind speeds than conventional wind turbines (Simpson et al., 2020). Such machines can be designed to “cut out” at lower wind speeds so that they only operate at lower wind speeds (reducing their LCoE compared to machines that operate over a larger wind speed range) (Madsen et al., 2020).
-
Wind farm machine selection. Increasingly, wind farm designers can consider a spectrum of technology solutions as manufacturers move to platform models with a large number of variants, including rotor diameter, rated power, hub height, and other features. Wind farm designers can choose between these variants or some combination of them. With new low-wind turbines, as in Madsen et al. (2020), it could be possible to create a wind farm that includes both conventional and low-wind machines, with revenue under a broader range of resource and market conditions for higher overall system value.
-
Wind farm meta-design. A key design lever for value maximization is the ability to constrain the capacity of the wind farm relative to the sum of the capacity of individual assets. This is done by increasing the number of turbines relative to the rated output of the facility (a strategy known as over-planting) (Dykes et al., 2019). This can also be accomplished through hybridization with other generation technologies (such as solar photovoltaics) or by combination with storage technologies (Dykes et al., 2020).
-
Wind farm physical design. This addresses the overall physical layout or placement of machines and the BOS design.
Physical design is difficult to separate from both topics of machine selection and meta-design in the process due to significant couplings. The type and number of turbines, coupled with their placement in the farm, will influence the overall energy production of the farm. Also influencing the energy production is the overall operation of the plant and thus the control of the wind farm or hybrid power plant over its lifetime. Thus, holistic optimization of a wind farm necessarily brings together farm design and control. Furthermore, the introduction of control strategies into the wind farm design problem, as will be discussed, can impact not just the wind farm performance but also trade-offs in system performance and cost.
5.2 Wind farm control co-design (CCD)
As previously mentioned, CCD is a promising field for wind energy research in general due to the strong couplings between the physical system design and the design of the software control system (Garcia-Sanz, 2019).
5.2.1 Wind farm CCD for AEP and LCoE objectives
Some of the first works looking at the subject of wind farm applications focused on static yaw-based wake-steering control and wind farm layout optimization. In Fleming et al. (2016), combined wake-steering and layout optimization was shown to increase the energy production of a wind farm at the same time as the farm power density was increased (moving the turbines closer together and thus enabling cost reductions in the balance of systems). Gebraad et al. (2017) extended the work by considering a full wind rose of wind speed and direction to optimize the plant AEP. More recently, combined static induction control and layout optimization also demonstrated some potential for improving farm AEP (Pedersen and Larsen, 2020).
The above work did not address the structural loading conditions as influenced by either the turbine placement, control strategy, or both. Recent work by Riva et al. (2020) and Stanley et al. (2020) looked at the coupling of the layout and wind turbine structural loading under normal operation. A key challenge for research in wind farm design and control optimization applications considering turbine structural loading is the computational costs, as a single optimization iteration can become computationally intensive – even when parallelizing across operational cases. This becomes even more challenging when considering CCD of the control strategy and farm design together.
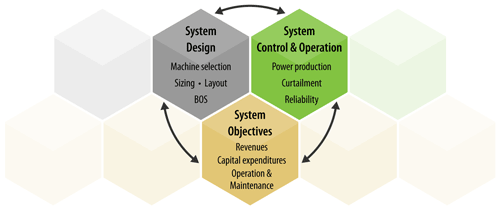
Figure 8Illustration of interdependencies for CCD considering system design, control strategies, and objectives.
The opportunities and challenges for CCD become larger as the operating degrees of freedom of the system increase. Thus, floating wind energy – which involves potential motions of the platform and turbine system in response to wind and wave conditions – creates a significant opportunity and challenge for CCD research (Garcia-Sanz, 2019). First, floating wind energy may be investigated similarly to fixed-bottom or land-based wind farms for CCD to benefit AEP, reduce LCoE, or address other objectives. However, floating wind energy may introduce additional design levers from the platform motion that might augment the benefits that can be realized from CCD (Barter et al., 2020). Furthermore, recent research has looked at the ability of using yaw-based control to displace floating wind turbines, thus not only inducing wake steering but also shifting the entire turbine and trajectory of the wake (Kheirabadi and Nagamune, 2020). To realize such a strategy, floating wind farm design would need to take into account these displacements in the broader optimization of not just the nominal layout of the project but also the topology and sizing of system components such as mooring systems, anchors, and the broader collection system.
5.2.2 Wind farm CCD for profitability objectives
Inherent in the entire discussion about wind farm design for value-based objectives beyond LCoE is an active control strategy that can no longer be treated as independent from the farm design itself. Similarly, the design and operation of wind farms for objectives beyond LCoE introduce further couplings of physical design and controls. By definition, over-planting means that some or many wind turbines in a wind farm will not operate or will operate at derated conditions, depending on the particular operational or control strategy. Similarly, hybridization of wind farms, including other generation assets, means that there are many objectives to balance: the overall farm revenue against the overall selection and sizing of the different assets, the physical park design, and the long-term reliability of the facility, in addition to minimizing the operational costs for the collection of assets.
In an LCoE-driven world, the long-term reliability of the assets is important. But, with discount factors and tax incentives favoring production in the early years of the project life cycle, designers and operators have focused largely on producing maximum energy at the lowest possible cost. Introducing value-based objectives where there is an explicit trade-off in the performance and reliability across the generation assets in a project necessitates a new approach to optimization that addresses the operation and control strategy upfront in the design process. Figure 8 illustrates this trade-off in a simplistic way. In short, the design of the system affects the operation and control strategies available, which in turn affect the overall project profitability (balancing revenues against costs). By taking into account the control strategy in the design process, through CCD, we can improve the life cycle profitability of the project. For wind energy systems with significant couplings between individual assets, due to wakes and their impacts on both downstream machine power production and structural loading, CCD will be necessary for realizing profitable wind farms in future, highly variable energy systems.
5.2.3 Wind farm CCD for non-economic objectives
As already discussed, the mitigation of environmental and/or social impacts can be an important objective of wind farm control. As with economic metrics, these are important for wind farm design and CCD as well. Current practice considers the external impacts of the wind farm design and operation or control in isolation. But there may be potential to further improve the overall farm economic performance while alleviating negative externalities through the employment of CCD. One recent example of this is through the mitigation of wind farm noise impacts (Cao et al., 2020). Noise generation and propagation are affected by the design of individual turbines, their placement in a farm relative to each other and a potential impact point, and how the turbines are operated throughout their lifetime. While Cao et al. (2020) looked only at the layout influence on noise, allowing for varying operational strategies for noise along with the design optimization holds promise for further improvement of the economic performance of the farm while minimizing noise impacts. Other externalities to the environment and communities could be similarly addressed in CCD research.
In the current paper, we have identified four major areas in which important open scientific challenges and opportunities exist for wind farm control: (1) WFFC physics, (2) algorithms and AI, (3) validation and implementation, and (4) integrating control with system design (co-design).
With respect to the first area (WFFC physics), we distinguish between quasi-steady and dynamic control. Quasi-steady induction control has been extensively studied, but remaining opportunities relate to its use for structural load reduction and the use of over-induction for increasing energy extraction. In the field of quasi-static yaw control, further insights in wake shape and its combination with induction control are interesting open research topics. Overall, the effect of shear, veer, and atmospheric stability on wake shape are also still poorly understood. Dynamic control is still in its infancy, with promising first results in the field of individual pitch control and dynamic induction control, both for wake breakup. Whether it is possible to control turbulence at a larger boundary layer scale for improved mixing and energy extraction also remains an interesting fundamental research question. Finally, larger mesoscale effects of wind farms – such as blockage, gravity waves, and wind farm wakes – may also be susceptible to control action. This area is fully unexplored to date and may lead to a lot of opportunities for large wind farms.
In the second area (algorithms and AI), we highlight the opportunities and challenges related to a shift from open-loop control (the current standard, e.g., mostly based on look-up tables) to closed-loop control as a means to reduce uncertainty and to reduce model errors. This becomes particularly important when considering more advanced control approaches in higher-dimensional control spaces. Challenges relate to the choice and construction of appropriate control models (the internal model), the necessary state estimation techniques to construct reliable virtual twins of turbines and flow, and possible trade-offs between observability and the type and number of sensors that are required for state estimation of more complex models. We foresee that current progress in machine learning and artificial intelligence will become a key enabler for solving some of these outstanding problems.
When considering the third area (validation and implementation), we highlight in particular the challenges associated with testing and implementing new control in modern wind farms, with investment costs in the billion-euro range. Direct testing of new ideas on the full scale is simply not possible, and instead, a careful proof-of-concept and validation strategy is required. To this end, we foresee that, in the coming years, the sequence of large-eddy simulations, wind tunnel experiments, and small field campaigns will play an ever larger role. Each of these faces its own challenges, respectively related to model bias, scale similarity, and establishing statistical significance. Moreover, for actual commercial implementation, additional issues arise, such as controller safety and proof of commercial value for different sites, among others.
Finally, we highlight a fourth area (co-design), which we believe to be instrumental in reaching the full potential of wind farm control. With the evolution towards subsidy-free wind energy and more variable energy markets, objectives for wind farm design (and control) are moving from LCoE to value-based metrics that incorporate the time-varying nature of energy prices as well as costs. Incorporating wind farm control in the design optimization process will lead to denser farms that optimally exploit varying conditions. During high electricity prices, control can be used to mitigate wake effects, while during low prices, more emphasis can be given to structural load reduction and lifetime extension. Moreover, in either scenario, ancillary service provision may provide an alternative income base. For instance, in this context, it is interesting to note that the Belgian government recently increased the capacity of the new Princess Elisabeth offshore wind development zone by 60 % (from 2.2 to 3.5 GW), based on the expectation of a shift in the techno-economic optimum as a result of technological progress (O'Brian, 2021).
In summary, the field of WFFC is an active area of research and innovation, with many interesting multidisciplinary challenges and exciting prospects for the increase of the total value of wind energy for society.
O & M | Operations & maintenance |
LCoE | Levelized cost of energy |
AEP | Annual energy production |
TSO | Transmission system operator |
LES | Large-eddy simulations |
LUT | Look-up table |
CFD | Computational fluid dynamics |
DMD | Dynamic mode decomposition |
AI | Artificial intelligence |
WFFC | Wind farm flow control |
HPC | High performance computing |
R & D | Research and development |
PIV | Particle image velocimetry |
TRL | Technology readiness level |
CCD | Control co-design |
CAPEX | Capital expenditure |
OPEX | Operational expenditure |
BOS | Balance of system |
No data sets were used in this article.
Authors contributed as follows to the manuscript: conceptualization of the work – JM, CB, KD, PF, PG, GG, TG, and JWvW; writing of the original draft – JM, CB, KD, PF, PG, TG, and JWvW; review of the original draft – JM, CB, KD, PF, PG, GG, TG, and JWvW.
At least one of the (co-)authors is a member of the editorial board of Wind Energy Science. The peer-review process was guided by an independent editor, and the authors have no other competing interests to declare.
Publisher's note: Copernicus Publications remains neutral with regard to jurisdictional claims in published maps and institutional affiliations.
The views expressed in the article do not necessarily represent the views of the DOE or the U.S. Government. The publisher, by accepting the article for publication, acknowledges that the U.S. Government retains a nonexclusive, paid-up, irrevocable, worldwide license to publish or reproduce the published form of this work or to allow others to do so for U.S. Government purposes.
The authors express their gratitude to Bart Doekemeijer, Wim Munters, and Vlaho Petrović for their useful comments and feedback on this manuscript.
Johan Meyers, Carlo Bottasso, Katherine Dykes, Gregor Giebel, Tuhfe Göçmen, and Jan-Willem van Wingerden have been supported from the FarmConners project, funded by the European Union's Horizon 2020 research and innovation program (grant no. 857844). This work was authored in part by the National Renewable Energy Laboratory, operated by Alliance for Sustainable Energy, LLC, for the U.S. Department of Energy (DOE; contract no. DE-AC36-08GO28308). Funding was provided by the U.S. Department of Energy Office of Energy Efficiency and Renewable Energy Wind Energy Technologies Office.
This paper was edited by Sandrine Aubrun and reviewed by Irene Eguinoa and one anonymous referee.
Abkar, M. and Porté-Agel, F.: Influence of the Coriolis force on the structure and evolution of wind turbine wakes, Phys. Rev. Fluids, 1, 063701, https://doi.org/10.1103/PhysRevFluids.1.063701, 2016. a
Abraham, A. and Hong, J.: Dynamic wake modulation induced by utility-scale wind turbine operation, Applied Energy, 257, 114003, https://doi.org/10.1016/j.apenergy.2019.114003, 2020. a
Adrian, R.: Twenty years of particle image velocimetry, Exp. Fluids, 39, 159–169, https://doi.org/10.1007/s00348-005-0991-7, 2005. a
Ahmad, M. A., Hao, M. R., Ismail, R. M. T. R., and Nasir, A. N. K.: Model-free wind farm control based on random search, in: 2016 IEEE International Conference on Automatic Control and Intelligent Systems (I2CACIS), 22–22 October 2016, Selangor, Malaysia, https://doi.org/10.1109/I2CACIS.2016.7885302, 2016. a
Ahmad, T., Basit, A., Ahsan, M., Coupiac, O., Girard, N., Kazemtabrizi, B., and Matthews, P.: Implementation and Analyses of Yaw Based Coordinated Control of Wind Farms, Energies, 12, 1266, https://doi.org/10.3390/en12071266, 2019. a, b, c
Ainslie, J. F.: Calculating the flowfield in the wake of wind turbines, J. Wind Eng. Indust. Aerodyn., 27, 213–224, 1988. a
Allaerts, D. and Meyers, J.: Boundary-layer development and gravity waves in conventionally neutral wind farms, J. Fluid Mech., 814, 95–130, https://doi.org/10.1017/jfm.2017.11, 2017. a
Allaerts, D. and Meyers, J.: Gravity waves and wind-farm efficiency in neutral and stable conditions, Bound.-Lay. Meteorol., 166, 269–299, https://doi.org/10.1007/s10546-017-0307-5, 2018. a
Allaerts, D. and Meyers, J.: Sensitivity and feedback of wind-farm-induced gravity waves, J. Fluid Mech., 862, 990–1028, https://doi.org/10.1017/jfm.2018.969, 2019. a
Andersen, S. J., Breton, S.-P., Witha, B., Ivanell, S., and Sørensen, J. N.: Global trends in the performance of large wind farms based on high-fidelity simulations, Wind Energ. Sci., 5, 1689–1703, https://doi.org/10.5194/wes-5-1689-2020, 2020. a
Andersson, L. E. and Imsland, L.: Real-time optimization of wind farms using modifier adaptation and machine learning, Wind Energ. Sci., 5, 885–896, https://doi.org/10.5194/wes-5-885-2020, 2020. a
Andersson, L. E., Doekemeijer, B., Van Der Hoek, D., van Wingerden, J.-W., and Imsland, L.: Adaptation of engineering wake models using Gaussian process regression and high-fidelity simulation data, J. Phys.: Conf. Ser., 1618, 022043, https://doi.org/10.1088/1742-6596/1618/2/022043, 2020. a
Andersson, L. E., Anaya-Lara, O., Tande, J. O., Merz, K. O., and Imsland, L.: Wind farm control – Part I: A review on control system concepts and structures, IET Renew. Power Generat., 15, 2085–2108, https://doi.org/10.1049/rpg2.12160, 2021. a, b
Annoni, J., Gebraad, P., and Seiler, P.: Wind farm flow modeling using an input-output reduced-order model, in: American Control Conference (ACC), 6–8 July 2016, Boston, MA, USA, 506–512, https://doi.org/10.1109/ACC.2016.7524964, 2016a. a
Annoni, J., Gebraad, P. M. O., Scholbrock, A. K., Fleming, P. A., and van Wingerden, J. W.: Analysis of axial-induction-based wind plant control using an engineering and a high-order wind plant model, Wind Energy, 19, 1135–1150, 2016b. a
Annoni, J., Bay, C., Johnson, K., Dall'Anese, E., Quon, E., Kemper, T., and Fleming, P.: Wind direction estimation using SCADA data with consensus-based optimization, Wind Energ. Sci., 4, 355–368, https://doi.org/10.5194/wes-4-355-2019, 2019. a
Atkinson, G. and Wilson, D.: Wake measurements on a free yawing 5 m HAWT, in: Proceedings of the 7th BWEA Wind Energy Conference, edited by: Garrad, A., Mechanical Engineering Publications, London, 75–83, ISBN 10:0852985762, ISBN 13:9780852985762, 1985. a
Atkinson, G. and Wilson, D.: Unsteadiness and Structure in the Wake of a Wind Turbine, Wind Eng., 10, 150–162, 1986a. a, b
Atkinson, G. and Wilson, D.: Aerodynamic interaction between two wind turbines, in: Proceedings of the eight BWEA Wind Energy Conference, edited by: Anderson, M. and Powles, S., Mechanical Engineering Publications, London, 107–113, ISBN 0852986076, 1986b. a, b
Barlas, T. K. and van Kuik, G. A. M.: State of the art and prospectives of smart rotor control for wind turbines, J. Phys.: Conf. Ser., 75, 012080, https://doi.org/10.1088/1742-6596/75/1/012080, 2007. a
Baros, S. and Annaswamy, A. M.: Distributed optimal wind farm control for fatigue load minimization: A consensus approach, Int. J. Elect. Power Energ. Syst., 112, 452–459, https://doi.org/10.1016/j.ijepes.2019.04.003, 2019. a
Barter, G. E., Robertson, A., and Musial, W.: A systems engineering vision for floating offshore wind cost optimization, Renew. Energ. Focus, 34, 1–16, https://doi.org/10.1016/j.ref.2020.03.002, 2020. a
Bartl, J. and Sætran, L.: Experimental testing of axial induction based control strategies for wake control and wind farm optimization, J. Phys.: Conf. Ser., 753, 032035, https://doi.org/10.1088/1742-6596/753/3/032035, 2016. a, b
Bartl, J., Ostovan, Y., Uzol, O., and Sætran, L.: Experimental study on power curtailment of three in-line turbines, Energy Procedia, 137, 307–314, https://doi.org/10.1016/j.egypro.2017.10.355, 2017. a, b
Bartl, J., Mühle, F., Schottler, J., Sætran, L., Peinke, J., Adaramola, M., and Hölling, M.: Wind tunnel experiments on wind turbine wakes in yaw: effects of inflow turbulence and shear, Wind Energ. Sci., 3, 329–343, https://doi.org/10.5194/wes-3-329-2018, 2018. a, b
Bastankhah, M. and Porté-Agel, F.: A wind-tunnel investigation of wind-turbine wakes in yawed conditions, J. Phys.: Conf. Ser., 625, 012014, https://doi.org/10.1088/1742-6596/625/1/012014, 2015. a
Bastankhah, M. and Porté-Agel, F.: Experimental and theoretical study of wind turbine wakes in yawed conditions, J. Fluid Mech., 806, 506–541, https://doi.org/10.1017/jfm.2016.595, 2016. a, b, c, d, e
Bastankhah, M. and Porté-Agel, F.: A New Miniature Wind Turbine for Wind Tunnel Experiments. Part I: Design and Performance, Energies, 10, 908, https://doi.org/10.3390/en10070908, 2017a. a
Bastankhah, M. and Porté-Agel, F.: Wind tunnel study of the wind turbine interaction with a boundary-layer flow: Upwind region, turbine performance, and wake region, Phys. Fluids, 29, 065105, https://doi.org/10.1063/1.4984078, 2017b. a, b
Bastankhah, M. and Porté-Agel, F.: Wind farm power optimization via yaw angle control: A wind tunnel study, J. Renew. Sustain. Energ., 11, 023301, https://doi.org/10.1063/1.5077038, 2019. a
Bauweraerts, P. and Meyers, J.: On the Feasibility of Using Large-Eddy Simulations for Real-Time Turbulent-Flow Forecasting in the Atmospheric Boundary Layer, Bound.-Lay. Meteorol., 171, 213–235, https://doi.org/10.1007/s10546-019-00428-5, 2019. a
Bauweraerts, P. and Meyers, J.: Reconstruction of turbulent flow fields from lidar measurements using large-eddy simulation, J. Fluid Mech., 906, A17, https://doi.org/10.1017/jfm.2020.805, 2021. a, b
Bech, J. I., Hasager, C. B., and Bak, C.: Extending the life of wind turbine blade leading edges by reducing the tip speed during extreme precipitation events, Wind Energ. Sci., 3, 729–748, https://doi.org/10.5194/wes-3-729-2018, 2018. a
Becker, M., Ritter, B., Doekemeijer, B., van der Hoek, D., Konigorski, U., Allaerts, D., and van Wingerden, J.-W.: The revised FLORIDyn model: implementation of heterogeneous flow and the Gaussian wake, Wind Energ. Sci., 7, 2163–2179, https://doi.org/10.5194/wes-7-2163-2022, 2022. a
Beiter, P., Kitzing, L., Spitsen, P., Noonan, M., Berkhout, V., and Kikuchi, Y.: Toward global comparability in renewable energy procurement, Joule, 5, 1485–1500, https://doi.org/10.1016/j.joule.2021.04.017, 2021. a
Bellman, R.: Adaptive Control Processes: A Guided Tour, Princeton Legacy Library, Princeton University Press, ISBN 13:978-0691625850, 1961. a
Bertelè, M., Bottasso, C. L., and Schreiber, J.: Wind inflow observation from load harmonics: initial steps towards a field validation, Wind Energ. Sci., 6, 759–775, https://doi.org/10.5194/wes-6-759-2021, 2021. a
Bleeg, J., Purcell, M., Ruisi, R., and Traiger, E.: Wind Farm Blockage and the Consequences of Neglecting Its Impact on Energy Production, Energies, 11, 1609, https://doi.org/10.3390/en11061609, 2018. a, b
Boccolini, M., Bossanyi, E., Bourne, S., Dombrowski, A., Ferraro, G., Harman, K., Harrison, M., Hille, N., Landberg, L., Levick, T., Manjock, A., Mercer, T., Neubert, A., Ruisi, R., and Skeen, N.: DNV position paper – Wind farm control: The route to bankability, Tech. rep., DNV, https://www.dnv.com/Publications/wind-farm-control-198162 (last access: 9 November 2022), 2021. a
Boersma, S., Vali, M., Kühn, M., and van Wingerden, J.-W.: Quasi Linear Parameter Varying modeling for wind farm control using the 2D Navier–Stokes equations, in: IEEE 2016 American Control Conference (ACC), 6–8 July 2016, Boston, MA, USA, 4409–4414, https://doi.org/10.1109/ACC.2016.7525616, 2016. a
Boersma, S., Doekemeijer, B., Gebraad, P., Fleming, P., Annoni, J., Scholbrock, A., Frederik, J., and van Wingerden, J.-W.: A tutorial on control-oriented modeling and control of wind farms, in: 2017 American Control Conference (ACC), 24–26 May 2017, Seattle, WA, USA, 1–18, https://doi.org/10.23919/ACC.2017.7962923, 2017. a
Boersma, S., Doekemeijer, B., Vali, M., Meyers, J., and van Wingerden, J.-W.: A control-oriented dynamic wind farm model: WFSim, Wind Energ. Sci., 3, 75–95, https://doi.org/10.5194/wes-3-75-2018, 2018. a, b
Boersma, S., Doekemeijer, B., Siniscalchi-Minna, S., and van Wingerden, J.: A constrained wind farm controller providing secondary frequency regulation: An LES study, Renew. Energy, 134, 639–652, https://doi.org/10.1016/j.renene.2018.11.031, 2019. a
Bossanyi, E.: Short-Term Wind Prediction Using Kalman Filters, Wind Eng., 9, 1–8, 1985. a
Bossanyi, E.: Individual Blade Pitch Control for Load Reduction, Wind Energy, 6, 119–128, https://doi.org/10.1002/we.76, 2003a. a
Bossanyi, E. A.: Wind Turbine Control for Load Reduction, Wind Energy, 6, 229–244, https://doi.org/10.1002/we.95, 2003b. a
Bossanyi, E.: Combining induction control and wake steering for wind farm energy and fatigue loads optimisation, J. Phys.: Conf. Ser., 1037, 032011, https://doi.org/10.1088/1742-6596/1037/3/032011, 2018. a
Bossanyi, E. and Ruisi, R.: Axial induction controller field test at Sedini wind farm, Wind Energ. Sci., 6, 389–408, https://doi.org/10.5194/wes-6-389-2021, 2021. a, b, c, d
Bossanyi, E. A., Fleming, P. A., and Wright, A. D.: Validation of individual pitch control by field tests on two-and three-bladed wind turbines, IEEE T. Control Syst. Technol., 21, 1067–1078, https://doi.org/10.1109/TCST.2013.2258345, 2013. a
Bossuyt, J., Scott, R., Ali, N., and Cal, R. B.: Quantification of wake shape modulation and deflection for tilt and yaw misaligned wind turbines, J. Fluid Mech., 917, A3, https://doi.org/10.1017/jfm.2021.237, 2021. a
Bottasso, C., Cacciola, S., and Schreiber, J.: Local wind speed estimation, with application to wake impingement detection, Renew. Energy, 116, 155–168, https://doi.org/10.1016/j.renene.2017.09.044, 2018. a
Bottasso, C. L. and Campagnolo, F.: Wind tunnel testing of wind turbines and farms, in: Handbook of Wind Energy Aerodynamics, edited by: Stoevesandt, B., Schepers, G., Fuglsang, P., and Sun, Y., Springer Nature, https://doi.org/10.1007/978-3-030-05455-7_54-1, 2020. a, b, c, d, e, f, g, h, i
Bottasso, C. L. and Schreiber, J.: Online model updating by a wake detector for wind farm control, in: IEEE 2018 Annual American Control Conference (ACC), 27–29 June 2018, Milwaukee, WI, USA, 676–681, https://doi.org/10.23919/ACC.2018.8431626, 2018. a
Bottasso, C. L., Campagnolo, F., and Petrović, V.: Wind tunnel testing of scaled wind turbine models: Beyond aerodynamics, J. Wind Eng. Indust. Aerodynam., 127, 11–28, https://doi.org/10.1016/j.jweia.2014.01.009, 2014. a, b
Bromm, M., Vollmer, L., and Kühn, M.: Numerical investigation of wind turbine wake development in directionally sheared inflow, Wind Energy, 20, 381–395, 2017. a
Brown, K., Houck, D., Maniaci, D. C., and Westergaard, C.: Rapidly Recovering Wind Turbine Wakes with Dynamic Pitch and Rotor Speed Control, in: AIAA Scitech 2021 Forum, 11–15 and 19–21 January 2021, virtual event, https://doi.org/10.2514/6.2021-1182, 2021. a
Buckingham, E.: On physically similar systems; illustrations of the use of dimensional equations, Phys. Rev., 4, 345–376, https://doi.org/10.1103/PhysRev.4.345, 1914. a
Calaf, M., Meneveau, C., and Meyers, J.: Large eddy simulation study of fully developed wind-turbine array boundary layers, Phys. Fluids, 22, 015110, https://doi.org/10.1063/1.3291077, 2010. a
Campagnolo, F., Bottasso, C. L., and Bettini, P.: Design, manufacturing and characterization of aero-elastically scaled wind turbine blades for testing active and passive load alleviation techniques within a ABL wind tunnel, J. Phys.: Conf. Ser., 524, 012061, https://doi.org/10.1088/1742-6596/524/1/012061, 2014. a, b
Campagnolo, F., Petrović, V., Schreiber, J., Nanos, E. M., Croce, A., and Bottasso, C. L.: Wind tunnel testing of a closed-loop wake deflection controller for wind farm power maximization, J. Phys.: Conf. Ser., 753, 032006, https://doi.org/10.1088/1742-6596/753/3/032006, 2016a. a, b, c, d, e
Campagnolo, F., Petrović, V., Bottasso, C. L., and Croce, A.: Wind tunnel testing of wake control strategies, in: Proceedings of the American Control Conference (ACC), 6–8 July 2016, Boston, MA, USA, 513–518, https://doi.org/10.1109/ACC.2016.7524965, 2016b. a
Campagnolo, F., Weber, R., Schreiber, J., and Bottasso, C. L.: Wind tunnel testing of wake steering with dynamic wind direction changes, Wind Energ. Sci., 5, 1273–1295, https://doi.org/10.5194/wes-5-1273-2020, 2020. a, b, c, d, e, f, g, h, i
Cañadillas, B., Foreman, R., Barth, V., Siedersleben, S., Lampert, A., Platis, A., Djath, B., Schulz-Stellenfleth, J., Bange, J., Emeis, S., and Neumann, T.: Offshore wind farm wake recovery: Airborne measurements and its representation in engineering models, Wind Energy, 23, 1249–1265, https://doi.org/10.1002/we.2484, 2020. a, b
Canet, H., Bortolotti, P., and Bottasso, C. L.: On the scaling of wind turbine rotors, Wind Energ. Sci., 6, 601–626, https://doi.org/10.5194/wes-6-601-2021, 2021. a, b, c
Cao, J. F., Zhu, W. J., Shen, W. Z., Sørensen, J. N., and Sun, Z. Y.: Optimizing wind energy conversion efficiency with respect to noise: A study on multi-criteria wind farm layout design, Renew. Energy, 159, 468–485, https://doi.org/10.1016/j.renene.2020.05.084, 2020. a, b
Cassamo, N. and van Wingerden, J.-W.: On the potential of reduced order models for wind farm control: a Koopman dynamic mode decomposition approach, Energies, 13, 6513, https://doi.org/10.3390/en13246513, 2020. a
Centurelli, G., Vollmer, L., Schmidt, J., Dörenkäper, M., Schröder, M., Lukassen, L. J., and Peinke, J.: Evaluating Global Blockage engineering parametrizations with LES, J. Phys.: Conf. Ser., 1934, 012021, https://doi.org/10.1088/1742-6596/1934/1/012021, 2021. a
Chai, T., Lin, C.-L., and Newsom, R. K.: Retrieval of microscale flow structures from high-resolution Doppler lidar data using an adjoint model, J. Atmos. Sci., 61, 1500–1520, 2004. a
Chamorro, L. P. and Porté-Agel, F.: A Wind-Tunnel Investigation of Wind-Turbine Wakes: Boundary-Layer Turbulence Effects, Bound.-Lay. Meteorol., 132, 129–149, https://doi.org/10.1007/s10546-009-9380-8, 2009. a
Chamorro, L. P. and Porté-Agel, F.: Effects of Thermal Stability and Incoming Boundary-Layer Flow Characteristics on Wind-Turbine Wakes: A Wind-Tunnel Study, Bound.-Lay. Meteorol., 136, 515–533, https://doi.org/10.1007/s10546-010-9512-1, 2010. a, b, c
Chatterjee, J. and Dethlefs, N.: Temporal Causal Inference in Wind Turbine SCADA Data Using Deep Learning for Explainable AI, J. Phys.: Conf. Ser., 1618, 022022, https://doi.org/10.1088/1742-6596/1618/2/022022, 2020. a
Chatterjee, J. and Dethlefs, N.: XAI4Wind: A Multimodal Knowledge Graph Database for Explainable Decision Support in Operations & Maintenance of Wind Turbines, arXiv preprint: arXiv 2012.10489, https://doi.org/10.48550/arXiv.2012.10489, 2021. a
Chen, Z., Doekemeijer, B. M., Lin, Z., Xie, Z., Si, Z., Liu, J., and van Wingerden, J.-W.: Data-Driven Modeling & Analysis of Dynamic Wake for Wind Farm Control: A Comparison Study, in: IEEE 2020 Chinese Automation Congress (CAC), 6–8 November 2020, Shanghai, China, 5326–5331, https://doi.org/10.1109/CAC51589.2020.9327624, 2020. a
Christiansen, M. B. and Hasager, C. B.: Wake effects of large offshore wind farms identified from satellite SAR, Remote Sens. Environ., 98, 251–268, https://doi.org/10.1016/j.rse.2005.07.009, 2005. a
Churchfield, M. J., Lee, S., Michalakes, J., and Moriarty, P. J.: A numerical study of the effects of atmospheric and wake turbulence on wind turbine dynamics, J. Turbulence, 13, N14, https://doi.org/10.1080/14685248.2012.668191, 2012. a
Ciri, U., Rotea, M. A., and Leonardi, S.: Model-free control of wind farms: A comparative study between individual and coordinated extremum seeking, Renew. Energy, 113, 1033–1045, 2017. a
Clark, C. E., Barter, G., Shaler, K., and DuPont, B.: Reliability-based layout optimization in offshore wind energy systems, Wind Energy, 25, 125–148, https://doi.org/10.1002/we.2664, 2022. a
Clayton, B. R. and Filby, P.: Measured effects of oblique flows and change in blade pitch angle on performance and wake development of model wind turbines, in: Proceedings of the fourth BWEA Wind Energy Conference, BHRA Fluid Engineering, Cranfield, Bedford, UK, 214–224, ISBN 0906085713, 1982. a, b
Corten, G. P. and Schaak, P.: Heat and Flux. Increase of Wind Farm Production by Reduction of the Axial Induction, Tech. Rep. ECN-RX-03-061, Energy research Centre of the Netherlands, https://publicaties.ecn.nl/PdfFetch.aspx?nr=ECN-RX--03-061 (last access: 17 November 2022), 2003. a
Cossu, C.: Replacing wakes with streaks in wind turbine arrays, Wind Energy, 24, 345–356, https://doi.org/10.1002/we.2577, 2020. a
Cossu, C.: Evaluation of tilt control for wind-turbine arrays in the atmospheric boundary layer, Wind Energ. Sci., 6, 663–675, https://doi.org/10.5194/wes-6-663-2021, 2021a. a
Cossu, C.: Wake redirection at higher axial induction, Wind Energ. Sci., 6, 377–388, https://doi.org/10.5194/wes-6-377-2021, 2021b. a
Couvreux, F., Bazile, E., Rodier, Q., Maronga, B., Matheou, G., Chinita, M. J., Edwards, J., van Stratum, B. J. H., van Heerwaarden, C. C., Huang, J., Moene, A. F., Cheng, A., Fuka, V., Basu, S., Bou-Zeid, E., Canut, G., and Vignon, E.: Intercomparison of Large-Eddy Simulations of the Antarctic Boundary Layer for Very Stable Stratification, Bound.-Lay. Meteorol., 176, 369–400, https://doi.org/10.1007/s10546-020-00539-4, 2020. a
Creaby, J., Li, Y., and Seem, J. E.: Maximizing wind turbine energy capture using multivariable extremum seeking control, Wind Eng., 33, 361–387, 2009. a
Damiani, R., Dana, S., Annoni, J., Fleming, P., Roadman, J., van Dam, J., and Dykes, K.: Assessment of wind turbine component loads under yaw-offset conditions, Wind Energ. Sci., 3, 173–189, https://doi.org/10.5194/wes-3-173-2018, 2018. a, b
Debusscher, C. M. J., Göçmen, T., and Andersen, S. J.: Probabilistic surrogates for flow control using combined control strategies, J. Phys.: Conf. Ser., 2265, 032110, https://doi.org/10.1088/1742-6596/2265/3/032110, 2022. a
Díaz, S., Carta, J. A., and Castañeda, A.: Influence of the variation of meteorological and operational parameters on estimation of the power output of a wind farm with active power control, Renew. Energy, 159, 812–826, https://doi.org/10.1016/j.renene.2020.05.187, 2020. a
Doekemeijer, B., Van Wingerden, J., Boersma, S., and Pao, L.: Enhanced Kalman filtering for a 2D CFD NS wind farm flow model, J. Phys.: Conf. Ser., 753, 052015, https://doi.org/10.1088/1742-6596/753/5/052015, 2016. a
Doekemeijer, B., Boersma, S., Pao, L. Y., and van Wingerden, J.-W.: Ensemble Kalman filtering for wind field estimation in wind farms, in: IEEE 2017 American Control Conference (ACC), 24–26 May 2017, Seattle, WA, USA, 19–24, https://doi.org/10.23919/ACC.2017.7962924, 2017. a
Doekemeijer, B. M., Boersma, S., Pao, L. Y., Knudsen, T., and van Wingerden, J.: Online model calibration for a simplified LES model in pursuit of real-time closed-loop wind farm control, Wind Energ. Sci., 3, 749–765, https://doi.org/10.5194/wes-3-749-2018, 2018. a
Doekemeijer, B. M., Fleming, P. A., and van Wingerden, J. W.: A tutorial on the synthesis and validation of a closed-loop wind farm controller using a steady-state surrogate model, in: American Control Conference (ACC), 10–12 July 2019, Philadelphia, PA, USA, https://doi.org/10.23919/ACC.2019.8815126, 2019. a, b
Doekemeijer, B. M., Boersma, S., Pao, L. Y., Knudsen, T., and van Wingerden, J.: Observability of the ambient conditions in model-based estimation for wind farm control: A focus on static models, Wind Energy, 23, 1777–1791, 2020a. a
Doekemeijer, B. M., van der Hoek, D., and van Wingerden, J.-W.: Closed-loop model-based wind farm control using FLORIS under time-varying inflow conditions, Renew, Energy, 156, 719–730, https://doi.org/10.1016/j.renene.2020.04.007, 2020b. a, b, c
Doekemeijer, B. M., Kern, S., Maturu, S., Kanev, S., Salbert, B., Schreiber, J., Campagnolo, F., Bottasso, C. L., Schuler, S., Wilts, F., Neumann, T., Potenza, G., Calabretta, F., Fioretti, F., and van Wingerden, J.-W.: Field experiment for open-loop yaw-based wake steering at a commercial onshore wind farm in Italy, Wind Energ. Sci., 6, 159–176, https://doi.org/10.5194/wes-6-159-2021, 2021. a, b, c, d, e, f
DTU Wind Energy: PyWake – an AEP calculator for wind farms implemented in Python including a collection of wake models, GitHub [code], https://github.com/DTUWindEnergy/PyWake (last access: 17 November 2022), 2021. a
Duc, T., Coupiac, O., Girard, N., Giebel, G., and Göçmen, T.: Local turbulence parameterization improves the Jensen wake model and its implementation for power optimization of an operating wind farm, Wind Energ. Sci., 4, 287–302, https://doi.org/10.5194/wes-4-287-2019, 2019. a, b
Dykes, K., Veers, P., Lantz, E., Holttinen, H., Carlson, O., Tuohy, A., Sempreviva, A. M., Clifton, A., Rodrigo, J. S., Berry, D., Laird, D., Carron, W., Moriarty, P., Marquis, M., Meneveau, C., Peinke, J., Paquette, J., Johnson, N., Pao, L., Fleming, P., Bottasso, C., Lehtomaki, V., Robertson, A., Muskulus, M., Sethuraman, L., Roberts, O., and Fields, J.: IEA Wind TCP Results of IEA Wind TCP Workshop on a Grand Vision for Wind Energy Technology, Tech. Rep. TP-5000-72437, NREL, https://www.nrel.gov/docs/fy19osti/72437.pdf (last access: 17 November 2022), 2019. a
Dykes, K., King, J., DiOrio, N., King, R., Gevorgian, V., Corbus, D., Blair, N., Anderson, K., Stark, G., Turchi, C., and Moriarty, P.: Opportunities for Research and Development of Hybrid Power Plants, Tech. Rep. TP-5000-75026, NREL, https://www.nrel.gov/docs/fy20osti/75026.pdf (last access: 17 November 2022), 2020. a
Dykes, K., Göçmen, T., Das, K., Pérez-Rúa, J.-A., Larsen, G., and Réthoré, P.-E.: Technology in context: optimization of wind farm and hybrid power plant design, operation and control, DTU Wind Energy, Denmark, 63–74, https://doi.org/10.11581/DTU.00000207, 2021. a
Eguinoa, I., Göçmen, T., Garcia-Rosa, P. B., Das, K., Petrović, V., Kölle, K., Manjock, A., Koivisto, M. J., and Smailes, M.: Wind farm flow control oriented to electricity markets and grid integration: Initial perspective analysis, Adv. Control Appl., 3, e80, https://doi.org/10.1002/adc2.80, 2021. a, b, c
Ela, E., Gevorgian, V., Fleming, P. A., Zhang, Y. C., Singh, M., Muljadi, E., Scholbrock, A. K., Aho, J., Buckspan, A., Pao, L. Y., Singhvi, V., Tuohy, A., Pourbeik, P., Brooks, D., and Bhatt, N.: Active power controls from wind power: bridging the gaps, Tech. rep., National Renewable Energy Laboratory, https://www.nrel.gov/docs/fy14osti/60574.pdf (last access: 17 November 2022), 2014. a
España, G., Aubrun, S., Loyer, S., and Devinant, P.: Spatial study of the wake meandering using modelled wind turbines in a wind tunnel, Wind Energy, 14, 923–937, https://doi.org/10.1002/we.515, 2011. a
Feng, J.: Artificial Intelligence for Wind Energy (AI4Wind): A state of the art report, Tech. Rep. 0180, project: Big Data and Digitalization CCA, DTU Wind Energy E, https://findit.dtu.dk/en/catalog/5c76e625d9001d35522d0a92 (last access: 17 November 2022), 2019. a
Fleming, P., Annoni, J., Shah, J. J., Wang, L., Ananthan, S., Zhang, Z., Hutchings, K., Wang, P., Chen, W., and Chen, L.: Field test of wake steering at an offshore wind farm, Wind Energ. Sci., 2, 229–239, https://doi.org/10.5194/wes-2-229-2017, 2017. a, b
Fleming, P., Annoni, J., Churchfield, M., Martinez-Tossas, L. A., Gruchalla, K., Lawson, M., and Moriarty, P.: A simulation study demonstrating the importance of large-scale trailing vortices in wake steering, Wind Energ. Sci., 3, 243–255, https://doi.org/10.5194/wes-3-243-2018, 2018. a, b, c
Fleming, P., King, J., Simley, E., Roadman, J., Scholbrock, A., Murphy, P., Lundquist, J. K., Moriarty, P., Fleming, K., van Dam, J., Bay, C., Mudafort, R., Jager, D., Skopek, J., Scott, M., Ryan, B., Guernsey, C., and Brake, D.: Continued results from a field campaign of wake steering applied at a commercial wind farm – Part 2, Wind Energ. Sci., 5, 945–958, https://doi.org/10.5194/wes-5-945-2020, 2020. a, b, c, d, e
Fleming, P. A., Gebraad, P. M., Lee, S., van Wingerden, J.-W., Johnson, K., Churchfield, M., Michalakes, J., Spalart, P., and Moriarty, P.: Evaluating techniques for redirecting turbine wakes using SOWFA, Renew. Energy, 70, 211–218, https://doi.org/10.1016/j.renene.2014.02.015, 2014. a, b
Fleming, P. A., Gebraad, P. M. O., Lee, S., van Wingerden, J. W., Johnson, K., Churchfield, M., Michalakes, J., Spalart, P., and Moriarty, P.: Simulation comparison of wake mitigation control strategies for a two-turbine case, Wind Energy, 18, 2135–2143, 2015. a, b
Fleming, P. A., Ning, A., Gebraad, P. M. O., and Dykes, K.: Wind plant system engineering through optimization of layout and yaw control, Wind Energy, 19, 329–344, https://doi.org/10.1002/we.1836, 2016. a
Fleming, P. A., King, J., Dykes, K., Simley, E., Roadman, J., Scholbrock, A. K., Murphy, P., Lundquist, J. K., Moriarty, P., Fleming, K., van Dam, J., Bay, C. J., Mudafort, R., Lopez, H., Skopek, J., Scott, M., Ryan, B., Guernsey, C., and Brake, D.: Initial results from a field campaign of wake steering applied at a commercial wind farm – Part 1, Wind Energ. Sci., 4, 273–285, https://doi.org/10.5194/wes-4-273-2019, 2019. a, b, c, d
Foti, D., Yang, X., and Sotiropoulos, F.: Similarity of wake meandering for different wind turbine designs for different scales, J. Fluid Mech., 842, 5–25, https://doi.org/10.1017/jfm.2018.9, 2018. a
Frandsen, S., Barthelmie, R., Pryor, S., Rathmann, O., Larsen, S., Højstrup, J., and Thøgersen, M.: Analytical modelling of wind speed deficit in large offshore wind farms, Wind Energy, 9, 39–53, 2006. a
Frederik, J. A., Doekemeijer, B. M., Mulders, S. P., and van Wingerden, J.-W.: The helix approach: Using dynamic individual pitch control to enhance wake mixing in wind farms, Wind Energy, 23, 1739–1751, https://doi.org/10.1002/we.2513, 2020a. a, b, c, d, e
Frederik, J. A., Weber, R., Cacciola, S., Campagnolo, F., Croce, A., Bottasso, C., and van Wingerden, J.-W.: Periodic dynamic induction control of wind farms: proving the potential in simulations and wind tunnel experiments, Wind Energ. Sci., 5, 245–257, https://doi.org/10.5194/wes-5-245-2020, 2020b. a, b, c, d
Galinos, C., Kazda, J., Lio, W. H., and Giebel, G.: T2FL: An Efficient Model for Wind Turbine Fatigue Damage Prediction for the Two-Turbine Case, Energies, 13, 1306, https://doi.org/10.3390/en13061306, 2020. a
Garcia-Sanz, M.: Control Co-Design: An engineering game changer, Adv. Control Appl., 1, e18, https://doi.org/10.1002/adc2.18, 2019. a, b, c, d
Garratt, J.: Review: the atmospheric boundary layer, Earth-Sci. Rev., 37, 89–134, https://doi.org/10.1016/0012-8252(94)90026-4, 1994. a
Gebraad, P., Thomas, J. J., Ning, A., Fleming, P. A., and Dykes, K.: Maximization of the annual energy production of wind power plants by optimization of layout and yaw-based wake control, Wind Energy, 20, 97–107, 2017. a
Gebraad, P. M., van Dam, F. C., and van Wingerden, J.: A model-free distributed approach for wind plant control, in: IEEE 2013 American control conference, 17–19 June 2013, Washington, DC, USA, 628–633, https://doi.org/10.1109/ACC.2013.6579907, 2013. a
Gebraad, P. M., Fleming, P. A., and van Wingerden, J.-W.: Comparison of actuation methods for wake control in wind plants, in: IEEE 2015 American Control Conference (ACC), 1–3 July 2015, Chicago, IL, USA, 1695–1701, https://doi.org/10.1109/ACC.2015.7170977, 2015. a, b
Gebraad, P. M. O. and van Wingerden, J. W.: Maximum power-point tracking control for wind farms, Wind Energy, 18, 429–447, 2015. a
Gebraad, P. M. O., Teeuwisse, F. W., van Wingerden, J. W., Fleming, P. A., Ruben, S. D., Marden, J. R., and Pao, L. Y.: Wind plant power optimization through yaw control using a parametric model for wake effects – a CFD simulation study, Wind Energy, 19, 95–114, https://doi.org/10.1002/we.1822, 2016. a, b
Göçmen, T., Giebel, G., Poulsen, N. K., and Sørensen, P. E.: Possible power of down-regulated offshore wind power plants: The PossPOW algorithm, Wind Energy, 22, 205–218, https://doi.org/10.1002/we.2279, 2019. a
Goit, J. and Meyers, J.: Optimal control of energy extraction in wind-farm boundary layers, J. Fluid Mech., 768, 5–50, https://doi.org/10.1017/jfm.2015.70, 2015. a, b, c, d, e, f
Goit, J. P., Munters, W., and Meyers, J.: Optimal Coordinated Control of Power Extraction in LES of a Wind Farm with Entrance Effects, Energies, 9, 29, https://doi.org/10.3390/en9010029, 2016. a, b
Graf, P., Dykes, K., Scott, G., Fields, J., Lunacek, M., Quick, J., and Rethore, P.-E.: Wind Farm Turbine Type and Placement Optimization, J. Phys.: Conf. Ser., 753, 062004, https://doi.org/10.1088/1742-6596/753/6/062004, 2016. a
Grieves, M.: Digital twin: Manufacturing excellence through virtual factory replication, Tech. rep., white paper, https://www.researchgate.net/publication/275211047_Digital_Twin_Manufacturing_Excellence_through_Virtual_Factory_Replication (last access: 17 November 2022), 2014. a
Guo, Y., Rotea, M., and Summers, T.: Stochastic dynamic programming for wind farm power maximization, in: IEEE 2020 American Control Conference (ACC), 1–3 July 2020, Denver, CO, USA, 4824–4829, https://doi.org/10.23919/ACC45564.2020.9148006, 2020. a
Hancock, P. E., Pascheke, F., and Zhang, S.: Wind Tunnel Simulation of Wind Turbine Wakes in Neutral, Stable and Unstable Offshore Atmospheric Boundary Layers, in: Wind Energy – Impact of Turbulence, edited by: Hölling, M., Peinke, J., and Ivanell, S., Springer, Berlin, Heidelberg, 109–114, https://doi.org/10.1007/978-3-642-54696-9_16, 2014. a
Hansen, A. D., Sørensen, P., Blaabjerg, F., and Becho, J.: Dynamic Modelling of Wind Farm Grid Interaction, Wind Eng., 26, 191–210, https://doi.org/10.1260/030952402321039403, 2002. a
Hansen, A. D., Sørensen, P., Iov, F., and Blaabjerg, F.: Centralised power control of wind farm with doubly fed induction generators, Renew. Energy, 31, 935–951, 2006. a, b
Hasager, C., Vejen, F., Bech, J., Skrzypiński, W., Tilg, A.-M., and Nielsen, M.: Assessment of the rain and wind climate with focus on wind turbine blade leading edge erosion rate and expected lifetime in Danish Seas, Renew. Energy, 149, 91–102, https://doi.org/10.1016/j.renene.2019.12.043, 2020. a
Haupt, S. E., Berg, L., Churchfield, M., Kosovic, B., Mirocha, J., and Shaw, W.: Mesoscale to Microscale Coupling for Wind Energy Applications: Addressing the Challenges, J. Phys.: Conf. Ser., 1452, 012076, https://doi.org/10.1088/1742-6596/1452/1/012076, 2020. a
Herbert-Acero, J., Probst, O., Réthoré, P.-E., Larsen, G., and Castillo-Villar, K.: A Review of Methodological Approaches for the Design and Optimization of Wind Farms, Energies, 7, 6930–7016, https://doi.org/10.3390/en7116930, 2014. a
Herges, T. G., Berg, J. C., Bryant, J. T., White, J. R., Paquette, J. A., and Naughton, B. T.: Detailed analysis of a waked turbine using a high-resolution scanning lidar, J. Phys.: Conf. Ser., 1037, 072009, https://doi.org/10.1088/1742-6596/1037/7/072009, 2018. a
Horvat, T., Spudić, V., and Baotić, M.: Quasi-stationary optimal control for wind farm with closely spaced turbines, in: 2012 Proceedings of the 35th International Convention MIPRO, 21–25 May 2012, Opatija, Croatia, 829–834, INSPEC Accession No. 12865459, 2012. a
Houck, D. R.: Review of wake management techniques for wind turbines, Wind Energy, 25, 195–220, https://doi.org/10.1002/we.2668, 2022. a
Howard, K., Hu, L., and Chamorro, L. P.: Characterizing the response of a wind turbine model under complex inflow conditions, Wind Energy, 18, 729–743, https://doi.org/10.1002/we.1724, 2015. a
Howland, M. F. and Dabiri, J. O.: Influence of Wake Model Superposition and Secondary Steering on Model-Based Wake Steering Control with SCADA Data Assimilation, Energies, 14, 52, https://doi.org/10.3390/en14010052, 2021. a
Howland, M. F., Bossuyt, J., Martínez-Tossas, L. A., Meyers, J., and Meneveau, C.: Wake structure in actuator disk models of wind turbines in yaw under uniform inflow conditions, J. Renew. Sustain. Energ., 8, 043301, https://doi.org/10.1063/1.4955091, 2016. a
Howland, M. F., Lele, S. K., and Dabiri, J. O.: Wind farm power optimization through wake steering, P. Natl. Acad. Sci. USA, 116, 14495–14500, https://doi.org/10.1073/pnas.1903680116, 2019. a, b, c, d, e
Howland, M. F., Ghate, A. S., Lele, S. K., and Dabiri, J. O.: Optimal closed-loop wake steering – Part 1: Conventionally neutral atmospheric boundary layer conditions, Wind Energ. Sci., 5, 1315–1338, https://doi.org/10.5194/wes-5-1315-2020, 2020. a
Hu, H., Yang, Z., and Sarkar, P.: Dynamic wind loads and wake characteristics of a wind turbine model in an atmospheric boundary layer wind, Exp. Fluids, 52, 1277–1294, https://doi.org/10.1007/s00348-011-1253-5, 2012. a
Hulsman, P., Andersen, S. J., and Göçmen, T.: Optimizing wind farm control through wake steering using surrogate models based on high-fidelity simulations, Wind Energ. Sci., 5, 309–329, https://doi.org/10.5194/wes-5-309-2020, 2020. a
Iungo, G. V., Viola, F., Camarri, S., Porté-Agel, F., and Gallaire, F.: Linear stability analysis of wind turbine wakes performed on wind tunnel measurements, J. Fluid Mech., 737, 499–526, https://doi.org/10.1017/jfm.2013.569, 2013. a, b
Javid, S. H., Hauth, R. L., Younkins, T. D., Reddoch , T. W., and Barnes, P. R.: A Method for Determining How to Operate and Control Wind Turbine Arrays in Utility Systems, IEEE T. Power Apparat. Syst., 104, 1335–1341, https://doi.org/10.1109/TPAS.1985.319153, 1985. a
Jensen, N. O.: A note on wind generator interaction, Tech. Rep. RISØ-M-2411, https://orbit.dtu.dk/en/publications/a-note-on-wind-generator-interaction (last access: 17 November 2022), 1983. a, b
Jianu, O., Rosen, M. A., and Naterer, G.: Noise pollution prevention in wind turbines: status and recent advances, Sustainability, 4, 1104–1117, 2012. a
Jiménez, A., Crespo, A., Migoya, E., and Garcia, J.: Advances in large-eddy simulation of a wind turbine wake, J. Phys.: Conf. Ser., 75, 012041, https://doi.org/10.1088/1742-6596/75/1/012041, 2007. a
Jiménez, A., Crespo, A., and Migoya, E.: Application of a LES technique to characterize the wake deflection of a wind turbine in yaw, Wind Energy, 13, 559–572, https://doi.org/10.1002/we.380, 2010. a, b
Johnson, K. E. and Fritsch, G.: Assessment of extremum seeking control for wind farm energy production, Wind Eng., 36, 701–715, 2012. a
Johnson, K. E. and Thomas, N.: Wind farm control: Addressing the aerodynamic interaction among wind turbines, in: 2009 American Control Conference, 10–12 June 2009, St. Louis, MO, USA, 2104–2109, https://doi.org/10.1109/ACC.2009.5160152, 2009. a
Jonkman, J. and Shaler, K.: FAST.Farm User's Guide and Theory Manual, Tech. Rep. NREL/TP-5000-7848, National Renewable Energy Laboratory, https://www.nrel.gov/docs/fy21osti/78485.pdf (last access: 17 November 2022), 2021. a
Kalman, R. E.: Mathematical description of linear dynamical systems, J. the Soc. Indust. Appl. Math. Ser. A, 1, 152–192, 1963. a
Kanev, S.: Dynamic wake steering and its impact on wind farm power production and yaw actuator duty, Renew. Energy, 146, 9–15, https://doi.org/10.1016/j.renene.2019.06.122, 2020. a
Kanev, S. K., Savenije, F. J., and Engels, W. P.: Active wake control: An approach to optimize the lifetime operation of wind farms, Wind Energy, 21, 488–501, https://doi.org/10.1002/we.2173, 2018. a
Kang, S., Yang, X., and Sotiropoulos, F.: On the onset of wake meandering for an axial flow turbine in a turbulent open channel flow, J. Fluid Mech, 744, 376–403, https://doi.org/10.1017/jfm.2014.82, 2014. a
Kelley, C. L., Maniaci, D. C., and Resor, B. R.: Scaled Aerodynamic Wind Turbine Design for Wake Similarity, in: AIAA 34th Wind Energy Symposium, AIAA paper 2016-1521, 4–8 January 2016, San Diego, California, USA, https://doi.org/10.2514/6.2016-1521, 2016. a
Kheirabadi, A. C. and Nagamune, R.: A quantitative review of wind farm control with the objective of wind farm power maximization, J. Wind Eng. Indust. Aerodynam., 192, 45–73, https://doi.org/10.1016/j.jweia.2019.06.015, 2019. a, b
Kheirabadi, A. C. and Nagamune, R.: Real-time relocation of floating offshore wind turbine platforms for wind farm efficiency maximization: An assessment of feasibility and steady-state potential, Ocean Eng., 208, 107445, https://doi.org/10.1016/j.oceaneng.2020.107445, 2020. a
Kirby, A. C., Brazell, M. J., Yang, Z., Roy, R., Ahrabi, B. R., Stoellinger, M. K., Sitaraman, J., and Mavriplis, D. J.: Wind farm simulations using an overset hp-adaptive approach with blade-resolved turbine models, Int. J. High Perform. Comput. Appl., 33, 897–923, https://doi.org/10.1177/1094342019832960, 2019. a
Knudsen, T., Bak, T., and Svenstrup, M.: Survey of wind farm control – power and fatigue optimization, Wind Energy, 18, 1333–1351, 2015. a
Kölle, K., Göçmen, T., Eguinoa, I., Das, K., Koivisto, M., Murcia Leon, J., Smailes, M., and Kanellas, P.: FarmConners market showcases for wind farm flow control, in: Proceedings of the 19th Wind Integration Workshop, Energynautics GmbH, 11–12 November 2020, virtual event, ISBN 978-3-9820080-8-0, 2020. a, b
Kristoffersen, J. R. and Christiansen, P.: Horns Rev Offshore Windfarm: Its Main Controller and Remote Control System, Wind Eng., 27, 351–359, https://doi.org/10.1260/030952403322770959, 2003. a, b
Lanfazame, R., Mauro, S., and Messina, M.: Numerical and experimental analysis of micro HAWTs designed for wind tunnel applications, Int. J. Energ. Environ. Eng., 7, 199–210, https://doi.org/10.1007/s40095-016-0202-8, 2016. a
Lanzilao, L. and Meyers, J.: Set-point optimization in wind farms to mitigate effects of flow blockage induced by atmospheric gravity waves, Wind Energ. Sci., 6, 247–271, https://doi.org/10.5194/wes-6-247-2021, 2021. a
Larsen, G. C., Madsen, H. A., Thomsen, K., and Larsen, T. J.: Wake meandering: a pragmatic approach, Wind Energy, 11, 377–395, https://doi.org/10.1002/we.267, 2008. a, b
Liew, J., Göçmen, T., Lio, W. H., and Larsen, G. C.: Streaming dynamic mode decomposition for short-term forecasting in wind farms, Wind Energy, 25, 719–734, https://doi.org/10.1002/we.2694, 2022. a
Liew, J., Andersen, S. J., Troldborg, N., and Göçmen, T.: LES verification of HAWC2Farm aeroelastic wind farm simulations with wake steering and load analysis, J. Phys.: Conf. Ser., 2265, 022069, https://doi.org/10.1088/1742-6596/2265/2/022069, 2022. a
Lin, C.-L., Chai, T., and Sun, J.: Retrieval of flow structures in a convective boundary layer using an adjoint model: Identical twin experiments, J. Atmos. Sci., 58, 1767–1783, 2001. a
Lin, C.-T.: Structural controllability, IEEE T. Automat. Control, 19, 201–208, https://doi.org/10.1109/TAC.1974.1100557, 1974. a
Lissaman, P. B. S.: Energy Effectiveness of Arbitrary Arrays of Wind Turbines, J. Energy, 3, 323–328, 1979. a
Liu, Y., Qin, H., Zhang, Z., Pei, S., Jiang, Z., Feng, Z., and Zhou, J.: Probabilistic spatiotemporal wind speed forecasting based on a variational Bayesian deep learning model, Appl. Energy, 260, 114259, https://doi.org/10.1016/j.apenergy.2019.114259, 2020. a
Lomas, C.: Fundamentals of hot wire anemometry, Cambridge University Press, ISBN 0521303400, 1986. a
Lundquist, J. K., Churchfield, M. J., Lee, S., and Clifton, A.: Quantifying error of lidar and sodar Doppler beam swinging measurements of wind turbine wakes using computational fluid dynamics, Atmos. Meas. Tech., 8, 907–920, https://doi.org/10.5194/amt-8-907-2015, 2015. a
Ma, K., Zhu, J., Soltani, M., Hajizadeh, A., and Chen, Z.: Optimal Power Dispatch of an Offshore Wind Farm under Generator Fault, Appl. Sci., 9, 1184, https://doi.org/10.3390/app9061184, 2019. a
Madsen, H. A., Zahle, F., Meng, F., Barlas, T., Rasmussen, F., and Rudolf, R. T.: Initial performance and load analysis of the LowWind turbine in comparison with a conventional turbine, J. Phys.: Conf. Ser., 1618, 032011, https://doi.org/10.1088/1742-6596/1618/3/032011, 2020. a, b
Magnusson, M. and Smedman, A.-S.: Influence of Atmospheric Stability on Wind Turbine Wakes, Wind Eng., 18, 139–152, 1994. a
Manjock, A., Madariaga, A., Eguinoa, I., Hille, N., Pires, O., Kölle, K., Bayo, R. T., Argyriadis, K., Göcmen, T., Das, K., and Nwobu, J.: Position paper on certification, standardisation, and other regulatory issues of Wind Farm Control, Tech. rep., ORE Catapult, DNV GL, Sintef, DTU, CENER, https://cordis.europa.eu/project/id/857844/results (last access: 17 November 2022), 2020. a, b
Marden, J. R., Ruben, S. D., and Pao, L. Y.: A Model-Free Approach to Wind Farm Control Using Game Theoretic Methods, IEEE T. Control Syst. Technol., 21, 1207–1214, https://doi.org/10.1109/TCST.2013.2257780, 2013. a, b
Marques, A. T., Batalha, H., Rodrigues, S., Costa, H., Pereira, M. J. R., Fonseca, C., Mascarenhas, M., and Bernardino, J.: Understanding bird collisions at wind farms: An updated review on the causes and possible mitigation strategies, Biol. Conserv., 179, 40–52, https://doi.org/10.1016/j.biocon.2014.08.017, 2014. a
Martínez-Tossas, L. A., Churchfield, M. J., and Meneveau, C.: Optimal smoothing length scale for actuator line models of wind turbine blades based on Gaussian body force distribution, Wind Energy, 20, 1083–1096, https://doi.org/10.1002/we.2081, 2017. a
Martínez-Tossas, L. A., Churchfield, M. J., Yilmaz, A. E., Sarlak, H., Johnson, P. L., Sørensen, J. N., Meyers, J., and Meneveau, C.: Comparison of four large-eddy simulation research codes and effects of model coefficient and inflow turbulence in actuator-line-based wind turbine modeling, J. Renew. Sustain. Energ., 10, 033301, https://doi.org/10.1063/1.5004710, 2018. a
Martínez-Tossas, L. A., Annoni, J., Fleming, P. A., and Churchfield, M. J.: The aerodynamics of the curled wake: a simplified model in view of flow control, Wind Energ. Sci., 4, 127–138, https://doi.org/10.5194/wes-4-127-2019, 2019. a
Martínez-Tossas, L. A., Branlard, E., Shaler, K., Vijayakumar, G., Ananthan, S., Sakievich, P., and Jonkman, J.: Numerical investigation of wind turbine wakes under high thrust coefficient, Wind Energy, 25, 605–617, https://doi.org/10.1002/we.2688, 2022. a
Marugán, A. P., Márquez, F. P. G., Perez, J. M. P., and Ruiz-Hernández, D.: A survey of artificial neural network in wind energy systems, Appl. Energy, 228, 1822–1836, https://doi.org/10.1016/j.apenergy.2018.07.084, 2018. a
Mbuvha, R., Mongwe, W. T., and Marwala, T.: Separable Shadow Hamiltonian Hybrid Monte Carlo for Bayesian Neural Network Inference in wind speed forecasting, Energy AI, 6, 100108, https://doi.org/10.1016/j.egyai.2021.100108, 2021. a
McTavish, S., Feszty, D., and Nitzsche, F.: An experimental and computational assessment of blockage effects on wind turbine wake development, Wind Energy, 17, 1515–1529, https://doi.org/10.1002/we.1648, 2014. a
Medici, D. and Alfredsson, P. H.: Measurements behind model wind turbines: further evidence of wake meandering, Wind Energy, 11, 211–217, https://doi.org/10.1002/we.247, 2008. a
Medici, D. and Dahlberg, J.: Potential improvement of wind turbine array efficiency by active wake control (AWC), in: Proceedings of the European Wind Energy Conference and Exhibition (EWEA), CD-ROM proceedings, 16–19 June 2003, Madrid, Spain, 65–84, 2003. a
Meyer Forsting, A. R., Troldborg, N., and Gaunaa, M.: The flow upstream of a row of aligned wind turbine rotors and its effect on power production, Wind Energy, 20, 63–77, https://doi.org/10.1002/we.1991, 2017. a
Meyer Forsting, A. R., Pirrung, G. R., and Ramos-García, N.: A vortex-based tip/smearing correction for the actuator line, Wind Energ. Sci., 4, 369–383, https://doi.org/10.5194/wes-4-369-2019, 2019. a
Mikkelsen, T., Angelou, N., Hansen, K., Sjöholm, M., Harris, M., Slinger, C., Hadley, P., Scullion, R., Ellis, G., and Vives, G.: A spinner-integrated wind lidar for enhanced wind turbine control, Wind Energy, 16, 625–643, 2013. a
Mittal, A., Sreenivas, K., Taylor, L. K., Hereth, L., and Hilbert, C. B.: Blade-resolved simulations of a model wind turbine: effect of temporal convergence, Wind Energy, 19, 1761–1783, https://doi.org/10.1002/we.1949, 2016. a
Moriarty, P., Hamilton, N., Debnath, M., Herges, T., Isom, B., Lundquist, J. K., Maniaci, D., Naughton, B., Pauly, R., Roadman, J., Shaw, W., van Dam, J., and Wharton, S.: American WAKE experimeNt (AWAKEN), Tech. Rep. NREL/TP-5000-75789, National Renewable Energy Laboratory, https://www.nrel.gov/docs/fy20osti/75789.pdf (last access: 17 November 2022), 2020. a
Muñoz-Esparza, D. and Kosović, B.: Generation of Inflow Turbulence in Large-Eddy Simulations of Nonneutral Atmospheric Boundary Layers with the Cell Perturbation Method, Mon. Weather Rev., 146, 1889–1909, https://doi.org/10.1175/MWR-D-18-0077.1, 2018. a
Muñoz-Esparza, D., Kosović, B., and Mirocha, J.: Bridging the Transition from Mesoscale to Microscale Turbulence in Numerical Weather Prediction Models, Bound.-Lay. Meteorol., 153, 409–440, https://doi.org/10.1007/s10546-014-9956-9, 2014. a
Munters, W. and Meyers, J.: Effect of wind turbine response time on optimal dynamic induction control of wind farms, J. Phys.: Conf. Ser., 753, 052007, https://doi.org/10.1088/1742-6596/753/5/052007, 2016. a
Munters, W. and Meyers, J.: An optimal control framework for dynamic induction control of wind farms and their interaction with the atmospheric boundary layer, Philos. T. Roy. Soc. Lond. A, 375, 20160100, https://doi.org/10.1098/rsta.2016.0100, 2017. a, b
Munters, W. and Meyers, J.: Dynamic Strategies for Yaw and Induction Control of Wind Farms Based on Large-Eddy Simulation and Optimization, Energies, 11, 177, https://doi.org/10.3390/en11010177, 2018a. a, b, c, d, e, f
Munters, W. and Meyers, J.: Optimal dynamic induction and yaw control of wind farms: effects of turbine spacing and layout, J. Phys.: Conf. Ser., 1037, 032015, https://doi.org/10.1088/1742-6596/1037/3/032015, 2018b. a, b
Munters, W. and Meyers, J.: Towards practical dynamic induction control of wind farms: analysis of optimally controlled wind-farm boundary layers and sinusoidal induction control of first-row turbines, Wind Energ. Sci., 3, 409–425, https://doi.org/10.5194/wes-3-409-2018, 2018c. a, b, c, d, e, f, g, h, i, j
Munters, W., Meneveau, C., and Meyers, J.: Turbulent Inflow Precursor Method with Time-Varying Direction for Large-Eddy Simulations and Applications to Wind Farms, Bound.-Lay. Meteorol., 159, 305–328, https://doi.org/10.1007/s10546-016-0127-z, 2016. a
Nanos, E., Letizia, S., Barreiro Clemente, D., Wang, C., Rotea, M., Iungo, V., and Bottasso, C.: Vertical wake deflection for offshore floating wind turbines by differential ballast control, J. Phys.: Conf. Ser., 1618, 022047, https://doi.org/10.1088/1742-6596/1618/2/022047, 2020. a, b
Nanos, E. M., Bottasso, C. L., Campagnolo, F., Mühle, F., Letizia, S., Iungo, G. V., and Rotea, M. A.: Design, steady performance and wake characterization of a scaled wind turbine with pitch, torque and yaw actuation, Wind Energ. Sci., 7, 1263–1287, https://doi.org/10.5194/wes-7-1263-2022, 2022. a
National Renewable Energy Laboratory: FLORIS Wake Modeling Utility, Tech. rep., Zenodo [code], https://doi.org/10.5281/zenodo.5062687, 2021. a
Neuhaus, L., Berger, F., Peinke, J., and Hölling, M.: Exploring the capabilities of active grids, Exp. Fluids, 62, 130, https://doi.org/10.1007/s00348-021-03224-5, 2021. a
Ning, A., Dykes, K., and Quick, J.: Systems engineering and optimization of wind turbines and power plants, in: Wind Energy Modeling and Simulation – Volume 2: Turbine and System, edited by: Veers, P., Institution of Engineering and Technology, London, https://doi.org/10.1049/PBPO125G_ch7, 2019. a
Nygaard, N. G. and Hansen, S. D.: Wake effects between two neighbouring wind farms, J. Phys.: Conf. Ser., 753, 032020, https://doi.org/10.1088/1742-6596/753/3/032020, 2016. a
Nygaard, N. G. and Newcombe, A. C.: Wake behind an offshore wind farm observed with dual-Doppler radars, J. Phys.: Conf. Ser., 1037, 072008, https://doi.org/10.1088/1742-6596/1037/7/072008, 2018. a
O'Brian, H.: Belgian government expands offshore wind zone, https://www.windpowermonthly.com/article/1730860/belgian-government-expands-offshore-wind-zone (last access: 17 November 2022), 2021. a
Ott, S. and Nielsen, M.: Developments of the offshore wind turbine wake model Fuga, DTU Wind Energy E No. 0046, DTU, https://backend.orbit.dtu.dk/ws/portalfiles/portal/118472784/DTU_Wind_Energy_E_0046.pdf (last access: 17 November 2022), 2014. a
Parandoush, P. and Lin, D.: A review on additive manufacturing of polymer-fiber composites, Compos. Struct., 182, 36–53, 2017. a
Park, J., Kwon, S., and Law, K. H.: Wind farm power maximization based on a cooperative static game approach, in: Active and Passive Smart Structures and Integrated Systems 2013, edited by: Sodano, H., Int. Soc. Optics Photon. SPIE, 8688, 86880R, https://doi.org/10.1117/12.2009618, 2013. a
Parkin, P., Holm, R., and Medici, D.: The application of PIV to the wake of a wind turbine in yaw, DLR-Mitteilung, 155–162, ISSN 14348462, 2001. a
Pedersen, M. M. and Larsen, G. C.: Integrated wind farm layout and control optimization, Wind Energ. Sci., 5, 1551–1566, https://doi.org/10.5194/wes-5-1551-2020, 2020. a, b
Perez-Moreno, S. S., Dykes, K., Merz, K. O., and Zaaijer, M. B.: Multidisciplinary design analysis and optimisation of a reference offshore wind plant, J. Phys.: Conf. Ser., 1037, 042004, https://doi.org/10.1088/1742-6596/1037/4/042004, 2018. a, b
Pérez-Rúa, J. A., Stolpe, M., Das, K., and Cutululis, N.: Global Optimization of Offshore Wind Farm Collection Systems, IEEE T. Power Syst., 35, 2256–2267, https://doi.org/10.1109/tpwrs.2019.2957312, 2020. a
Pimenta, F., Pacheco, J., Branco, C., Teixeira, C., and Magalhaes, F.: Development of a digital twin of an onshore wind turbine using monitoring data, IOP J. Phys.: Conf. Ser., 1618, 022065, https://doi.org/10.1088/1742-6596/1618/2/022065, 2020. a
Platis, A., Siedersleben, S. K., Bange, J., Lampert, A., Bärfuss, K., Hankers, R., Cañadillas, B., Foreman, R., Schulz-Stellenfleth, J., Djath, B., Neumann, T., and Emeis, S.: First in situ evidence of wakes in the far field behind offshore wind farms, Scient. Rep., 8, 2163, https://doi.org/10.1038/s41598-018-20389-y, 2018. a
Porté-Agel, F., Wu, Y.-T., Lu, H., and Conzemius, R. J.: Large-eddy simulation of atmospheric boundary layer flow through wind turbines and wind farms, J. Wind Eng. Indust. Aerodynam., 99, 154–168, https://doi.org/10.1016/j.jweia.2011.01.011, 2011. a
Porté-Agel, F., Bastankhah, M., and Shamsoddin, S.: Wind-Turbine and Wind-Farm Flows: A Review, Bound.-Lay. Meteorol., 174, 1–59, https://doi.org/10.1007/s10546-019-00473-0, 2020. a
Qiu, H., Min, F., and Yang, Y.: Fiber optic sensing technologies potentially applicable for hypersonic wind tunnel harsh environments, Adv. Aerodynam., 2, 1–22, 2020. a
Quaranta, H. U., Bolnot, H., and Leweke, T.: Long-wave instability of a helical vortex, J. Fluid Mech., 780, 687–716, https://doi.org/10.1017/jfm.2015.479, 2015. a, b
Quick, J., King, J., King, R. N., Hamlington, P. E., and Dykes, K.: Wake steering optimization under uncertainty, Wind Energ. Sci., 5, 413–426, https://doi.org/10.5194/wes-5-413-2020, 2020. a
Raach, S., Schlipf, D., Haizmann, F., and Cheng, P. W.: Three dimensional dynamic model based wind field reconstruction from lidar data, J. Phys.: Conf. Ser., 524, 012005, https://doi.org/10.1088/1742-6596/524/1/012005, 2014. a
Raach, S., Schlipf, D., and Cheng, P. W.: Lidar-based wake tracking for closed-loop wind farm control, Wind Energ. Sci., 2, 257–267, https://doi.org/10.5194/wes-2-257-2017, 2017. a
Réthoré, P.-E., Fuglsang, P., Larsen, G. C., Buhl, T., Larsen, T. J., and Madsen, H. A.: TOPFARM: Multi-fidelity optimization of wind farms, Wind Energy, 17, 1797–1816, https://doi.org/10.1002/we.1667, 2014. a
Riva, A. D., Hethey, J., Luers, S., Wallasch, A.-K., Rehfeldt, K., Duffy, A., Weir, D. E., Stenkvist, M., Uihlein, A., Stehly, T. J., Lantz, E., and Wiser, R.: IEA Wind TCP Task 26: Wind Technology, Cost, and Performance Trends in Denmark, Germany, Ireland, Norway, Sweden, the European Union, and the United States: 2008–2016, Tech. rep., NREL – National Renewable Energy Lab., Golden, CO, USa, https://www.nrel.gov/docs/fy19osti/71844.pdf (last access: 17 November 2022), 2019. a
Riva, R., Liew, J., Friis-Møller, M., Dimitrov, N., Barlas, E., Réthoré, P.-E., and Beržonskis, A.: Wind farm layout optimization with load constraints using surrogate modelling, J. Phys.: Conf. Ser., 1618, 042035, https://doi.org/10.1088/1742-6596/1618/4/042035, 2020. a, b
Rotea, M. A.: Dynamic programming framework for wind power maximization, IFAC Proc. Vol., 47, 3639–3644, 2014. a
Rott, A., Doekemeijer, B., Seifert, J. K., van Wingerden, J.-W., and Kühn, M.: Robust active wake control in consideration of wind direction variability and uncertainty, Wind Energ. Sci., 3, 869–882, https://doi.org/10.5194/wes-3-869-2018, 2018. a, b
RWE Renewables: Offshore wind industry consortium gears up to conduct first of a kind full-scale measurement campaign focused on `Global Blockage Effect', https://www.carbontrust.com/news-and-events/news/offshore-wind-global-blockage-effect (last access: 17 November 2022), 2021. a
Schepers, J. G. and van der Pijl, S. P.: Improved modelling of wake aerodynamics and assessment of new farm control strategies, J. Phys.: Conf. Ser., 75, 012039, https://doi.org/10.1088/1742-6596/75/1/012039, 2007. a
Schlipf, D., Schlipf, D. J., and Kühn, M.: Nonlinear model predictive control of wind turbines using LIDAR, Wind Energy, 16, 1107–1129, 2013. a
Schlueter, R., Dorsey, J., Lotfalian, M., Park, G., and Shayanfar, M.: Methods of reducing wind power changes from large turbine arrays, IEEE T. Power Apparat. Syst., 102, 1642–1650, https://doi.org/10.1109/TPAS.1983.317900, 1983. a
Schneemann, J., Theuer, F., Rott, A., Dörenkämper, M., and Kühn, M.: Offshore wind farm global blockage measured with scanning lidar, Wind Energ. Sci., 6, 521–538, https://doi.org/10.5194/wes-6-521-2021, 2021. a, b
Schottler, J., Holling, A., Peinke, J., and Holling, M.: Design and implementation of a controllable model wind turbine for experimental studies, J. Phys.: Conf. Ser., 753, 506–541, https://doi.org/10.1088/1742-6596/753/7/072030, 2016. a
Schreiber, J., Nanos, E. M., Campagnolo, F., and Bottasso, C. L.: Verification and Calibration of a Reduced Order Wind Farm Model by Wind Tunnel Experiments, J. Phys.: Conf. Ser., 854, 012041, https://doi.org/10.1088/1742-6596/854/1/012041, 2017. a, b
Schreiber, J., Bottasso, C. L., Salbert, B., and Campagnolo, F.: Improving wind farm flow models by learning from operational data, Wind Energ. Sci., 5, 647–673, https://doi.org/10.5194/wes-5-647-2020, 2020a. a, b, c, d
Schreiber, J., Bottasso, C. L., and Bertelè, M.: Field testing of a local wind inflow estimator and wake detector, Wind Energ. Sci., 5, 867–884, https://doi.org/10.5194/wes-5-867-2020, 2020b. a
Scott, R., Bossuyt, J., and Cal, R.: Characterizing tilt effects on wind plants, J. Renew. Sustain. Energ., 12, 2135–2143, https://doi.org/10.1063/5.0009853, 2020. a
Segalini, A. and Dahlberg, J.-A.: Blockage effects in wind farms, Wind Energy, 23, 120–128, https://doi.org/10.1002/we.2413, 2020. a
Shapiro, C. R., Bauweraerts, P., Meyers, J., Meneveau, C., and Gayme, D. F.: Model-based receding horizon control of wind farms for secondary frequency regulation, Wind Energy, 20, 1261–1275, https://doi.org/10.1002/we.2093, 2017a. a, b
Shapiro, C. R., Meyers, J., Meneveau, C., and Gayme, D. F.: Dynamic wake modeling and state estimation for improved model-based receding horizon control of wind farms, in: IEEE 2017 American Control Conference (ACC), 24–26 May 2017, Seattle, WA, USA, 709–716, https://doi.org/10.23919/ACC.2017.7963036, 2017b. a
Shapiro, C. R., Gayme, D. F., and Meneveau, C.: Modelling yawed wind turbine wakes: a lifting line approach, J. Fluid Mech., 841, R1, https://doi.org/10.1017/jfm.2018.75, 2018a. a
Shapiro, C. R., Meyers, J., Meneveau, C., and Gayme, D. F.: Wind farms providing secondary frequency regulation: evaluating the performance of model-based receding horizon control, Wind Energ. Sci., 3, 11–24, https://doi.org/10.5194/wes-3-11-2018, 2018b. a
Shapiro, C. R., Starke, G. M., and Gayme, D. F.: Turbulence and Control of Wind Farms, Annu. Rev. Control Robot. Auton. Syst., 5, 579–602, https://doi.org/10.1146/annurev-control-070221-114032, 2022. a
Siemens Gamesa Renewable Energy: Siemens Gamesa now able to actively dictate wind flow at offshore wind locations, https://www.siemensgamesa.com/en-int/newsroom/2019/11/191126-siemens-gamesa-wake-adapt-en (last access: 23 August 2021), 2019. a, b, c, d
Simley, E., Pao, L., Frehlich, R., Jonkman, B., and Kelley, N.: Analysis of wind speed measurements using continuous wave LIDAR for wind turbine control, in: 49th AIAA Aerospace Sciences Meeting including the New Horizons Forum and Aerospace Exposition, 4–7 January 2011, Orlando, Florida, p. 263, https://doi.org/10.2514/6.2011-263, 2011. a
Simley, E., Pao, L. Y., Frehlich, R., Jonkman, B., and Kelley, N.: Analysis of light detection and ranging wind speed measurements for wind turbine control, Wind Energy, 17, 413–433, 2014a. a
Simley, E., Pao, L. Y., Gebraad, P., and Churchfield, M.: Investigation of the Impact of the Upstream Induction Zone on LIDAR Measurement Accuracy for Wind Turbine Control Applications using Large-Eddy Simulation, J. Phys.: Conf. Ser., 524, 12003, https://doi.org/10.1088/1742-6596/524/1/012003, 2014b. a
Simley, E., Fleming, P., Girard, N., Alloin, L., Godefroy, E., and Duc, T.: Results from a wake-steering experiment at a commercial wind plant: investigating the wind speed dependence of wake-steering performance, Wind Energ. Sci., 6, 1427–1453, https://doi.org/10.5194/wes-6-1427-2021, 2021. a, b
Simpson, J., Loth, E., and Dykes, K.: Cost of Valued Energy for design of renewable energy systems, Renew. Energy, 153, 290–300, https://doi.org/10.1016/j.renene.2020.01.131, 2020. a, b
Siniscalchi-Minna, S., Bianchi, F. D., De-Prada-Gil, M., and Ocampo-Martinez, C.: A wind farm control strategy for power reserve maximization, Renew. Energy, 131, 37–44, https://doi.org/10.1016/j.renene.2018.06.112, 2019. a
Smith, R. B.: Gravity wave effects on wind farm efficiency, Wind Energy, 13, 449–458, 2010. a, b
Soltani, M. N., Knudsen, T., Svenstrup, M., Wisniewski, R., Brath, P., Ortega, R., and Johnson, K.: Estimation of rotor effective wind speed: A comparison, IEEE T. Control Syst. Technol., 21, 1155–1167, https://doi.org/10.1109/TCST.2013.2260751, 2013. a
Sørensen, J. N.: Instability of helical tip vortices in rotor wakes, J. Fluid Mech., 682, 1–4, https://doi.org/10.1017/jfm.2011.277, 2011. a
Sørensen, J. N., Shen, W. Z., and Munduate, X.: Analysis of wake states by a full-field actuator disc model, Wind Energy, 1, 73–88, https://doi.org/10.1002/(SICI)1099-1824(199812)1:2<73::AID-WE12>3.0.CO;2-L, 1998. a
Sørensen, J. N. and Shen, W. Z.: Numerical Modeling of Wind Turbine Wakes, J. Fluid. Eng., 124, 393–399, https://doi.org/10.1115/1.1471361, 2002. a
Sørensen, P. and Nielsen, T.: Recalibrating wind turbine wake model parameters – validating the wake model performance for large offshore wind farms, in: Proceedings of the European Wind Energy Conference and Exhibition, 27–February–2 March 2006, Athens, Greece, p. BL3.114, ISBN 9781622764679, 2006. a
Sørensen, P., Hansen, A., Janosi, L., Bech, J., and Bak-Jensen, B.: Simulation of interaction between wind farm and power system, Tech. Rep. Risø-R-1281(EN), Risø National Laboratory, Denmark, https://backend.orbit.dtu.dk/ws/portalfiles/portal/7712591/ris_r_1281.pdf (last access: 17 November 2022), 2002. a
Sœrensen, P., Hansen, A. D., Iov, F., Blaabjerg, F., and Donovan, M. H.: Wind farm models and control strategies, Tech. Rep. Risø-R-1464(EN), Risø National Laboratory, Denmark, https://backend.orbit.dtu.dk/ws/portalfiles/portal/7711074/ris_r_1464.pdf (last access: 17 Novembver 2022), 2005. a
Sprague, M. A., Ananthan, S., Vijayakumar, G., and Robinson, M.: ExaWind: A multifidelity modeling and simulation environment for wind energy, J. Phys.: Conf. Ser., 1452, 012071, https://doi.org/10.1088/1742-6596/1452/1/012071, 2020. a
Spruce, C. J.: Simulation and Control of Windfarms, PhD dissertation, University of Oxford, Department of Engineering Science, https://ora.ox.ac.uk/objects/uuid:24f51a31-e2f9-422f-9837-3c28cfe12ccc (last access: 17 November 2022), 1993. a
Stanley, A. P. and Ning, A.: Coupled Wind Turbine Design and Layout Optimization with Non-Homogeneous Wind Turbines, Wind Energ. Sci., 4, 99–114, https://doi.org/10.5194/wes-4-99-2019, 2019. a
Stanley, A. P., Ning, A., and Dykes, K.: Benefits of Two Turbine Rotor Diameters and Hub Heights in the Same Wind Farm, in: Wind Energy Symposium, 8–12 January 2018, Kissimmee, FL, https://doi.org/10.2514/6.2018-2016, 2018. a
Stanley, A. P. J., Ning, A., and Dykes, K.: Optimization of Turbine Design in Wind Farms with Multiple Hub Heights, Using Exact Analytic Gradients and Structural Constraints, Wind Energy, 22, 605–619, https://doi.org/10.1002/we.2310, 2019. a
Stanley, A. P. J., King, J., and Ning, A.: Wind Farm Layout Optimization with Loads Considerations, J. Phys.: Conf. Ser., 1452, 012072, https://doi.org/10.1088/1742-6596/1452/1/012072, 2020. a
Steinbuch, M., de Boer, W., Bosgra, O., Peters, S., and Ploeg, J.: Optimal control of wind power plants, J. Wind Eng. Indust. Aerodynam., 27, 237–246, https://doi.org/10.1016/0167-6105(88)90039-6, 1988. a, b
Stevens, R. J. and Meneveau, C.: Flow Structure and Turbulence in Wind Farms, Annu. Rev. Fluid Mech., 49, 311–339, https://doi.org/10.1146/annurev-fluid-010816-060206, 2017. a, b
Stevens, R. J., Graham, J., and Meneveau, C.: A concurrent precursor inflow method for Large Eddy Simulations and applications to finite length wind farms, Renew. Energy, 68, 46–50, https://doi.org/10.1016/j.renene.2014.01.024, 2014. a
Stock, A., Cole, M., Leithead, W., and Amos, L.: Distributed Control of Wind Farm Power Set Points to Minimise Fatigue Loads, in: 2020 American Control Conference (ACC), 1–3 July 2020, Denver, CO, USA, 4843–4848, https://doi.org/10.23919/ACC45564.2020.9147732, 2020. a
Storey, R. C., Norris, S. E., and Cater, J. E.: An actuator sector method for efficient transient wind turbine simulation, Wind Energy, 18, 699–711, https://doi.org/10.1002/we.1722, 2015. a
Stull, R. B.: An Introduction to Boundary Layer Meteorology, Kluwer, Dordrecht, https://doi.org/10.1007/978-94-009-3027-8, 1988. a
Sundén, B. and Wu, Z.: On icing and icing mitigation of wind turbine blades in cold climate, J. Energ. Resour. Technol., 137, 051203, https://doi.org/10.1115/1.4030352, 2015. a
Taylor, G., Milborrow, D., McIntosh, D., and Swift-Hokk, D.: Wake measurements on the Nibe windmills, in: Proceedings of the 7th British Wind Energy Association Conference, 27–29 March 1985, Oxford, 67–74, ISBN 10:0852985762, ISBN 13:9780852985762, 1985. a
Teixeira, M. A. C.: The physics of orographic gravity wave drag, Front. Phys., 2, 43, https://doi.org/10.3389/fphy.2014.00043, 2014. a
Tian, J., Su, C., Soltani, M., and Chen, Z.: Active power dispatch method for a wind farm central controller considering wake effect, in: IECON 2014 – 40th Annual Conference of the IEEE Industrial Electronics Society, 29 October–1 November 2014, Dallas, TX, USA, 5450–5456, https://doi.org/10.1109/IECON.2014.7049333, 2014. a
Torres, P., van Wingerden, J.-W., and Verhaegen, M.: Modeling of the flow in wind farms for total power optimization, in: IEEE 2011 9th IEEE International Conference on Control and Automation (ICCA), 19–21 December 2011, Santiago, Chile, 963–968, https://doi.org/10.1109/ICCA.2011.6137984, 2011. a
Troldborg, N., Sørensen, J. N., and Mikkelsen, R.: Actuator Line Simulation of Wake of Wind Turbine Operating in Turbulent Inflow, J. Phys.: Conf. Ser., 75, 012063, https://doi.org/10.1088/1742-6596/75/1/012063, 2007. a
Vali, M., Petrović, V., Boersma, S., van Wingerden, J.-W., and Kühn, M.: Adjoint-based model predictive control of wind farms: Beyond the quasi steady-state power maximization, IFAC-PapersOnLine, 50, 4510–4515, 2017. a
Vali, M., Petrović, V., Boersma, S., van Wingerden, J.-W., Pao, L. Y., and Kühn, M.: Adjoint-based model predictive control for optimal energy extraction in waked wind farms, Control Eng. Pract., 84, 48–62, 2019a. a
Vali, M., Petrović, V., Steinfeld, G., Pao, L. Y., and Kühn, M.: An active power control approach for wake-induced load alleviation in a fully developed wind farm boundary layer, Wind Energ. Sci., 4, 139–161, https://doi.org/10.5194/wes-4-139-2019, 2019b. a, b, c, d
Vali, M., Petrović, V., Pao, L. Y., and Kühn, M.: Model Predictive Active Power Control for Optimal Structural Load Equalization in Waked Wind Farms, IEEE T. Control Syst. Technol., 30, 30–44, https://doi.org/10.1109/TCST.2021.3053776, 2022. a
Vallejo, D. and Chaer, R.: Mixture Density Networks applied to wind and photovoltaic power generation forecast, in: 2020 IEEE PES Transmission Distribution Conference and Exhibition – Latin America, 28 September–2 October 2020, Montevideo, Uruguay, 1–5, https://doi.org/10.1109/TDLA47668.2020.9326221, 2020. a
Van Den Broek, M. J. and van Wingerden, J.-W.: Dynamic Flow Modelling for Model-Predictive Wind Farm Control, J. Phys.: Conf. Ser., 1618, 022023, https://doi.org/10.1088/1742-6596/1618/2/022023, 2020. a
van der Hoek, D., Kanev, S., Allin, J., Bieniek, D., and Mittelmeier, N.: Effects of axial induction control on wind farm energy production – A field test, Renew. Energy, 140, 994–1003, https://doi.org/10.1016/j.renene.2019.03.117, 2019. a, b
van Der Hoek, D., Sinner, M., Simley, E., Pao, L., and van Wingerden, J.-W.: Estimation of the Ambient Wind Field From Wind Turbine Measurements Using Gaussian Process Regression, in: IEEE 2021 American Control Conference (ACC), 25–28 May 2021, New Orleans, LA, USA, 558–563, https://doi.org/10.23919/ACC50511.2021.9483088, 2021. a
van Dooren, M. F., Campagnolo, F., Sjöholm, M., Angelou, N., Mikkelsen, T., and Kühn, M.: Demonstration and uncertainty analysis of synchronised scanning lidar measurements of 2-D velocity fields in a boundary-layer wind tunnel, Wind Energ. Sci., 2, 329–341, https://doi.org/10.5194/wes-2-329-2017, 2017. a
van Wingerden, J. W., Hulskamp, A. W., Barlas, T., Marrant, B., van Kuik, G. A. M., Molenaar, D.-P., and Verhaegen, M.: On the proof of concept of a `Smart' wind turbine rotor blade for load alleviation, Wind Energy, 11, 265–280, https://doi.org/10.1002/we.264, 2008. a
van Wingerden, J.-W., Pao, L., Aho, J., and Fleming, P.: Active Power Control of Waked Wind Farms, IFAC-PapersOnLine, 50, 4484–4491, https://doi.org/10.1016/j.ifacol.2017.08.378, 2017. a
van Wingerden, J. W., Fleming, P. A., Göçmen, T., Eguinoa, I., Doekemeijer, B. M., Dykes, K., Lawson, M., Simley, E., King, J., Astrain, D., Iribas, M., Bottasso, C. L., Meyers, J., Raach, S., Kölle, K., and Giebel, G.: Expert Elicitation on Wind Farm Control, J. Phys.: Conf. Ser., 1618, 022025, https://doi.org/10.1088/1742-6596/1618/2/022025, 2020. a, b
Veers, P., Dykes, K., Lantz, E., Barth, S., Bottasso, C., Carlson, O., Clifton, A., Green, J., Green, P., Holttinen, H., Laird, D., Lehtomäki, V., Lundquist, J., Manwell, J., Marquis, M., Meneveau, C., Moriarty, P., Munduate, X., Muskulus, M., Naughton, J., Pao, L., Paquette, J., Peinke, J., Robertson, A., Sanz Rodrigo, J., Sempreviva, A. M., Smith, J. C., Tuohy, A., and Wiser, R.: Grand challenges in the science of wind energy, Science, 366, 443, https://doi.org/10.1126/science.aau2027, 2019. a
Vermeulen, P. E. and Builtjes, P. J.: Mathematical modelling of wake interaction in wind turbine arrays. Part 1. Description and evaluation of the mathematical model, Tech. Rep. PB-84-164680, Netherlands Organization for Applied Scientific Research TNO, https://www.osti.gov/etdeweb/biblio/6770825 (last access: 17 November 2022), 1981. a
Viola, F., Iungo, G., Camarri, S., Porté-Agel, F., and Gallaire, F.: Prediction of the hub vortex instability in a wind turbine wake: stability analysis with eddy-viscosity models calibrated on wind tunnel data, J. Fluid Mech., 750, R1, https://doi.org/10.1017/jfm.2014.263, 2014. a, b
Vitsas, A. and Meyers, J.: Multiscale aeroelastic simulations of large wind farms in the atmospheric boundary layer, J. Phys.: Conf. Ser., 753, 082020, https://doi.org/10.1088/1742-6596/753/8/082020, 2016. a
Wang, C., Wang, J., Campagnolo, F., Carreon, D., and Bottasso, C. L.: Validation of large-eddy simulation of scaled waked wind turbines in different yaw misalignment conditions, J. Phys.: Conf. Ser., 1037, 062007, https://doi.org/10.1088/1742-6596/1037/6/062007, 2018. a
Wang, C., Campagnolo, F., and Bottasso, C. L.: Does the use of load-reducing IPC on a wake-steering turbine affect wake behavior?, J. Phys.: Conf. Ser., 1618, 022035, https://doi.org/10.1088/1742-6596/1618/2/022035, 2020a. a
Wang, C., Campagnolo, F., and Bottasso, C. L.: Identification of airfoil polars from uncertain experimental measurements, Wind Energ. Sci., 5, 1537–1550, https://doi.org/10.5194/wes-5-1537-2020, 2020b. a
Wang, C., Campagnolo, F., Sharma, A., and Bottasso, C. L.: Effects of dynamic induction control on power and loads, by LES-ALM simulations and wind tunnel experiments, J. of Phys.: Conf. Ser., 1618, 022036, https://doi.org/10.1088/1742-6596/1618/2/022036, 2020c. a, b, c
Wang, C., Campagnolo, F., Canet, H., Barreiro, D. J., and Bottasso, C. L.: How realistic are the wakes of scaled wind turbine models?, Wind Energ. Sci., 6, 961–981, https://doi.org/10.5194/wes-6-961-2021, 2021. a
Wang, J., Foley, S., Nanos, E. M., Yu, T., Campagnolo, F., Bottasso, C. L., Zanotti, A., and Croce, A.: Numerical and Experimental Study of Wake Redirection Techniques in a Boundary Layer Wind Tunnel, J. Phys.: Conf. Ser., 854, 012048, https://doi.org/10.1088/1742-6596/854/1/012048, 2017. a, b
Wang, J., Wang, C., Campagnolo, F., and Bottasso, C. L.: Wake behavior and control: comparison of LES simulations and wind tunnel measurements, Wind Energ. Sci., 4, 71–88, https://doi.org/10.5194/wes-4-71-2019, 2019. a, b
WindEEE: WindEEE Web Site, https://www.eng.uwo.ca/windeee/index.html (last access: 18 November 2022), 2020. a
Winslow, J., Otsuka, H., Govidarajan, B., and Chopra, I.: Basic understanding of airfoil characteristics at low Reynolds numbers (104–105), J. Aircraft, 55, 1050–1061, https://doi.org/10.2514/1.C034415, 2018. a
Wise, A. S. and Bachynski, E. E.: Wake meandering effects on floating wind turbines, Wind Energy, 23, 1266–1285, https://doi.org/10.1002/we.2485, 2020. a
Witha, B., Steinfeld, G., and Heinemann, D.: High-Resolution Offshore Wake Simulations with the LES Model PALM, in: Wind Energy – Impact of Turbulence, edited by: Hölling, M., Peinke, J., and Ivanell, S., Springer, Berlin, Heidelberg, 175–181, https://doi.org/10.1007/978-3-642-54696-9_26, 2014. a
Wu, L. K. and Porté-Agel, F.: Flow Adjustment Inside and Around Large Finite-Size Wind Farms, Energies, 10, 2164, https://doi.org/10.3390/en10122164, 2017. a
Wu, X.: Inflow Turbulence Generation Methods, Annu. Rev. Fluid Mech., 49, 23–49, https://doi.org/10.1146/annurev-fluid-010816-060322, 2017. a
Wu, Y.-T. and Porté-Agel, F.: Simulation of Turbulent Flow Inside and Above Wind Farms: Model Validation and Layout Effects, Bound.-Lay. Meteorol., 146, 181–205, https://doi.org/10.1007/s10546-012-9757-y, 2013. a
Yang, Z., Li, Y., and Seem, J. E.: Multi-model predictive control for wind turbine operation under meandering wake of upstream turbines, Control Eng. Pract., 45, 37–45, https://doi.org/10.1016/j.conengprac.2015.08.009, 2015. a
Yang, Z., Sarkar, P., and Hu, H.: An Experimental Investigation on the Aeromechanic Performance and Wake Characteristics of a Wind Turbine Model Subjected to Pitch Motions, in: 29th AIAA Applied Aerodynamics Conference, AIAA paper 2016-1997, 4–8 January 2016, San Diego, California, USA, https://doi.org/10.2514/6.2016-1997, 2016. a
Yılmaz, A. E. and Meyers, J.: Optimal dynamic induction control of a pair of inline wind turbines, Phy. Fluids, 30, 085106, https://doi.org/10.1063/1.5038600, 2018. a, b
Zasso, A., Giappino, S., Muggiasca, S., and Rosa, L.: Optimization of the boundary layer characteristics simulated at Politecnico di Milano Boundary Layer Wind Tunnel in a wide scale ratio range, in: Proceedings of the 6th Asia-Pacific Conference on Wind Engineering, 12–14 September 2005, Seoul, Korea, 708–723, ISBN 8989693152, ISBN 9788989693154, 2005. a
Zhang, H., Liu, Y., Yan, J., Han, S., Li, L., and Long, Q.: Improved Deep Mixture Density Network for Regional Wind Power Probabilistic Forecasting, IEEE T. Power Syst., 35, 2549–2560, https://doi.org/10.1109/TPWRS.2020.2971607, 2020. a
Zhao, H., Zhao, J., Qiu, J., Liang, G., and Dong, Z. Y.: Cooperative Wind Farm Control With Deep Reinforcement Learning and Knowledge-Assisted Learning, IEEE T. Indust. Inform., 16, 6912–6921, https://doi.org/10.1109/TII.2020.2974037, 2020. a
Zhu, W.: Models for wind tunnel tests based on additive manufacturing technology, Progr. Aerosp. Sci., 110, 100541, https://doi.org/10.1016/j.paerosci.2019.05.001, 2019. a
- Abstract
- Introduction
- WFFC physics: turbulence, wakes, and the atmospheric boundary layer
- Control algorithms
- Validation and industrial implementation
- Integrated design and systems perspective
- Conclusions
- Appendix A
- Data availability
- Author contributions
- Competing interests
- Disclaimer
- Acknowledgements
- Financial support
- Review statement
- References
- Article
(5817 KB) - Full-text XML
- Abstract
- Introduction
- WFFC physics: turbulence, wakes, and the atmospheric boundary layer
- Control algorithms
- Validation and industrial implementation
- Integrated design and systems perspective
- Conclusions
- Appendix A
- Data availability
- Author contributions
- Competing interests
- Disclaimer
- Acknowledgements
- Financial support
- Review statement
- References