the Creative Commons Attribution 4.0 License.
the Creative Commons Attribution 4.0 License.
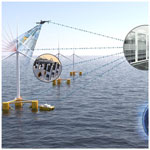
Grand challenges in the digitalisation of wind energy
Andrew Clifton
Sarah Barber
Andrew Bray
Peter Enevoldsen
Jason Fields
Anna Maria Sempreviva
Lindy Williams
Julian Quick
Mike Purdue
Philip Totaro
Yu Ding
The availability of large amounts of data is starting to impact how the wind energy community works. From turbine design to plant layout, construction, commissioning, and maintenance and operations, new processes and business models are springing up. This is the process of digitalisation, and it promises improved efficiency and greater insight, ultimately leading to increased energy capture and significant savings for wind plant operators, thus reducing the levelised cost of energy. Digitalisation is also impacting research, where it is both easing and speeding up collaboration, as well as making research results more accessible. This is the basis for innovations that can be taken up by end users. But digitalisation faces barriers. This paper uses a literature survey and the results from an expert elicitation to identify three common industry-wide barriers to the digitalisation of wind energy. Comparison with other networked industries and past and ongoing initiatives to foster digitalisation show that these barriers can only be overcome by wide-reaching strategic efforts, and so we see these as “grand challenges” in the digitalisation of wind energy. They are, first, creating FAIR data frameworks; secondly, connecting people and data to foster innovation; and finally, enabling collaboration and competition between organisations. The grand challenges in the digitalisation of wind energy thus include a mix of technical, cultural, and business aspects that will need collaboration between businesses, academia, and government to solve. Working to mitigate them is the beginning of a dynamic process that will position wind energy as an essential part of a global clean energy future.
- Article
(2326 KB) - Full-text XML
- BibTeX
- EndNote
The global green transition is the process of changing our technological base and consumption patterns to reduce and ultimately reverse anthropogenic climate change. An integral part of the green transition is the energy transition, which is the change in energy generation and consumption away from fossil fuels towards renewable energy from sources such as wind energy, coupled with increased electrification (Jacobson, 2017).
1.1 What are “grand challenges”?
In order for wind to be one of the primary sources of electricity generation in 2050, Veers et al. (2019) argue in their paper, “Grand challenges in the science of wind energy”, that the wind energy sector must address three high-level issues, which they describe as grand challenges:
-
improved understanding of atmospheric and wind power plant flow physics;
-
aerodynamics, structural dynamics, and offshore wind hydrodynamics of enlarged wind turbines; and
-
systems science for the integration of wind power plants into the future electricity grid.
Several things are clear from the grand challenges paper and other discussions of the future direction for wind energy (e.g. van Kuik et al., 2016; Wood, 2020). First, there are also important challenges related to environmental and ecological impacts (Ross et al., 2020; Gauld et al., 2019), as well as social, “territorial and institutional dimensions” (Brannstrom et al., 2019). Second, the challenges cover multiple scales in space and time. Third, they are interrelated and cannot easily be separated, and thus addressing them will need an interdisciplinary research and development (R & D) approach. These points suggest in turn that combining, using, and sharing multiscale and interdisciplinary data will be an essential, cross-cutting capability to enable the transition to a renewable energy system.
Other industries have already recognised the considerable potential for the opportunities for the use of data; as the authors of the U.S. Chamber of Commerce Foundation research report The Future of Data-Driven Innovation noted in 2014,
Regardless of what form it takes, data tells a story. It can identify cost savings and efficiencies, new connections and opportunities, and an improved understanding of the past to shape a better future. It also provides the details necessary to allow us to make more informed decisions about the next step we want to take. (Heinz et al., 2014)
1.2 Using data to enable the green energy transition
The effective use of data is foreseen as a lever for the global green transition through empowering information and communication technologies to increase resource efficiency and to accelerate research and development (Fernández-Portillo et al., 2019; Castro et al., 2021). We consider the effective use of data to be a key characteristic and outcome of digitalisation, which we define as the organisational and sector-wide use of data and digital technologies to improve efficiency, create insights, and develop products and services. Digitalisation should not be confused with digitisation, which is the conversion of information into digital forms and thus one of several precursors to digitalisation.
This use of data is increasing in other sectors as well; the manufacturing sector has seen that significant business advantages can be obtained by combining automation and data exchange with manufacturing technologies, which is itself a form of digitalisation. This trend is known as “Industry 4.0” and follows previous sector-wide manufacturing industry transitions including the use of steam power, electrification, and the introduction of computing. There are reasons to expect similar trends in energy; in 2017, a report by the United Nations Industrial Development Organization (UNIDO) noted that
The sustainable energy transition and Industry 4.0 share important characteristics: both are highly influenced by technological innovations, dependent on the development of new suitable infrastructures and regulations as well as are potential enablers for new business models. (Nagasawa et al., 2017)
Historically, technological transitions like the renewable energy transition and digitalisation are driven by a combination of curiosity, need, and cultural pressures and occur when a disruptive technological innovation emerges from a niche (Geels, 2002). Strong connections between the niches and the broader landscape help to create a pull for new technologies that are relevant to users, helping increase adoption. Often the process of adoption is transformational for both the new technology and the market, and while some new innovations fail, others go on to become part of the technological landscape. The process of innovation therefore does not happen at the same time across a sector but is instead uneven and happens opportunistically and probably where the barriers are lowest. The more barriers can be removed or lowered, the higher the potential for innovation will be.
This paper discusses how the digitalisation of the wind energy industry could support the renewable energy transition, the barriers that exist to digitalisation, and how the wind energy industry itself must adapt to enable digitalisation. We explore the possibilities offered to the wind energy sector by the availability of continuously developing digital technologies – such as storage, connectivity, computational power, data management, data science tools, digital twins, and many others – to exploit the ever-increasing amount of data. These lead to opportunities for efficiency, innovation, and entrepreneurialism. Together, these are many of the essential aspects of digitalisation. Businesses, organisations, industry sectors, and societies undergo a digital transformation when they adopt digitalisation on a large scale, while digital businesses are those that are largely based on digital products and services. To help understand and differentiate between these similar terms, we provide an overview in Table 1.
(Rachinger et al., 2019)(Mergel et al., 2019)1.3 Scope of this paper
Our goal in this paper is to identify sector-level grand challenges in the digitalisation of wind energy. We consider these grand challenges to be things (1) that must be done for the wind energy sector to digitalise, (2) that impact many different stakeholders, and (3) that require coordinated effort to solve. They might also be issues that are seen again and again in different markets such that they are the same challenge but with different solutions. Identifying the grand challenges for digitalisation therefore requires combining an understanding of the process of digital transformation with many different stakeholder perspectives.
An initial literature review revealed that there is extensive research and development (R & D) taking place within the wind energy community along many avenues (see, for example, van Kuik et al., 2016, for an overview). This R & D often leads to the creation of algorithms or software that could have applications in industry or form the basis of digital businesses. There are many transferable digitalisation technologies available as part of the Industry 4.0 transition. But, we also found that few studies have examined the consequences, level, and trends of digitalisation in the wind energy industry. In response to this apparent lack of research, we report here the results of a literature survey and data collection activities to understand how digitalisation is progressing, how it might lead to fundamental changes in the wind energy industry, and the issues that would be faced en route. Understanding these issues is crucial for identifying the grand challenges.
We identified three grand challenges in the digitalisation of wind energy. These are related to data, culture, and coopetition. They include (1) the need to create a framework of findable, accessible, interoperable, and (re-)usable (FAIR) data; (2) the need to connect people to those data to foster innovation; and (3) the need to enable collaboration and competition between organisations.
This paper is targeted at policy advisers, funding agencies, research managers, and others involved with technology transfer at a strategic level. We expect that it will also be of interest to technologists and researchers, as it may provide insight into future research directions.
The next section provides a short introduction to the opportunities presented by the digitalisation of wind energy. Section 3 presents the results of an initial expert elicitation that informed this study and the identification of the grand challenges. Section 4 describes the process of digital transformation in the context of wind energy. Section 5 provides lessons learned from other high-technology networked industries. Based on this, we carry out a more specific expert interview series and use the results to identify the grand challenges for the digitalisation of wind energy in Sect. 6. Section 7 presents our conclusions.
The financial performance of a wind energy facility can be summarised using the levelised cost of energy (LCOE). This is the average cost to produce a unit of electrical energy over the lifespan of a wind plant. There has been a strong drive to reduce LCOE over recent years (Duffy et al., 2020), resulting in wind energy often being the cheapest new source of energy in many markets (IRENA, 2021). This trend is expected to continue (Wiser et al., 2021), which is essential if wind energy is to remain a significant source of energy in the future.
LCOE is driven by several factors including CAPEX (capital expenditures) and OPEX (operational expenditure), the cost of project financing, and the income from energy sales. Data can be used in many different ways to reduce the LCOE of a wind energy plant.
-
CAPEX is the capital cost of the wind turbines and foundations or substructures of a wind farm. Access to better site information, more accurate wind turbine models, and better wind farm simulation tools as a result of digitalisation therefore allows wind turbine and wind plant designs to be better adapted to the likely operating conditions, potentially saving on raw materials or increasing reliability. Similarly, digitalisation makes it easier to use data from existing plants to validate design tools and the design process.
-
OPEX includes both operational and maintenance expenses.
Operational costs arise through business management and technical management of the plant; data and digitalisation could help reduce these costs through improved (and cheaper) condition monitoring. Predictive maintenance based on data from condition monitoring systems can avoid unplanned maintenance, which reduces OPEX and increases energy production. These were high-priority applications in a 2020 survey reported in Berkhout et al. (2020).
Maintenance costs can be reduced by minimising the need for maintenance and by reducing the business impact and cost of those actions. Wear and tear – which require maintenance – can be reduced by improved turbine design and by minimising loads through controls that use turbine sensors (Pettas et al., 2018) or integrate external information from, for example, wind lidar (Schlipf et al., 2018). Digital twins driven by sensor data can be used to quantify the remaining lifetime and inform control actions or maintenance decisions (Branlard et al., 2020; Pettas et al., 2018; Rinker et al., 2018). Maintenance can also be made easier or more effective through the use of virtual reality (VR) for maintenance planning, augmented reality (AR) to help technicians identify equipment, online manuals accessed using speech-recognition systems, inspection by unmanned aerial vehicles (UAVs), or the use of robots to carry out repairs, as well as many other tools (see, for example, Kapp et al., 2022; Eggert et al., 2020).
-
Digitalisation can increase the amount of energy generated by a turbine by detecting losses due to blade soiling, icing, damage, or erosion and allowing the operator to clean the blades, start de-icing systems, or carry out repairs. It can enable wind-plant-level control strategies that reduce wake interaction and thus reduce energy losses (Fleming et al., 2017; Simley et al., 2023).
Access to wind data and market data – another aspect of digitalisation – can be used when planning regional and national transmission and distribution to help avoid supply shortages or curtailment (Nycander et al., 2020), while automation can reduce curtailment compared to manual processes (Bird et al., 2014).
-
Another is value of energy: in some markets it may be possible to make more money from wind energy by selling it when market prices are high. Digitalisation can enable this by providing market simulations, through decision support tools for energy trading, or by informing energy storage strategies. Improved weather and demand forecasts – made possible by more data and digital technologies – are already being used by grid operators and energy suppliers to reduce imbalance charges and penalties, but the value of this depends on market structures (see, for example, González-Aparicio and Zucker, 2015). Weather forecasts can also be improved by data sharing by multiple organisations in a region (Wilczak et al., 2015).
-
Financing costs for a new wind plant are a function of many factors including the predicted uncertainty of energy production and the historical industry-wide accuracy of energy predictions. Reducing either uncertainty can reduce the cost of financing. This requires developers and their engineers to demonstrate the accuracy of their energy yield assessment processes, which can be done through internal or public benchmarking exercises, whereby energy yield assessments are repeated for old development sites and compared against actual production data (Fields et al., 2021). Digitalisation enables this to be done more easily and faster within an organisation, or across a whole sector.
A future wind plant that benefits from digitalisation is visualised in Fig. 1. In this plant, many different organisations collaborated during the design phase to plan a facility that was optimised both for the operating conditions and grid conditions, reducing CAPEX and OPEX. Whilst in operation, the plant operator is able to make informed decisions about energy production and plant maintenance while accounting for market conditions and environmental or societal constraints. They can also share data and decisions across organisational boundaries with the many parties involved. This also reduces OPEX while maximising the income from energy production, increasing worker safety, and reducing the environmental impact.
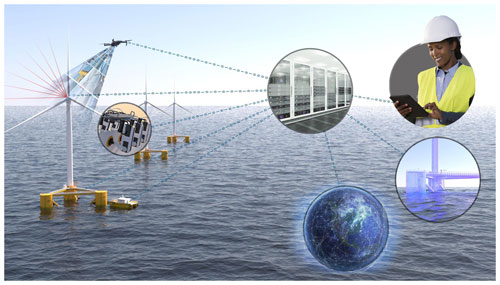
Figure 1Digitalisation in action. In this future floating wind energy plant, digitalisation enables a plant manager to take data-based decisions in real time, increasing safety and reducing the cost of energy. Image credit: NREL graphics team.
The LCOE of wind energy can also be impacted through other related activities such as academic research, by developing ports and transport infrastructure, by advances in manufacturing, by workforce development, and through other activities. Digitalisation in those areas could thus help reduce the LCOE of wind energy.
In researching this paper we found that it was very difficult to quantify the potential value of digitalisation and its contribution to LCOE. There are very few clear value statements available, and what statements exist, they are hard to map from one use case to another as CAPEX, OPEX, and other terms in the LCOE equation are very wind farm specific. Digitalisation is also an enabler of other services, and thus its value is realised through those other services. One example of this is wake steering in operational wind farms, which requires data from across the wind farm to be collected, analysed, and acted upon. Recent analysis suggests its adoption could release an additional 0.5 % to 2 % in annual energy production (AEP) in US wind farms (Simley et al., 2023).
Cost models have been developed for OPEX (Koukoura et al., 2019) that could be used to estimate the impact of certain digitalisation scenarios, but we lack the data to interpret these types of cost models across the entire wind energy industry. We note though that, as a whole, digitalisation will be one of the enabling factors for the expected continued reduction in LCOE through 2050, which Wiser et al. (2021) summarise as “across all wind applications, LCOE is anticipated to decline by 17–35 % in 2035 and 37–49 % in 2050 [relative to 2019]”, with the majority of the reduction in LCOE (5 %–15 %) due to improvements in CAPEX and turbine capacity factor, depending on whether the turbine is built on land or offshore. Reductions in OPEX were the third largest contributor to LCOE reductions by 2050 but might be possible to realise more immediately rather than needing to be designed in a new plant. They could have a significant impact on a wind turbine's (financial) performance. For example, one vendor of predictive maintenance using data and machine learning claims that
Using [brand name of a digital service], one can expect a reduction in replacement and labour costs. Assuming a 20 % reduction in the repair and maintenance portion of O & M [operations and maintenance] costs, this would translate into annual cost savings of $ 11 383 for a 2.5-MW turbine and $ 34 148 for a 7.5-MW turbine. (SKF, 2021)
Over 25 years this offers approximately USD 285 000 to 854 000 in savings. But not all digital services achieve these savings: a wind energy industry market research company interviewed for this paper noted that
… an average reduction of 11 % of the operations and maintenance cost for operational assets when utilising predictive maintenance facilitated by data analytics versus conventional reactive maintenance practices.
It is also not clear how much these services cost, so the net savings are unknown. Without a clear understanding of the costs and benefits, adoption by customers will most likely be slow, sporadic, and limited (Wang et al., 2022; Naeher, 2022).
From the examples above it can also be seen that digitalisation in the wind energy sector is progressing but tends to be vertical or siloed within an organisation or takes place directly between one organisation and another. Steps have been taken to initiate collaboration across organisational boundaries, for example by sharing (some) research data on online platforms, but very little operational data are freely available, and the wind energy industry acknowledges its own difficulties in sharing data (e.g. Lee et al., 2020). This internal focus is not unusual during the adoption of digitalisation; Branca et al. (2020) found that initial digitalisation efforts in the metals processing industry were often around optimising internal processes, manufacturing, or products instead of across organisations' boundaries.
The wind energy industry is highly interconnected both physically and in terms of the interdisciplinary science and engineering that underpins it. This, in combination with the innovation style of the leading industry actors (Sovacool and Enevoldsen, 2015), suggests that collaboration may become a more important aspect of the digitalisation of wind energy in the future. Advances in other collaboration-related technologies such as communications and cyber security are therefore likely to be vital to enabling digitalisation in the wind sector, but this need is not unique to the wind energy sector.
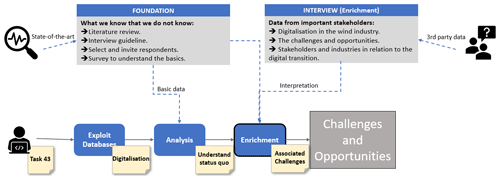
Figure 2Flowchart showing how data collection, processing, and analysis were used to help identify the grand challenges.
These examples also show that the barriers to new business models are often not scientific or technical – because the underlying technologies generally already exist – but might be related to reluctance to share data, concerns around competition, commercial interest, or the market they operate in. If the return on investment is unclear, the decision to initiate a digital transformation within an organisation might be deferred until more information is available.
The examples also introduce some of the important stakeholders in the digitalisation of a commercial wind energy facility, which include investors, project managers, technologists, site workers, and many others. Research projects may have different stakeholders, such as researchers and funding agencies. Each stakeholder group has their own perspectives, which raises the possibility that digitalisation is potentially as much a cultural challenge as it is a technical challenge.
The grand challenges in the science of wind energy were identified through a process of expert elicitation and synthesis (Veers et al., 2019; Dykes et al., 2019). In this paper, we follow a similar process, shown in Fig. 2. First, a literature survey coupled with expert elicitations was used to understand the current state of digitalisation in the wind energy sector and actors' perceived challenges. We then refined some of this information through further interviews. The results of the expert elicitation are presented in this section, while the results of the literature survey are presented in context in Sects. 4 and 5. This information is combined in Sect. 6 to identify the grand challenges in the digitalisation of wind energy.
3.1 Data collection
The data presented and applied in this study rely on an empirical collection via a survey and semi-structured interviews with digitalisation experts, innovation frontrunners, and energy industry market leaders. Other community surveys have also been carried out recently, and their published results have been considered when preparing this document. For example, in 2019, a survey was carried out to explore the expectations and priorities of wind energy operations and maintenance experts (Berkhout et al., 2020). The data collection and subsequent processing and analysis followed the process exemplified in Fig. 2.
3.2 Survey
Members of the International Energy Agency (IEA) Wind Task 43 and the wind energy industry were surveyed to determine trends of digitalisation in wind energy. The 102 respondents provided 68 different open answers to 4 questions, which were analysed and used as background knowledge in this study. A specific emphasis was targeted towards critical trends, where data management and data sharing were found to be the most critical trends in digitalisation today, while smart energy and robotics were listed as the topics of the future. The output is interesting, as respondents decided to focus on the prerequisite of digital activities as the critical trend of today before diving into actual digitalisation activities.
3.3 Expert interviews
In parallel to the survey, semi-structured interviews were conducted with 44 digitalisation experts mainly from the wind energy sector. The respondents were not involved in the initial survey. The interviews followed a guideline based on the initial literature review and IEA Wind Task 43's activities. They were organised to gather insights from a broad range of wind energy life cycle experts and digitalisation technology experts. The Task 43 team interviewed experts in many wind energy life cycle areas, including turbine design and manufacturing, development, construction, operations, and maintenance, as well as life assessment and decommissioning. Several technology area experts in data collection, data management, data analytics, and automation were also interviewed (Fig. 3).
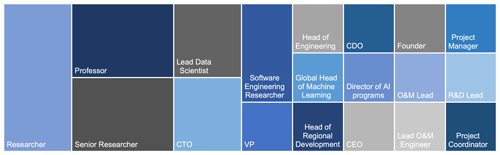
Figure 3The interviewees' job titles. IEA Wind Task 43 interviewed 44 experts about wind energy digitalisation to help identify the grand challenges. The experts come from across the wind energy industry and have a wide range of roles, as evidenced by the many different job titles that they use. The size of the blocks shows the relative frequency of each title. Colours are arbitrary.
Beyond providing a general understanding of digitalisation efforts in the wind energy sector, we categorised the output of the interviews into separate challenges (Fig. 4) and opportunities (Fig. 5).
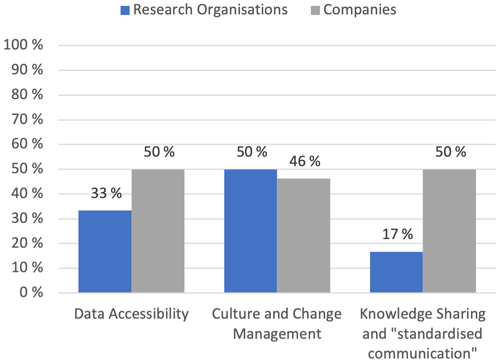
Figure 4The top three digitalisation challenges in the wind energy sector mentioned during the interviews. The result is presented and analysed by stakeholders in academia, including research organisations, and in the private sector, respectively.
Figure 4 indicates that companies perceive data accessibility and knowledge sharing as greater challenges than culture and change management, when compared to research organisations. Nevertheless, consensus was established among the two stakeholder groups on the three dominant challenges.
Figure 5 highlights that the two stakeholder groups also consider data accessibility an opportunity. Model development and “communication and open source” were also considered opportunities; research organisations see the greatest potential in the latter.
The interview outputs were analysed and divided into industry and research-focused stakeholder groups, which again were represented by eight companies and five research institutions. Table 2 explains the top challenges and opportunities.
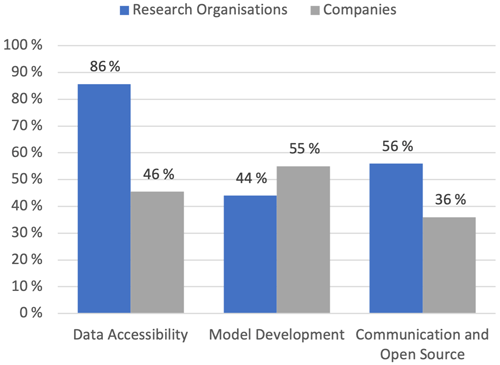
Figure 5The top three digitalisation opportunities in the wind energy sector mentioned during the interviews for different types of organisations.
The identified challenges will be analysed following a retroductive approach in the following sections where the requirements, process, and opportunities related to the digital transformation of the wind energy industry will be discussed based on the output from the survey and interviews. Then, the outlook on digital opportunities will be analysed in relation to the digitalisation experienced in other industries. The empirical data collection, literature review, and analyses then identify the grand challenges in the digitalisation of the wind industry.
The survey data and interview findings described in the previous sections led us to further investigate the meaning of a digital transformation. Establishing what is meant by a digital transformation provides a framework for how digitalisation can unfold, both making use of and taking advantage of digitised data and novel data science tools. This transition leads to new products, processes, and business models (Fig. 6), transforming the technological landscape.
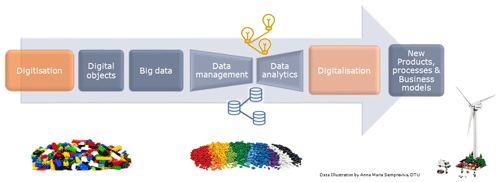
Figure 6Digital transformation is a process that goes from digitisation to digitalisation. Based on Sempreviva (2020).
In this section, we consider each of the components of the digital transformation. We describe some of the benefits and challenges associated with each of them and where we see a bottleneck in the process. Finally, we summarise the key aspects of the digital transformation most relevant for the wind energy industry, which are used towards defining the grand challenges in Sect. 6.
4.1 Digitisation: converting information from analogue to digital
Digitisation is the process of converting information into digital signals (Rachinger et al., 2019). The aim of digitisation is to provide the raw material for later use in digital business, and so it is important to capture as much information as possible in digital formats. Examples of digitisation in the wind energy industry include
-
converting analogue sensor signals from turbines or infrastructure into digital form,
-
converting written records such as maintenance logbooks into digital records,
-
formalising decision-making processes as algorithms and recording how individual decisions are made,
-
capturing business processes in software.
Sensor information from hardware in the wind energy system will be fundamental for releasing the opportunities of digitalisation. Wind turbines and other hardware in the wind energy system are extensively instrumented, and the data are brought together in data systems (often known as supervisory control and data acquisition systems or SCADA systems). This simplifies the process of data access. However, turbines are usually only instrumented with the sensors necessary to support the manufacturer's and operators' goals, which might be fewer than are required for other applications. This helps reduce costs of manufacturing and sensor maintenance. Also, although extensive databases are in place at most wind farms to connect wind turbines and the plant controller, security limitations often mean that it is impossible to connect other sensors to these networks and that it might only be possible to access sensor data through the turbine's own SCADA system.
The lack of access to turbine data limits the development of software-based third-party solutions, while the lack of data connections means that third-party solution providers often have to develop both the sensor and the data transmission tools, raising costs further. To avoid a situation in which multiple entirely new networks are built on top of each other in future wind farms, it would be helpful for wind farm developers and their engineers to plan for a high-bandwidth open network in new wind farms, with strong security implemented at the hardware level (Ahmed and Kim, 2014).
Even with access to SCADA data, challenges remain in extracting information from the data. SCADA data semantics are particularly complex, and it may be necessary to develop an ontology (Sect. 4.4) in order to understand data from each different turbine model. This ontology could in turn be leveraged to form a schema to enable data exchange.
For all that wind energy uses wind for its fuel, there is a relative lack of information about the wind fields in and around wind farms. This may change through the deployment of remote sensing by lidar, sodar, and radar or mobile in situ sensing by drones (Fig. 1). The extra costs of such devices will have to be balanced by cost savings such as reduced turbine fatigue or increased income in flexible energy markets where an operator can make an informed cost-benefit decision (Fig. 7). As remote sensing and mobile sensors have not yet become standard equipment on wind turbines or at wind farms, there is evidently still work to be done to justify their integration.
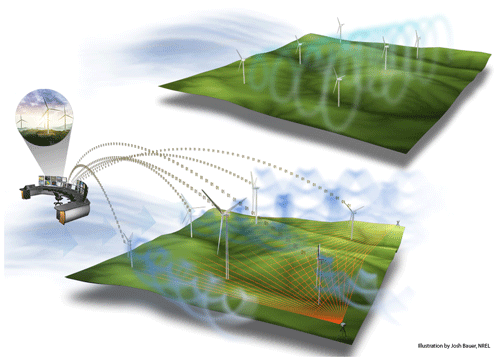
Figure 7Combining data from sensors across all parts of the wind energy system will be key to transitioning from today's isolated assets (top panel) to the interconnected wind plant of the future (bottom panel). Illustration by Josh Bauer, NREL from NREL/TP-5000-68123 (Dykes et al., 2017). Used with permission.
The wind energy sector is transitioning to digital, but the process is far from complete. The digitisation of data, knowledge, and tools is producing big data in the sector. However, creating the right environment for innovation requires further digital technologies. Wind energy requires information science to keep datasets retrievable by agreeing on and creating sets of general and domain-specific metadata (i.e. information about data) with related controlled vocabularies. Additionally, it needs data engineering to create and implement digital infrastructures for storing and analysing data, as well as creating and connecting infrastructures for data and governance to regulate data sharing and collaboration to deal with sensitive and proprietary data. All of the above actions in turn require money and time to convince stakeholders of the value of the investment in digital technologies; without a clear business case or plan for using digitised data as part of the digitalisation process, the perception may be that the process is expensive and might be met with resistance. While researchers have shown potential benefits from digitalisation, such as reducing fatigue loads or actuator use, they often cannot convert these into meaningful fiscal benefits. This step will require further collaboration between the research community, wind turbine original equipment manufacturers (OEMs), plant operators, and others to develop baseline cost models that could be used for such studies.
4.2 Digital objects
Digitalisation requires that people or machines can find the data created by digitisation and then make sense of them. Digital data alone (i.e. raw binary records) become usable through the addition of human- and machine-readable metadata that describe and package the data and place them in context, which turns data into discrete “digital objects” (Schwardmann, 2020).
Digital objects can be created from many different data sources. As well as turbine sensor readings, they might include research reports, software models or tools, algorithms, and many other things (Sect. 4.1). But these digital objects alone cannot be used effectively as part of a process. Instead, they need to be made findable, accessible, interoperable, and reusable (FAIR; Wilkinson et al., 2016).
-
Findable means that data can be discovered by people or machines through a search engine. Data can be made findable by identifying the data through the addition of metadata (a list of terms) which follow a defined schema. The metadata can then be aggregated for a dataset, and users can search through it to find the specific data they require. The process of making data findable adds time and cost to the creation of data, but if data are not findable, they will never have the chance to generate value.
-
Once the data have been made findable, they need to be made accessible. This means that the data should be retrievable using secure but open and free protocols, for example through the internet.
-
It is rare that one piece of data is sufficient. Therefore, data need to be interoperable so that they can be used in a workflow or applications. In practice, this means the need for machine-readable data and relationships.
-
To maximise the benefit of sharing data and digitising processes, data should be reusable so that they can be applied to different settings. Reusability by anyone besides the original creator requires that data be findable, accessible, and interoperable.
Making data FAIR enables their effective use as part of a process and makes it easier for a digital business to obtain and use the data they require and is thus an important step in digitalisation. For example, the creation of digital objects makes it feasible to implement large “data lakes” of all kinds of data in multiple formats instead of rigid warehouses or structured relational databases (Mathis, 2017). In data lakes, digital objects can be added to the lake with appropriate metadata and then retrieved as required. Indexing services offer the potential to create distributed data lakes that cross organisational boundaries, for example for research data so that users can access data from an ad hoc mix of sources (e.g. for the New European Wind Atlas; Hahmann et al., 2020; Dörenkämper et al., 2020). The use of data lakes reduces the overhead required for maintaining a data structure, such as weather observations organised by year, month, and day. In some cases, the transition away from organised or structured data to data lakes can be challenging. It can be perceived as risky, as unstructured data can become hard for humans to find and rely instead on software to be accessed. Although this risk can be mitigated through software testing, user training, and other measures, the perception of this risk is one example of the non-technical challenges that are faced in digitalisation.
Making FAIR domain data involves a range of challenges, including (i) gaining agreement among large communities on a common metadata schema; (ii) determining common terms for controlled vocabularies/taxonomies, incentivising data accessibility; and (iii) developing infrastructures for storing, analysing, and curating data. The European Commission has supported the use of FAIR principles by researchers through initiatives such as assessing research funding applicants on their plans for open data access and indirectly funding the development of sector-specific taxonomies. During the European FP7 Projects Integrated Research Programme on Wind Energy (IRPWind), a metadata schema and related taxonomies for wind energy were created, resulting in the development of a proof-of-concept for a metadata management system and registry based on semantics (see Sect. 4.3) (Sempreviva et al., 2017). The goal of IRPWind was to promote collaboration, standardisation, harmonisation, and knowledge transfer within the project while ensuring that the data produced complied with FAIR principles. The involvement of major organisations in wind energy in Europe associated with the European Energy Research Alliance Joint Programme on Wind Energy (EERA JPWind) further ensured the quality of work done by expert elicitation, enabled dissemination, and increased acceptance of the results. IRPWind helped advance the adoption of FAIR data principles in the wind energy sector (Schwanitz et al., 2022; Michiorri et al., 2022).
FAIR data are an enabler of open science. Open science is the shift to collaborative scientific development based on transparent and accessible knowledge (Sempreviva et al., 2019), which has recently gathered pace. Making data FAIR is a prerequisite for openness as it follows the principle that “data should be as open as possible, as closed as necessary” (the H2020 Program's Guidelines on FAIR Data; European Commission, 2016).
The official adoption of open science for “Horizon Europe” projects in 2018 will thus likely lead to more awareness and adoption of the FAIR principles. In addition, the United States Department of Energy issued a funding opportunity announcement (FOA) in 2020 for artificial intelligence frameworks (including data) that utilise the FAIR guidelines and principles:
The DOE SC programme in Advanced Scientific Computing Research (ASCR) hereby announces its interest in making research data and artificial intelligence (AI) models findable, accessible, interoperable, and reusable (FAIR) to facilitate the development of new AI applications in SC's congressionally authorised mission space, which includes the advancement of AI research and development. In particular, ASCR is interested in supporting FAIR benchmark data for AI; and FAIR frameworks for relating data and AI models. (From FOA DE-FOA-0002306, US Department Of Energy, 2020)
4.3 Big data
Digitisation will change the characteristics of the data available to people and organisations. Data volumes increase – hence “big data” – the data are moving or changing at higher velocity, the data are only useful if they can be given value, the data come in many different varieties, and the veracity of the data needs to be checked and ensured. These challenges are known as the “five V's” of big data for the volume, velocity, value, variety, and veracity of data (Ishwarappa and Anuradha, 2015). Practically, data become “big” when they cannot be handled using existing approaches. For example, when data volumes exceed storage capacities on a typical desktop computer, they require specialised storage and analysis tools. As a result, what is considered big data is not fixed but changes depending on the user as storage gets cheaper, computing power increases, or new software is developed.
Although it brings challenges, such a level of data availability also brings advantages, for example the ability to aggregate information across a fleet of wind turbines, or to look at an entire wind plant project from the first idea through to decommissioning. Furthermore, this ability to see the whole picture allows organisations to identify, explore, and act upon hidden relationships when appropriate data management and analytics tools are available.
Many tools have already been developed to help users deal with big data. One way to cope with big data is to store them together with metadata to enable their management. Cloud-based computing allows users to manipulate much larger amounts of data than can be processed on a desktop computer, while dedicated search tools allow the data to be queried. Similarly, visualisation can help in understanding big data (Bush et al., 2017).
Another challenge with big data is the need for organisational IT services that span physical infrastructure and cloud-based or remote infrastructure. This can bring pricing uncertainty and also increases an organisation's attack surface, and further, it can require very specialised knowledge. An alternative is to devolve responsibility to individual users, but this can rapidly result in a patchwork of independent solutions.
Taking cues from the tech sector (e.g. LinkedIn, Google), we expect that metadata will be an increasingly important aspect of big data analytics. A database noting previously useful connections and verification studies, as well as framing a general knowledge map, will likely assist in developing next-generation analysis approaches. For example, a knowledge map could assist a large owner in recognising and connecting maintenance data from a diversity of contractors. This is known as the “open-world” assumption, where it is not assumed that all data will be acquired in a standard form.
The issues and challenges of big data are generally well-known, and solutions exist. The next step in digitalisation is then to make data available to the right users to provide insight and apply outcomes. This requires effective data management and data analytics.
4.4 Data management: right data, right user, right time
A digital workflow set-up for a problem solving process requires access to data, such as sensor data, data processing, or simulation codes, or other types of digital information. Data management policies describe how those data are collected, stored, and preserved, as well as who can access what data. Data management is therefore required to get the right data to the right users within an organisation and to manage data sharing across organisations. It is one of the steps for making data FAIR, as discussed in Sect. 4.2.
Data management is the enabler for data discovery and data sharing. Data discovery is the process of finding the appropriate data in a data storage. Although this can be done by querying the data directly, it is easier to search for data when they are tagged with the required metadata (Lund and Sempreviva, 2019). Ideally, metadata would follow a standard schema so that all data can be queried at once. The schema would ideally be populated using terms from a controlled vocabulary (e.g. a sensor might measure a physical property that is one of “wind speed”, “air temperature”, or “air relative humidity”) rather than letting the user choose freely (Lund and Sempreviva, 2019). This approach has been used in the recent IEA Wind Task 43 Wind Resource Assessment Data Model (Holleran et al., 2022), as well as the IEA Wind Task 43 Glossary (IEA Wind Task 43, 2022b). The IRPWind project introduced in Sect. 4.2 produced a metadata schema, as well as the design and demonstration of a metadata catalogue called ShareWind. The metadata schema includes the list of general Dublin Core metadata, as well as wind energy domain-specific metadata, populated using terms from related controlled vocabularies and taxonomies.
These controlled vocabularies can have whatever structure is required. For example, they could just be a list of terms, or could have a hierarchical structure that also implicitly includes relations between terms; these are known as taxonomies. They could also include relationships between vocabularies which are known as ontologies. Describing data by tagging it using these ontologies creates so-called semantic data models, which enables data discovery. Some examples include the ISO 81346 reference designation system for power plants (RDS-PP; ISO, 2022), as well as the IRPWind registry prototype (was hosted at Sharewind.eu, but the website is no longer active) which catalogued data using a metadata schema and related taxonomies or even ontologies based on expert elicitation (Sempreviva et al., 2017).
Different domains may have their own ontologies and thus use different approaches to naming a variable depending on historical reasons and the importance of the variable in each field. As an example, the wind energy community might describe a physical property measured by a thermometer simply as “air temperature”, while the meteorological community might tag the same data using the class “atmosphere” with a sequence of children (e.g. “dry bulb temperature” and “wet bulb temperature”). Such varied classifications prevent the easy exchange of digital objects across domains. Avoiding this discrepancy across domains is difficult, but it can be mitigated by making equivalences between terms, making the ontologies public and visible, and leveraging existing ontologies rather than developing new ones.
Following data discovery, sharing data is required if the data are to be used in a productive way by the organisation. Sharing can take many forms, including sharing data within a single team in an organisation to work on a problem together; sharing the data with different teams or units within an organisation to benefit from the different knowledge and capabilities of different teams; sharing the data with partner organisations to get the most out of the data; sharing the data with clients; sharing the data with specific researchers within a project to work on R & D projects together; or even sharing the data with the wider community in an open innovation process. Data sharing can be made easier by using common metadata schema across organisations and by developing data portal(s) that can query metadata catalogues at multiple organisations.
Willingness to share data is often linked to its perceived commercial importance. Commercially sensitive data might only be shared with a small group and need to be protected and will almost never be shared outside of an organisation; this is usually known as closed data. Some data might be made available to a small group outside the organisation or more broadly but under specific licence conditions. These are known as shared data. Other data might be freely accessible to anyone without restrictions; these are open data. Note that open data are distinct from FAIR data and that closed data should still be made FAIR for access within an organisation. There is an emergent need for a spectrum of open data licensing approaches especially for artificial intelligence and benchmarking applications (Benjamin et al., 2019; Maksimov and Fricker, 2020)
Sharing data or making it openly available would be a change from today's protected data and would require a new mindset, which could be encouraged by providing examples of the benefits of data sharing and its safety. One potential approach to get the wind energy sector used to sharing is to map and link databases that are distributed within and over different organisations. Each organisation then compiles and exposes a metadata catalogue of the data available for sharing. Then, a web crawler harvests the metadata and updates a central catalogue that is searchable by the community members. This approach has been proposed by the EERA JPWind community using the data registry ShareWind to compile a metadata catalogue; datasets are searchable and discoverable but not directly accessible, and data owners can register their data in a catalogue and tag it using terms from joint vocabularies. This allows data owners to maintain control over data access without having to transfer the data. The use of tags also allows for filtered searches that single out the most suitable dataset.
Overall, this solution improves the visibility and findability of state-of-the-art tools and data that can support the digitalisation process towards innovative solutions. Data are housed at the premises of the owners who are responsible for protecting the data and can then make them available to approved users. In 2021, the EU H2020 coordination action project FarmConners allocated funding to translate ShareWind into a next generation, truly FAIR Share-Wind.net catalogue resource by adopting the GO FAIR foundation FAIRification principles: the most important is to make metadata understandable for both humans and machines by assigning digital object identifiers (DOIs) to data and persistent URLs (PURLs) to standardised community metadata and controlled vocabularies. The ShareWind and Share-Wind prototype family has been tested by different communities including the New European Wind Atlas (NEWA), FarmConners, and IEA Wind Task 41.
Several other platforms exist to share wind energy data with the wider scientific community. Zenodo.org is an often-used platform in which some wind-energy-related communities have started to build up data collections. These platforms are not automatically FAIR as datasets can be tagged using owner-chosen keywords, and some effort is often required to add FAIR domain metadata and controlled vocabularies and/or taxonomies.
The wind energy industry is also starting to make data available; the Danish energy company Ørsted has provided access to data from several sites since 2018, and in 2021, SGRE (Siemens Gamesa Renewable Energy) announced a data portal that can be used by industry and researchers. These few initiatives highlight (by comparison) that most energy-sector data are closed data and cannot be accessed. In 2022, IEA Wind Task 43 made a concerted effort of compiling a catalogue of 38 open data sources related to the wind energy sector (IEA Wind Task 43, 2022a).
A significant challenge to data sharing outside organisations comes from the need to agree on the terms of use for the data. This is usually done through licenses. But there are many potential licenses that can be chosen; as an example, the zenodo.org data repository allows users to license data under one of over 500 pre-existing licenses (zenodo.org, 2023) or to add their own. The need for data providers to choose a license and for data consumers to understand the license costs time and requires specialist support. Licensing can therefore be expensive and slow and so may act as a barrier to sharing by organisations. Some solutions to this challenge that are being worked on to the knowledge of the authors include the following:
-
digital rights management (DRM) solutions, which aim to control the use, modification, and distribution of copyrighted information;
-
data licensing, whereby asset owners and operators generate extra royalty income at no extra cost by providing data platform operators the opportunity to monetise the sales and market intelligence value of their data on their behalf, for example;
-
pre-emptive licensing, which has been used by the Open Energy initiative (participants in the initiative were required to agree to a common license to be able to participate);
-
data leasing, in which access is granted to data through an application programming interface (API) where all the data analysis scripts are monitored;
-
encrypted analysis, in which algorithms are delivered to the data rather than the other way round;
-
federated analytics and differential privacy machine learning techniques, where data are virtually aggregated, allowing organisations to anonymously pool their data.
While these techniques offer great opportunity to bypass concerns around proprietary data sharing, there can be a trade-off between privacy and data accuracy, which needs further exploration.
4.5 Data analytics
Data analytics is the processing of data to find insight. It includes inspecting, cleaning, transforming, and modelling data. Although data analytics has been used in the wind energy industry for some time, the increased amount of data resulting from digitisation and the increase in computing power (following Moore's law and through the availability of cloud computing) will give data analytics an opportunity to add value and uncover new business models (see examples in Sect. 2).
With the availability of more data, both historical and real time, models will be able to scale accordingly to be deployable in the real world. Bach-Andersen et al. (2015) highlights this difficulty in wind energy when explaining that “A big challenge such organisations now face is the question of how the massive amount of operational data that are generated by large fleets are effectively managed and how value is gained from the data”. This will present a new and unique problem to the wind energy industry regarding how to tailor previous solutions to larger-scale data and opportunities to distil information to then be used in real time. One example of this is in the analysis of vibration data for failures (Koukoura et al., 2019), and another is condition assessment for wind turbine gearboxes (Zhu et al., 2019), as well as predicting failures with weather data (Reder et al., 2018).
Data analytics frequently uses models to help in understanding data. These models can take many forms. For example, they could be models of a physical process such as the weather, simulations of goods flowing through a manufacturing plant, or a simple statistical model used for measurement instrument calibration. These models help identify deviations from expected performance given known inputs or forecast future states. The availability of increased computational power allows the use of more complex models, but such models are expensive to operate and maintain and require specialist skills. In contrast, Rezaei et al. (2015) note that “… reduced order models (ROMs) are more desirable due to less computational cost and enough accuracy”. ROMs – often known as “engineering models” – are especially important for real-time or near-real-time applications such as energy forecasting and grid integration, where fast and/or low-cost calculations are needed. ROMs can take many different forms; they may support wind or power forecasting on wind farms, where forecasting horizons could be of the order of a few minutes (Würth et al., 2019). They can be used for wind plant design optimisation, which often needs to run on an analyst's workstation as those analysts may not have access to high-performance computing resources. Similarly, ROMs can also take the form of pre-trained machine learning models or adjoints for uncertainty quantification. For these kinds of scenarios ROMs can be appropriate and more useful than complex models requiring high-performance computing. That said, the authors recognise that computational resources and efficiency are constantly evolving, and the definition of ROMs needs to be adjusted accordingly. These applications also highlight users' need for increased model accuracy and speed improvements without requiring additional computational resources. Data-driven and physics-informed models can fill this critical need for faster, less resource-intensive ROMs.
There is also an additional opportunity with federated learning and privacy-preserving machine learning to collaborate across organisations and share results, as well as models. Lameh et al. (2020) explain the introduction of federated learning by McMahan et al. (2017) as “a decentralized ML approach suitable for edge computing”, with an ability to respond to processing needs. This will bring the ability to advance the wind energy field without the concerns regarding data privacy. Federated learning and privacy-preserving machine learning can enable the deployment and training of a global model system where multiple independent organisations contribute their data (for example, sensor data from a specific wind turbine type) and all users benefit from more accurate models based on more training data, but contributors only ever have access to their own data, as described in Jenkel et al. (2022).
These new opportunities will bring new challenges. With the opportunity to collaborate across the industry and share more information, questions will arise regarding data ownership and control of models.
4.6 Digitalisation
As noted in the Introduction, we define digitalisation as the organisational and industry-wide use of data and digital technologies to improve efficiency, create insights, and develop products and services (Table 1). Digital technologies typically span from sensing to data management to data analytics to artificial intelligence and automation. In short, digitalisation is doing new and value-driven things with digital tools.
The previous sections discussed the combination of technical and cultural measures that are needed to bring data to users to assist informed decision-making. They can form part of a workflow that brings data from sensors to users, allows them to make decisions, and then passes information about that decision to other users (as for smartphones) or back to hardware-level actuators (as in the internet of things). Although many individual workflows might be digitised and rely on digital objects and data management to get data to users and then make decisions based on data analytics, this does not mean that the full benefit of digitalisation has been realised, as digitalisation also benefits from network effects. As more organisations undergo a digital transformation, case studies reported in the literature suggest they will be better able to interact with customers and other digitalised organisations, which in turn leads to more valuable interaction with customers and partners (Lee et al., 2012; Bugshan, 2015; Chen et al., 2020). This cycle of compounding successes forms a virtuous upward spiral (as opposed to a vicious downward cycle).
Like all innovations, new digital workflows or products will go through an adoption process. This process starts with innovators and early adopters who try something out of curiosity or their own values, followed by later adopters who are driven by economic considerations and thus require some degree of certainty that adopting the new approach is worth the effort (see, for example, Palm, 2020). Ultimately, new technologies thrive when they offer a good fit to the social, economic, and technological landscape (Geels, 2002). It is reasonable therefore to expect adoption to vary across organisations and across the wind energy industry, with some groups being further ahead than others. Sharing experience within and across organisations can help build evidence for the benefits and overcome reluctance to change.
The challenges within organisations relate both to people and culture. We have already noted that adoption of digitalisation will require skills such as computer programming, but it will also require that these be better integrated into existing training for science, technology, engineering, and mathematics (STEM) subjects and professional trades. Users will need to be given the opportunity to apply digitalisation – requiring that their organisations support innovation – and will need to be rewarded for their efforts. Furthermore, organisations need to understand the potential value of their data (in all its forms) and develop processes that simplify data-led collaboration. In some cases, these changes may be difficult or disruptive to implement within existing structures, and it may make sense to create in-house incubators, accelerators, or skunkworks to enable innovation.
The wind energy industry needs to explore ways to encourage digitalisation. One way to do this may be to demonstrate the benefits of digitalisation. This could include nationally funded pilot or lighthouse projects but could also include marketplaces to connect innovators and early adopters in rewarding partnerships; one option here may be to (further) open existing marketplaces such as day-ahead energy trading or contract-for-differences schemes and give digital solutions a low-risk environment for testing, where failure or malfunctions have limited or no impact on the rest of the system. These environments are often known as sandboxes. As well as digital sandboxes, hardware test beds are also required to allow solutions to be trialled in realistic settings where risks can be managed. This could include wind turbines from a few hundred kilowatts through to multimegawatt machines (allowing solutions to be transitioned into products) or even multiple wind turbines within operating wind plants. Importantly, these sandboxes and test beds would allow benchmarking against traditional approaches, showing the benefits of digitalisation. These initiatives would also have intangible benefits such as increasing awareness, developing trust, and creating networks of service providers.
4.7 New products, processes, and businesses
The culmination of digitalisation is new modes of business, new ways of doing things, and new means of interacting with people and machines (Ignat, 2017). It is more than simply combining all these features into the latest gadgets but is about effective ways of organising human efforts to make the most out of these innovations. Taken all together, these new models lead to “enabling various new forms of cooperation between companies and leading to new product and service offerings as well as new forms of company relationships with customers and employees” (Rachinger et al., 2019).
New technologies can bring about new businesses, the emergence of failure prediction models, and benefits from predicting failures, but coupled with the open shared data, a few companies can now specialise in failure prediction, providing the service to others and bringing huge economies of scale to the whole industry. Not only is a new company created, but all the clients of the new company receive its benefits. This is particularly the case if digital processes can be turned into software and sold repeatedly (Berkhout et al., 2020).
Properly resourced and directed research institutions and industry organisations are the starting point to spurring research connected to real problems in the field. The research funding needs to be willing to fund efforts further along the innovation pipeline to help bridge the so-called “valley of death” that separates research from commercially relevant products. Industry needs to be properly developed enough to be willing to take risks in reaching backwards across the valley of death. Industry groups need to be well developed enough to guide all actors and instil enough trust and industry guidance to know that their investments will work out and all actors agree on base standards and overall direction.
The organisations need to be designed to be willing to make use of these innovations. Chesbrough (2010) discusses how, without the capability to innovate their business models, companies will have no means of commercialising their ideas and technology. Organisations must have communication to spread ideas across the company, a culture which allows people to speak up, leadership that fosters free thought, and autonomy that allows every employee to act.
Leaders should describe all these efforts in a digitalisation strategy that sets out “a commitment to a set of coherent, mutually reinforcing policies or behaviors aimed at achieving a specific competitive goal” (Pisano, 2015). These organisational principles are true whether the organisational unit is a company, research institute, or government.
Moving beyond simply implementing new technologies into creating entirely new markets requires an ecosystem of new ideas to develop. For example, without a wide combination of technologies, drones for offshore wind would not be possible. This level of innovation requires coordination across organisations. Drones require battery tech, electronic controls, cameras and sensors, data connectivity, and AI control. The potential market of drones for offshore wind energy applications will bring about numerous companies for the support of all these factors. This is encapsulated by Christian Burmeister and Piller (2016), who define business models as “a management hypothesis about what customers want, how they want it, and how the enterprise can organize itself to best meet these needs, get paid for doing so, and make a profit”. The integration of all these aspects, technology, product, customer, and employees, play a part in higher-level digitalisation efforts that provide real value.
How can this vision be implemented in reality? Some of the many relevant initiatives in the wind energy community that aim to bring together all of these needs by sharing data and knowledge to mutually benefit the entire wind energy industry are listed in Table 3.
(Fields et al., 2021)(Macduff and Sivaraman, 2017)(Buljan, 2020)Rodrigo et al., 2014Moriarty et al., 2014(Robertson et al., 2020)(Anderson and Mortensen, 2013)(Perr-Sauer et al., 2021)(Kumar et al., 2022)(Holleran et al., 2022)4.8 Summary
Based on the previous sections, the key aspects of the digital transformation most relevant for the wind energy industry can be summarised as follows.
-
From digitisation to digitalisation. The measurement and storage of data that can be used to make business decisions can be time-consuming and expensive, and new methods and systems are required.
-
Digital objects. These need to be FAIR so that they can be used effectively in the digital transformation.
-
Big data. Handling the large amount of data involved in digitalisation requires both semantic and technical solutions. Semantic solutions include standard metadata and related ontologies, while technical solutions include either new efficient storage systems or new strategies such as linking data distributed across organisations.
-
Data management. A data management policy concerning the way data are collected, stored, and preserved is crucial for getting the right data to the right users within an organisation and for data sharing amongst organisations.
-
Data analytics. New innovations such as federated learning and privacy-preserving machine learning are required to reduce the computational power required for data analytics applied to big data.
-
Digitalisation. The new processes and ways of working required for the successful implementation of the digital transformation need to be fully adopted by people within organisations.
-
New products, processes, and businesses. The successful transformation of new findings based on data analytics into new products, processes, and business can only happen if research institutes, funding bodies, and industry partners actively come together to bridge the valley of death. This could be achieved through new digitalisation strategies.
Wind energy is characterised by a large amount of dispersed infrastructure that generates lots of data, a flexible network between that infrastructure and other systems, and many different stakeholders. Those stakeholders need access to widely varying data and have different abilities to act within or upon the system or with each other. Together the wind turbines, stakeholders, and service providers form a digital ecosystem.
These characteristics are also found in other sectors. For example, the internet of things and smartphones also combine networked hardware, large numbers of users, and large volumes of data. In this section, we examine these sectors and summarise the lessons learned from their specific challenges that could be transferable to the wind energy industry.
5.1 The internet of things
The internet of things (IoT) is a web of a multitude of physical objects – things – that are connected to each other, other systems, and users through the internet. It is gradually becoming a reality as more goods are equipped with sensors during manufacturing and can be connected to ubiquitous internet connections. It leverages cheap sensors and easy access to the internet to provide regular data transfer.
The IoT can be thought of as having several discrete layers that facilitate the movement of data between hardware and users, as well as informed interaction with the hardware (e.g. Sethi and Sarangi, 2017). These include
-
the perception layer, where data are obtained by sensors and actuators can act upon infrastructure;
-
the transport layer, where data are collected from sensors and made accessible for processing (services in this layer also provide secure access to the sensors);
-
the processing layer, where sensor data are stored, aggregated, and interpreted;
-
the application layer, where data are visible to users through specific interfaces, for example for building management, health, or other applications;
-
the business layer, where decisions are made with relation to a person's motives or business's goals.
This layered approach allows data to be transferred back and forth between users and actuators through specialist service providers. A user can then use vertically integrated solutions or combine different services (potentially with the help of an integrator) to get the solution that they need.
The IoT enables many different applications, including vehicle fleet monitoring, automated inventory management, and monitoring national infrastructure. It also has applications for consumers, for example for access passes at entertainment parks (e.g. Disney's “Magic Band”; Marr, 2016) or for order-on-press buttons (e.g. Amazon's “Dash” button). The internet of things is already enabling wind turbine condition monitoring (Coronado and Fischer, 2015).
Adoption of the IoT has not been uniform. The major challenges to adoption reported in a 2016 survey included (in descending order of frequency) privacy and security concerns, the cost, lack of knowledge about solutions, inadequate infrastructure, lack of standards, interoperability concerns, uncertainty that it will deliver the promised benefits, poorly defined workflows, and immature technology (Buntz, 2016). The same challenges should be expected for the digitalisation of wind energy, which is in many ways just an application of IoT approaches.
5.2 The smartphone hardware and software ecosystem
Smartphones are small, portable computers with an internet connection. They run specialised applications or “apps” to carry out a wide range of tasks, from telephony to web browsing, shopping, participating in social networks, and many other purposes.
The apps run on the smartphone's operating system. The apps leverage other apps or service providers to identify users, manage their rights to interact with the app, and charge them for services. From the user's perspective, this is mostly seamless, and improvements can be made by modifying software so long as the hardware is flexible enough. Apps allow consumers to pay for what they consider important and customise their smartphones to meet their needs. Consumers have demonstrated their willingness to spend on apps: in 2021 just under USD 190 billion of revenue was generated by app purchases and subscriptions (Statista, 2023a).
In the early days of smartphones, vendors often created “walled gardens” in that each vendor had their own operating system, effectively locking customers and software developers into that platform (Eisenmann et al., 2008). Today, smartphone vendors instead use several common operating systems, allowing software developers to target the operating system and not the vendor, as well as enabling customers to keep their apps and data, even if they change vendor. For those smartphone businesses that are vertically integrated in that they develop and sell smartphone hardware, operating systems, and apps, almost all allow third-party services to be run on the handset or operating systems. This change was in response to consumers requiring interoperability across hardware, as well as the growth of providers who provide apps for multiple operating systems. However, it has also raised concerns about the ability of handset manufacturers to access data stored on those devices.
The smartphone market includes traditional business-to-consumer (B2C) services such as selling the phone hardware, selling one-time services (e.g. apps), subscription services, and disruptive innovation and experimentation (e.g. Uber and Instagram). It also includes large business-to-business (B2B) services, for example selling network infrastructure, hardware components, software, and back-office services. In 2021, around 1.4 billion handsets were sold for USD 481 billion (Statista, 2023b, c), while the telecom network equipment market was estimated to be around USD 539 billion in 2021 (Acumen, 2023). These related markets grow at between 2 % and 10 % per year as technologies are updated, new businesses are launched, and customer expectations change.
5.3 Lessons for the future wind energy digital ecosystem
The relevant lessons learned from the examples above can be summarised as follows.
-
The digital future will be dynamic, with an unpredictable mixture of actors and technologies.
-
There will be very few, if any, vertical solutions that directly connect the user with the hardware. Instead, most interactions will be between one layer and the next.
-
Standards about interaction between and within different layers will be required to avoid market fragmentation.
-
Specialist service providers will develop to support activities. These may be within a single layer or link one layer to the next.
-
Services such as user authentication, permissions management, and intrusion detection will be essential for safe and reliable operation of the system.
-
Innovation will happen in unexpected places and will take unexpected forms.
-
It will involve a competitive, but collaborative, community.
These lessons learned should also be considered by the wind energy industry as it undergoes its digital transformation.
In this section, we first describe how we identified the grand challenges, and then we introduce them.
6.1 Methodology for identifying the grand challenges
The grand challenges for the digitalisation of wind energy were identified by
-
consideration of the conclusions from the previous sections of this paper;
-
further stakeholder data collection, including (a) data sharing interviews and (b) diversity in wind energy;
-
a combination of findings from steps (1) and (2).
These steps are described in more detail below.
6.1.1 Step 1: consideration of previous conclusions
The conclusions from the previous sections of this paper that are relevant for identifying the grand challenges include the following.
-
Data. A data management policy concerning the way data are collected and stored and preserved is crucial for getting the right data to the right users within an organisation and data sharing amongst organisations. The measurement and storage of data that can be used to make business decisions can be time-consuming and expensive, and new methods and systems are required. To utilise data effectively for the digital transformation, it needs to be FAIR. The large amount of data involved in digitalisation means that new, efficient storage systems and agreed-upon metadata standards are required. New innovations such as federated learning and privacy-preserving machine learning are required to reduce the required computational power of data analytics applied to big data. Services such as user authentication, permissions management, and intrusion detection will be essential for safe and reliable operation of the system.
-
Culture. The digital future will be dynamic, with an unpredictable mixture of actors and technologies. Innovation will happen in unexpected places and will take unexpected forms. The new processes and ways of working required for the successful implementation of the digital transformation need to be fully adopted by people within organisations.
-
Coopetition. The successful transformation of new findings based on data analytics into new products, processes, and business can only happen if research institutes, funding bodies, and industry partners actively come together to bridge the commercialisation valley of death. This could be achieved through new digitalisation strategies. There will be very few, if any, vertical solutions that directly connect the user with the hardware. Instead, most interactions will be between one layer and the next. Standards about interaction between and within different layers will be required to avoid market fragmentation. This will require a competitive, but collaborative, community.
Considering the conclusions from the previous sections of this paper allows us to make two further observations relevant for identifying the grand challenges. The first is that, despite the issues identified in the stakeholder interviews and in this paper, the digitalisation of wind energy is already progressing. Some organisations have made progress in adopting it for parts or all of their businesses. Evidently, the challenges identified by the survey and expert elicitation can be mitigated, and the technical solutions required for digitalisation must exist. Therefore, “the future is already here – it's just not very evenly distributed” (attributed to William Gibson, possibly apocryphal). The second observation is that, because of its cyclical and transitional nature, the process of digitalisation will never be complete.
6.1.2 Step 2(a): data sharing interviews
The data collection activity focused on the specific barriers seen by the wind energy sector in the actual implementation of digitalisation. For this, a total of 30 members of the global wind energy sector were asked to describe their main barriers to data sharing in individual interviews lasting approximately 1 h. This included 7 owner/operators, 7 researchers, and 16 technology providers from the wind energy industry. This specific question was asked because it has already been established that the topic of data sharing and reusing is one of the central challenges in the implementation of digitalisation in wind energy (Fig. 4). The survey was done as part of the activities of IEA Wind Task 43 Technical Area 2. The results are summarised in Table 4. This shows the five barriers with the highest frequencies of occurrence for each interviewee type. Some of the barriers are quite similar between interviewee types, in particular the topics of (a) standard data format/structure, (b) data availability and quality, and (c) data processing and/or preparation time.
Other barriers that came up frequently but did not make the top five include the following.
-
Wind turbine OEMs can make unannounced changes to the controller or be difficult to communicate with.
-
It is difficult to access the most state-of-the-art tools and models, and there is no central location summarising the relevant information.
-
Analysts have different methods, skills sets, and working styles. It is hard to identify what actions will really have an impact.
-
There can be concerns about data security and data privacy related to both personal data and confidential information.
-
It is challenging to find people who are capable of both data analytics and understanding the operation of wind turbines.
It should be noted that these findings generally agree well with the literature survey in Sect. 4. As well as this, it is interesting that most of these barriers are related to company culture, people skills, and communication between different people. These aspects should therefore be included in any discussion about the digitalisation of wind energy, and this is the topic of the second interview series described below.
6.1.3 Step 2(b): diversity in wind energy
We have shown that some of the key barriers to the successful implementation of digitalisation in the wind energy industry are related to company culture, people skills, and communication between different people. This raises the topics of diversity, equity, and inclusion (DEI). In this context, “diversity” refers to involving people from a range of different backgrounds (social and ethnic) and of different ages, genders, religion, sexual orientations, disabilities, education, nationality, and others. “Equity” refers to the quality of being fair and impartial, and “inclusion” refers to providing equal access to opportunities and resources for all. DEI is an increasingly important topic especially in technology- and innovation-based industries.
To examine this topic in more detail and in the context of the wind energy industry, a second data collection activity was carried out by the Diversity Committee of the European Academy of Wind Energy (EAWE). This data collection was focused around a DEI panel discussion at the TORQUE 2020 conference and a DEI workshop series.
The TORQUE 2020 panel discussion was postponed due to the COVID-19 pandemic and actually took place in June 2021. A literature survey carried out in advance of the event showed that technical workforces in general do not represent the general population's diversity in race, gender, and sexual orientation. For example, women represent only 21 % of the global wind energy industry's workforce and only 8 % of its senior management (Ferroukhi et al., 2020). This is known as “under-representation”. It is a problem because the literature survey also found that diverse teams are more productive (for example, gender-diverse companies are 15% more likely to outperform their respective national industry medians) and that diversity is good for business (as diverse companies are 35 % likelier to financially outperform the industry medians). The panel discussion and audience contributions from more than 50+ members of the wind energy research community during the event revealed that the community generally sees and understands the benefits of increasing diversity in wind energy, but many people do not know how to contribute. The expert panellists made some suggestions about how to contribute, including sharing facts and stories with each other, noticing and correcting one's own unconscious biases, and ensuring diverse recruiting teams.
Around 30 wind energy professionals took part in the DEI workshop series. As in the panel discussion described above, it was found that many people simply do not know how to contribute to improving diversity. The main DEI challenges of the wind energy community were found to be (1) attracting, (2) recruiting, and (3) keeping a diverse range of people, as well as (4) ensuring a diverse range of people in higher management. Possible solutions to these challenges suggested by the community included organising specific diversity events at conferences and trade fairs; providing a platform for sharing experiences; developing recruiting guidelines; providing diversity training for team leaders; providing mentoring schemes, networks, and role model events; and providing a framework for proactive hires, exchange programmes, female-only positions, and others.
In summary, the main findings of this data collection activity were that (a) diversity has to be improved in the wind energy industry in order to successfully exploit the advantages of digitalisation and that (b) concrete steps are already being undertaken by the wind energy industry in order to achieve this goal.
As far as the authors are aware, the specific topic of diversity in wind energy digitalisation has not yet been studied. However, discrimination of under-represented groups caused by digitalisation has been well documented – for example, digital technologies are trained on male-biased data (Feldman and Peake, 2021) or existing recruiting patterns that support homogeneous workforces. These technologies then recreate and reinforce these patterns. This is known as the “digital divide” (Sorgner et al., 2017). This topic should be further investigated in connection to wind energy.
6.1.4 Step 3: combination of findings to identify the grand challenges
Based on the observations and interview results presented above, we propose the following three grand challenges in the digitalisation of wind energy:
-
data – creating FAIR data frameworks;
-
culture – connecting people and data to foster innovation;
-
coopetition – enabling collaboration and competition between organisations.
These challenges form a cycle that combines the process of technology transition (Sect. 7) and technology adoption (Venkatesh and Davis, 1996). In this cycle, data are made available, the data are then used as the basis for innovation, and the innovations are bought to market. As a result, more data become available and the cycle repeats. These grand challenges are described in more detail in the following section.
6.2 Defining the grand challenges
The three grand challenges in the digitalisation of wind energy are as follows.
1. Data: creating FAIR data frameworks
Digitalisation is, at its root, the ability to act upon data. An example of this is a decision-making process that brings together many different data sources, analyses them, and takes decisions around that data. Because the same data might be used by many different actors and for many different purposes, the data need to be FAIR.
The interviews presented in Sect. 6.1 confirmed our experience that much of the data generated in the wind energy sector today are not reusable because they are not documented by metadata and thus remain isolated or siloed within organisations or data stores and are simply not findable. In the initial interview round, it was noted that one of the main barriers for a successful digital transformation is the “lack of data standards and interoperability”. This is a well-known problem, and policy leadership by governments, public bodies, and organisations is helping to mitigate this (e.g. European Commission, 2018). The growing requirement by funding agencies for data management plans for research projects is helping to train the next generation of wind energy sector researchers to think about data. Reporting requirements also help generate the data that are needed, for example from the US Energy Information Administration about plant construction and performance (the IEA 860 report) or the North American Electric Reliability Corporation (NERC) reports about generator availability (the NERC GADS reports).
As noted in the interviews, an opportunity for overcoming the challenge of the findability of data is to implement “top-down policies such as FAIR data requirements for scientific journals and research projects”. As introduced in Sect. 4.2, this implies the need for an agreed standard metadata schema and related ontologies to help navigate the wealth of data. There have been some efforts to produce these standard metadata schema and related ontologies, e.g. by the IRPWind project and through activities in IEA Wind Tasks 32, 37, and 43. However, making them converge, bringing them to a maturity level, and spurring community adoption need a concerted effort and sector-level collaboration. This is one example of how reusing data can be considered a grand challenge.
Reusability also requires ways to get data to the right users at the right time. Once data have been found, they need to be made accessible to users. This applies equally within organisations and outside of organisations. While internal data access is an organisation's own challenge to deal with, the wind energy industry needs to improve access to data at the sector level, and the interviews revealed that especially industrial stakeholders saw an opportunity in “data markets allowing access to data, which even can be paid for”. As noted earlier, this access to data can often be essential for innovations (Sovacool and Enevoldsen, 2015).
Although they are required to incentivise data sharing, marketplaces for digital services are rare. Some marketplaces exist for data, such as the US Atmosphere to Electrons (A2e) data archive and portal (https://a2e.energy.gov/about/dap, last access: 1 February 2023) (Macduff and Sivaraman, 2017), while the prototype of the ShareWind metadata registry from EERA JP Wind launched in 2017 has been upgraded to a FAIR searchable data catalogue called Share-wind (https://www.share-wind.com/, last access: 1 October 2022) by the H2020 Coordination Action project FarmConners and is being populated by different projects. These markets are focused on discovering existing data rather than buying or selling data. First steps are being taken to make high-frequency real-time data available, which will be essential for the more flexible operation of wind turbines and wind plants. True markets for digital services (i.e. where services and data can be bought and sold) are needed so that innovators and early adopters can try them out and develop processes that the majority can then benefit from. Like other new markets, these will probably be small, illiquid, and unprofitable at first and need some time to generate enough users to be effective. Therefore, national or sector funding may be required to support the initial growth of data and service markets.
It is our opinion that it is the difficulty of making data reusable across organisations, the need to collaborate to provide the framework to do so, and the potentially huge reward as a result which together make FAIR data a grand challenge for the wind energy industry.
2. Culture: connecting people and data to foster innovation
It has become clear in this paper that reusing data alone will not allow the full potential of the digital transformation to be exploited in the wind energy industry. As expressed in our qualitative data collection, digitalisation and digital R & D succeed when connecting data with models and with people. This can be done, for example, by developing and maintaining new types of organisational cultures, combining staff skills and training needs in new ways, enhancing communication skills, working together on new types of innovation and change processes, and increasing diversity. However, a frequent point mentioned in the interviews was that “organisational change and change management are the biggest challenges … of digital transformations”.
Digitalisation is also a change to established ways of working. One interviewee's comment captured the consensus from many others by noting that “researchers require more digital skills, and furthermore a stronger collaboration with software developers”. It can require new skills from individuals (e.g. computer programming), new ways of thinking (modular and collaborative as opposed to siloed), and new infrastructure (cloud computing and distributed teams rather than centralised services). It also requires organisations to embrace open innovation styles such as co-developing new digital products or reconfiguring existing processes to enable new business ideas. Organisations need to bring these innovations to market, while the market needs to be willing to accept new products or services.
The interviews described in Sect. 6.1 confirmed that some of the key barriers to adopting these new ways of working are actually part of the organisation's own culture and furthermore in the strategy and perception of other actors. Overall, and regardless of the respondents' background, open innovation and open-source paradigms were determined to be key levers for success with the digital transition.
Organisations that can change their culture become well-placed to benefit from digitalisation, while organisations that are less able to change risk losing innovative staff to other organisations that can support them. Such support can take many and varied forms that aim to align internal creativity with the organisation's mission, leading to new products and services (Isaacs and Ancona, 2019). There are many well-established ways to train staff in new skills, change existing corporate culture, or establish new corporate cultures. Therefore, we do not see the process of corporate change per se as a grand challenge.
Similarly, there are many well-established ways for governments to reduce the risk of innovation. Governments can help to reduce financial risk by reducing the cost of innovation through grant funding, tax credits, and many other means. Governments and regulators can also help open the window of opportunity by establishing the right market conditions and by providing safe spaces to trial new technologies, such as specially developed infrastructure.
Instead, it is important to recognise that digitalisation is different from other innovation and change processes because it relies on bringing data and people together to trigger and support innovation. Therefore, organisations need to provide the internal processes for people to access and explore data, mechanisms for these innovations to be identified and nurtured, and spaces to try out their ideas in a business context. Governments and regulators can also support this by creating sandboxes – real or virtual infrastructure – that can be used for pilot projects and, as expressed by a respondent, “understanding that this data revolution brings the energy industry to an inflection point” where regulatory support is required. These are often national or flagship facilities such as the National Renewable Energy Laboratory's (NREL) Energy Systems Integration Facility (ESIF) but can also be regional infrastructure.
In the surveys carried out as part of this paper, a common desire among the respondents, regardless of job title, company type, or geographical location, was to enhance the opportunities for collaboration amongst the diverse actors in the energy industry and beyond. Improving diversity, equality, and inclusion in the wind energy sector may increase the effectiveness of that collaboration. Long-term initiatives to increase gender equality and increase the workforce diversity are therefore essential to the future success of digitalisation.
We consider the need to connect people and data to foster innovation as a sector-wide grand challenge, as it impacts nearly all organisations in the wind energy sector. It requires organisations to offer new approaches to doing business, their customers and partners to request and support these innovations, and government support to be successful. Establishing innovation cultures inside organisations therefore needs collaboration between many stakeholders on a wide range of initiatives, making this a sector-wide challenge.
The result of overcoming this grand challenge will be organisations that have innovation cultures (either in parts or as a whole) and can thus respond to the changing landscape around them. This will be an essential characteristic both to drive digitalisation and to benefit from it.
3. Coopetition: enabling collaboration and competition between organisations
Addressing the grand challenges of reusing data and creating innovation cultures within organisations provides the basis for digitalisation within organisations or narrow sectors. However, one of the biggest potentials of digitalisation is that it allows artificial barriers to be broken down.
Our final grand challenge is therefore to enable cooperation, collaboration, and competition between organisations. This means working together to create marketplaces or business opportunities that would not otherwise exist and that are mutually beneficial. This has happened in other businesses; possibly the best-known example of this is the Bluetooth standard for short-range wireless data exchange, which grew out of collaboration between device manufacturers in the 1990s. This process is sometimes known as coopetition or co-creation and is the antithesis of vertical integration in which internal R & D leads to internal development, production, and distribution.
There are several steps to be taken before this can occur across the wind energy sector, which we define based on our experience combined with the results of the interviews described in this paper. The first is to simplify the process of cooperation across organisations by streamlining non-disclosure agreements, licensing, and other non-technical barriers to cooperation. Together with making data reusable, this will mean that the barriers to cooperation are reduced, which is more likely to happen within small, trusted groups initially (e.g. the UK Open Energy initiative). This may turn into a “race to the bottom,” as streamlined organisations are easier to collaborate with, and once one business starts the process, others must follow or risk being sidelined.
The next step is to set up liquid, easily accessible markets where innovative, collaborative organisations can meet customers. Such marketplaces will likely make extensive use of metadata, sector-level taxonomies, standardised virtual environments, and open-source data libraries to make their services easily findable, interoperable, and reproducible. Data are likely to become a more widely traded commodity. There will be a need for data providers, marketplaces, and data users to implement processes to keep data up to date and maintain interoperability. These processes could leverage approaches used in the software community for open-source software versioning, distribution, and compatibility monitoring (e.g. GitHub.com, PyPI, and others). Otherwise, there is a risk of data becoming unusable, irrelevant, or even misleading over time.
The final aspect of this grand challenge is the need to help all stakeholders understand the potential for digitalisation. This could take many forms, from clearly communicating the results of research projects to benchmarking new tools against established ways of doing things or even by businesses sharing the need for certain new tools. All of these factors together will help generate demand for the new businesses arising from digitalisation.
Together, simplified cooperation across organisational boundaries and liquid markets makes the collaborative promise of digitalisation a reality. However, these are not the final step in digitalisation; making progress on any of these three grand challenges creates the potential for more innovation, new businesses, and new opportunities for coopetition. Therefore, we consider the grand challenges to be cyclical rather than an end-to-end process.
In this paper, we have identified three grand challenges in the digitalisation of wind energy. This was done by first exploring digitalisation as a key pathway for the wind industry to be a cornerstone of a decarbonised, low-cost energy future. Ongoing digitalisation efforts across the wind energy industry were used as examples to identify some of the opportunities and challenges that the industry faces. They also show that the digital wind plants of the future could utilise a host of technologies such as sensors, data, analytics, digital twins, and automation but require clear value propositions to help drive adoption. These technologies will improve safety, efficiency, and reliability, all while reducing costs. The vision of the future in this paper is further informed by collaborative research and expert elicitation across many sectors of the industry. This research has helped illuminate the current state of the industry and critical barriers to overcome. Next, we put forward a more detailed and consensus definition of digitalisation to help readers understand the digital transformation and the key concepts underpinning it, as well as to help us define the grand challenges in the digitalisation of wind energy. We also looked at examples from other industries that can help us accelerate through the learning curve. We find that walled gardens and vendor lock-in prevent innovation ecosystems. However, ecosystems that are open enough to have common data formats and interoperability can lead to new business models, rapid adoption, and best-in-class offerings.
Digitalisation of the wind energy sector offers increased reliability, cost savings, new business models, and cost-effective integration of wind energy as an energy source. But it brings three grand challenges that need to be solved. These are as follows:
-
data – creating FAIR data frameworks;
-
culture – connecting people and data to foster innovation;
-
coopetition – enabling collaboration and competition between organisations.
Addressing the first two grand challenges for the digitalisation of wind energy provides the right conditions for sector-wide adoption. Then, the third grand challenge brings together actors to make progress. Addressing these grand challenges creates a virtuous circle that would lead to increasing digitalisation and increasing benefits to the entire wind energy industry such as reduced costs, improved performance, increased safety, and new business models.
Solving these grand challenges will require contributions from a variety of stakeholders. To that end, we highlight recommendations for individuals and organisations in Table 5. These recommendations were informed by the literature survey and expert interviews presented in this paper.
These recommendations for ways to address the grand challenges in the digitalisation of wind energy provide a framework for a more efficient, inclusive, and innovative wind energy industry. They are only the beginning of a dynamic process that will position wind energy as an essential part of a global clean energy future.
We are unable to release the data from the surveys as they contain opinions that can be directly linked to specific people.
AC led the writing of this paper. AC, SB, AB, PE, JF, AMS, and LW contributed equally to writing and editing all sections, with the following notable contributions: PE came up with the concept for the first interview series and led the writing of Sect. 2; AMS proposed the structure for Sect. 3; AC led Sects. 1, 3.1–3.4, 4, and 6; LW led Sect. 3.5; AB led Sect. 3.6; SB led Sect. 3.8; JF and PE led Sect. 5; and SB led Sect. 5.1.3. YD, JQ, and PT contributed references and to internal reviews. JF led the contribution of members of IEA Wind Task 43 to interviews and early reviews. Figure 1 was produced by the NREL graphics team. Figures 2–5 were produced by PE and revised by AC. Figure 6 was produced by AMS. Figure 7 was produced by Josh Bauer, NREL.
Andrew Clifton, Sarah Barber, Yu Ding, Peter Enevoldsen, Jason Fields, Anna Maria Sempreviva, and Lindy Williams have all received research funding related to the digitalisation of wind energy. Andrew Clifton, Andrew Bray, Mike Purdue, and Philip Totaro provide commercial services related to the digitalisation of wind energy.
The mentioning of any company, brand, product, or service in this paper is purely by way of an example and should not be taken as an endorsement or recommendation.
Publisher's note: Copernicus Publications remains neutral with regard to jurisdictional claims in published maps and institutional affiliations.
This paper benefited from extensive review by members of IEA Wind Task 43. We thank those reviewers for their contributions. The interviews were conducted by some of the authors and others; the interviewers included Sarah Barber, Anna Maria Sempreviva, Andrew Bray, Lindy Williams, Jason Fields, Alec Fiala (RES Americas), Yu Ding, Berthold Hahn (Fraunhofer IWES), Des Farren (SERVUSNet), Corinne Dubois (Meteolien), and Vasiliki Klonari (WindEurope). We thank the interviewers for their efforts and the interviewees for their valuable insights.
We thank the reviewers who provided constructive criticism of this paper after it was submitted to Wind Energy Science.
Anna Maria Sempreviva and Peter Enevoldsen have received support from the Danish Energy Agency EUDP programme, grant no. 64019-0531.
The authors of this paper were individually supported by a range of sources. These include the Ministerium für Wissenschaft, Forschung und Kunst Baden-Württemberg (WindForS Geschäftstelle) and the Energistyrelsen (grant no. 64019-0531).
This paper was edited by Athanasios Kolios and reviewed by Jethro Browell and one anonymous referee.
Acumen: Telecom Equipment Market Size, Share, Analysis Report By Component (Hardware, Software), By Infrastructure (Wired, Wireless), By Technology (2G and 3G, 4G LTE, 5G), By End-user (BFSI, Retail, IT and Telecommunication, Media and Entertainment, Healthcare, Military and Defense, Consumer Electronics, Others), And Region Forecast, 2022–2030, https://www.acumenresearchandconsulting.com/telecom-equipment-market, last access: 1 February 2023. a
Ahmed, M. A. and Kim, Y.-C.: Communication network architectures for smart-wind power farms, Energies, 7, 3900–3921, 2014. a
Anderson, M. and Mortensen, N.: Comparative Resource and Energy Yield Assessment Procedures (CREYAP) Pt. II, AWEA Wind Resource & Project Energy Assessment Seminar, 10–11 December 2013, Las Vegas, NV, USA, https://orbit.dtu.dk/en/publications/comparative-resource-and-energy-yield-assessment-procedures (last access: 1 February 2023), 2013. a
Bach-Andersen, M., Winther, O., and Rømer-Odgaard, B.: Scalable systems for early fault detection in wind turbines: a data driven approach, in: Annual Conference of the European Wind Energy Association, 17–20 November 2015, Paris, France, https://www.ewea.org/annual2015/conference/submit-an-abstract/pdf/6401120788396.pdf (last access: 1 February 2023), 2015. a
Benjamin, M., Gagnon, P., Rostamzadeh, N., Pal, C., Bengio, Y., and Shee, A.: Towards Standardization of Data Licenses: The Montreal Data License, arxiv [preprint], https://doi.org/10.48550/ARXIV.1903.12262, 2019. a
Berkhout, V., Machill, B., and Reintanz, L.: Applications and platforms in digitalisation of wind farm O&M – community feedback and survey results, J. Phys. Conf. Ser., 1669, 012006, https://doi.org/10.1088/1742-6596/1669/1/012006, 2020. a, b, c
Bird, L., Cochran, J., and Wang, X.: Wind and Solar Energy Curtailment: Experience and Practices in the United States, Tech. Rep. NREL/TP-6A20-60983, National Renewable Energy Laboratory, Golden, CO, USA, https://doi.org/10.2172/1126842, 2014. a
Branca, T. A., Fornai, B., Colla, V., Murri, M. M., Streppa, E., and Schröder, A. J.: The Challenge of Digitalization in the Steel Sector, Metals, 10, 288, https://doi.org/10.3390/met10020288, 2020. a
Branlard, E., Giardina, D., and Brown, C. S. D.: Augmented Kalman filter with a reduced mechanical model to estimate tower loads on a land-based wind turbine: a step towards digital-twin simulations, Wind Energ. Sci., 5, 1155–1167, https://doi.org/10.5194/wes-5-1155-2020, 2020. a
Brannstrom, C., Pasqualetti, M. J., Gorayeb, A., Wolsink, M., Slattery, M., Devine-Wright, P., and Sovacool, B.: Why ignore a major challenge of wind energy science?, https://science.sciencemag.org/content/366/6464/eaau2027/tab-e-letters (last access: 1 February 2023), 2019. a
Bugshan, H.: Co-innovation: the role of online communities, J. Strateg. Market., 23, 175–186, https://doi.org/10.1080/0965254X.2014.920905, 2015. a
Buljan, A.: New Drone Prototype to Start Offshore Wind Trials Next Year, https://www.offshorewind.biz/2020/12/10/new-drone-prototype-to-start-offshore-wind-trials-next-year/ (last access: 22 February 2022), 2020. a
Buntz, B.: Top 10 reasons people aren't embracing the IOT, https://www.iotworldtoday.com/2016/04/20/top-10-reasons-people-aren-t-embracing-iot/ (last access: 22 February 2022), 2016. a
Bush, B., Brunhart-Lupo, N., Bugbee, B., Krishnan, V., Potter, K., and Gruchalla, K.: Coupling visualization, simulation, and deep learning for ensemble steering of complex energy models, in: 2017 IEEE Workshop on Data Systems for Interactive Analysis (DSIA), 1–2 October 2017, Phoenix, AZ, USA, https://doi.org/10.1109/dsia.2017.8339087, 2017. a
Castro, G. D. R., Fernández, M. C. G., and Colsa, Á. U.: Unleashing the convergence amid digitalization and sustainability towards pursuing the Sustainable Development Goals (SDGs): A holistic review, J. Clean. Product., 280, 122204, https://doi.org/10.1016/j.jclepro.2020.122204, 2021. a
Chen, S., Kang, J., Liu, S., and Sun, Y.: Cognitive computing on unstructured data for customer co-innovation, Eur. J. Market., 54, 570–593, https://doi.org/10.1108/EJM-01-2019-0092, 2020. a
Chesbrough, H.: Business Model Innovation: Opportunities and Barriers, Long Range Plan., 43, 354–363, https://doi.org/10.1016/j.lrp.2009.07.010, 2010. a
Christian Burmeister, D. L. and Piller, F. T.: Business Model Innovation for Industrie 4.0: Why the “Industrial Internet” Mandates a New Perspective on Innovation, Unternehmung, 70, 124–152, https://doi.org/10.5771/0042-059x-2016-2-124, 2016. a
Coronado, D. and Fischer, K.: Condition monitoring of wind turbines: State of the art, user experience and recommendations, Tech. rep., Fraunhofer IWES, Bremerhaven, Germany, https://publica.fraunhofer.de/documents/N-352558.html (last access: 1 February 2023), 2015. a
Dörenkämper, M., Olsen, B. T., Witha, B., Hahmann, A. N., Davis, N. N., Barcons, J., Ezber, Y., García-Bustamante, E., González-Rouco, J. F., Navarro, J., Sastre-Marugán, M., Sīle, T., Trei, W., Žagar, M., Badger, J., Gottschall, J., Rodrigo, J. S., and Mann, J.: The Making of the New European Wind Atlas – Part 2: Production and evaluation, Geosci. Model Dev., 13, 5079–5102, https://doi.org/10.5194/gmd-13-5079-2020, 2020. a
Duffy, A., Hand, M., Wiser, R., Lantz, E., Dalla Riva, A., Berkhout, V., Stenkvist, M., Weir, D., and Lacal-Arántegui, R.: Land-based wind energy cost trends in Germany, Denmark, Ireland, Norway, Sweden and the United States, Appl. Energy, 277, 114777, https://doi.org/10.1016/j.apenergy.2020.114777, 2020. a
Dykes, K., Hand, M., Stehly, T., Veers, P., Robinson, M., Lantz, E., and Tusing, R.: Enabling the SMART Wind Power Plant of the Future Through Science-Based Innovation, Tech. Rep. NREL/TP-5000-68123, National Renewable Energy Laboratory, Golden, CO, USA, https://www.nrel.gov/docs/fy17osti/68123.pdf (last access: 1 February 2023), 2017. a
Dykes, K. L., Veers, P. S., Lantz, E. J., Holttinen, H., Carlson, O., Tuohy, A., Sempreviva, A. M., Clifton, A., Rodrigo, J. S., Berry, D. S., Laird, D., Carron, W. S., Moriarty, P. J., Marquis, M., Meneveau, C., Peinke, J., Paquette, J., Johnson, N., Pao, L., Fleming, P. A., Bottasso, C., Lehtomaki, V., Robertson, A. N., Muskulus, M., Manwell, J., Tande, J. O., Sethuraman, L., Roberts, J. O., and Fields, M. J.: IEA Wind TCP: Results of IEA Wind TCP Workshop on a Grand Vision for Wind Energy Technology, Tech. rep., National Renewable Energy Laboratory, https://doi.org/10.2172/1508509, 2019. a
Eggert, M., Stepputat, M., and Fluegge, W.: Digital Assistance in the Maintenance of Offshore Wind Parks, J. Phys.: Conf. Ser., 1669, 012001, https://doi.org/10.1088/1742-6596/1669/1/012001, 2020. a
Eisenmann, T. R., Parker, G., and Alstyne, M. W. V.: Opening Platforms: How, When and Why?, SSRN Electron. J., Working Paper 09-030, Harvard Business School, https://doi.org/10.2139/ssrn.1264012, 2008. a
European Commission: H2020 Programme: Guidelines on FAIR Data Management in Horizon 2020, https://ec.europa.eu/research/participants/data/ref/h2020/grants_manual/hi/oa_pilot/h2020-hi-oa-data-mgt_en.pdf (last access: 1 February 2023), 2016. a
European Commission: Turning FAIR into reality: final report and action plan from the European Commission expert group on FAIR data, European Commission Directorate General for Research and Innovation, https://doi.org/10.2777/1524, 2018. a
Feldman, T. and Peake, A.: End-To-End Bias Mitigation: Removing Gender Bias in Deep Learning, arxiv [preprint], https://doi.org/10.48550/ARXIV.2104.02532, 2021. a
Fernández-Portillo, A., Almodóvar-González, M., Coca-Pérez, J. L., and Jiménez-Naranjo, H. V.: Is Sustainable Economic Development Possible Thanks to the Deployment of ICT?, Sustainability, 11, 6307, https://doi.org/10.3390/su11226307, 2019. a
Ferroukhi, R., Renner, M., and García-Baños, C.: Wind energy: A gender perspective, International Renewable Energy Agency, Dubai, https://www.irena.org/publications/2020/Jan/Wind-energy-A-gender-perspective (last access: 1 February 2023), 2020. a
Fields, M. J., Optis, M., Perr-Sauer, J., Todd, A., Lee, J. C. Y., Meissner, J., Simley, E., Bodini, N., Williams, L., Sheng, S., and Hammond, R.: Wind Plant Performance Prediction Benchmark Phase 1 Technical Report, Tech. Rep. NREL/TP-5000-78715, National Renewable Energy Laboratory, Golden, CO, USA, https://www.nrel.gov/docs/fy22osti/78715.pdf (last access: 1 February 2023), 2021. a, b
Fleming, P., Annoni, J., Shah, J. J., Wang, L., Ananthan, S., Zhang, Z., Hutchings, K., Wang, P., Chen, W., and Chen, L.: Field test of wake steering at an offshore wind farm, Wind Energ. Sci., 2, 229–239, https://doi.org/10.5194/wes-2-229-2017, 2017. a
Gauld, J., Bennun, L., Cook, A., Jobson, B., Oppel, S., Allinson, T. S., Franco, A., Gregory, R., Green, R., Humphreys, L., McCluskie, A., Petrovan, S., Silva, J. P., Thaxter, C., Wischnewski, S., and Wright, L.: The fourth grand challenge in the science of wind energy: minimizing biodiversity impacts, https://science.sciencemag.org/content/366/6464/eaau2027/tab-e-letters (last access: 1 February 2023), 2019. a
Geels, F. W.: Technological transitions as evolutionary reconfiguration processes: a multi-level perspective and a case-study, Res. Policy, 31, 1257–1274, https://doi.org/10.1016/s0048-7333(02)00062-8, 2002. a, b
González-Aparicio, I. and Zucker, A.: Impact of wind power uncertainty forecasting on the market integration of wind energy in Spain, Appl. Energy, 159, 334–349, https://doi.org/10.1016/j.apenergy.2015.08.104, 2015. a
Hahmann, A. N., Sīle, T., Witha, B., Davis, N. N., Dörenkämper, M., Ezber, Y., García-Bustamante, E., González-Rouco, J. F., Navarro, J., Olsen, B. T., and Söderberg, S.: The making of the New European Wind Atlas – Part 1: Model sensitivity, Geosci. Model Dev., 13, 5053–5078, https://doi.org/10.5194/gmd-13-5053-2020, 2020. a
Heinz, J., Kennedy, J., Bradshaw, L., Raidt, J., Harding, M., Gurin, J., Wittes, B., and Cooper, R.: The Future of Data-Driven Innovation, Tech. rep., US Chamber of Commerce Foundation, Washington, DC, https://www.uschamberfoundation.org/sites/default/files/Data Report Final 10.23.pdf (last access: 1 February 2023), 2014. a
Holleran, S., Roscheck, F., Westermann, H., Fields, J., Kersting, G., Bohara, A., Purdue, M., and Lee, J.: IEA-Task-43/digital_wra_data_standard: 1.0.0-2022.01, Zenodo [code], https://doi.org/10.5281/ZENODO.5841468, 2022. a, b
IEA Wind Task 43: IEA Wind Task 43 Open Data Catalogue, https://www.ieawindtask43.org/proceedings-work-products/open-data-resources (last access: 1 February 2023), 2022a. a
IEA Wind Task 43: IEA Wind Task 43 Glossary, https://github.com/IEA-Task-43/glossary (last access: 1 February 2023), 2022b. a
Ignat, V.: Digitalization and the global technology trends, IOP Conf. Ser.: Mater. Sci. Eng., 227, 012062, https://doi.org/10.1088/1757-899x/227/1/012062, 2017. a
IRENA: Renewable Energy Power Generation Costs in 2020, Tech. rep., International Renewable Energy Agency, Abu Dhabi, https://www.irena.org/publications/2021/Jun/Renewable-Power-Costs-in-2020 (last access: 1 February 2023), 2021. a
Isaacs, K. and Ancona, D.: 3 Ways to Build a Culture of Collaborative Innovation, https://hbr.org/2019/08/3-ways-to-build-a-culture-of-collaborative-innovation (last access: 22 February 2022), 2019. a
Ishwarappa and Anuradha, J.: A Brief Introduction on Big Data 5Vs Characteristics and Hadoop Technology, Proced. Comput. Sci., 48, 319–324, https://doi.org/10.1016/j.procs.2015.04.188, 2015. a
ISO: Industrial systems, installations and equipment and industrial products – Structuring principles and reference designations – Part 10: Power supply systems, Standard, ISO – International Organization for Standardization, Geneva, https://www.iso.org/standard/75471.html (last access: 1 February 2023), 2022. a
Jacobson, M. Z.: Roadmaps to Transition Countries to 100 % Clean, Renewable Energy for All Purposes to Curtail Global Warming, Air Pollution, and Energy Risk, Earth's Future, 5, 948–952, https://doi.org/10.1002/2017ef000672, 2017. a
Jenkel, L., Jonas, S., and Meyer, A.: Towards Fleet-wide Sharing of Wind Turbine Condition Information through Privacy-preserving Federated Learning, arxiv [preprint], https://doi.org/10.48550/ARXIV.2212.03529, 2022. a
Kapp, F., Matthes, N., Kruse, L., Niebeling, M., and Spangenberger, P.: Fehlerdiagnose mit Virtual Reality trainieren – Entwicklung und Erprobung einer virtuellen Offshore-Windenergieanlage, Z. Arbeitswissen., 76, 192–201, https://doi.org/10.1007/s41449-022-00316-8, 2022. a
Koukoura, S., Carroll, J., and McDonald, A.: On the use of AI based vibration condition monitoring of wind turbine gearboxes, J. Phys.: Conf. Ser., 1222, 012045, https://doi.org/10.1088/1742-6596/1222/1/012045, 2019. a, b
Kumar, N., Prakash, A., and Ding, Y.: Data Science for Wind Energy, https://cran.r-project.org/web/packages/DSWE/ (last access: 22 February 2021), 2022. a
Lameh, S. F., Noble, W., Amannejad, Y., and Afshar, A.: Analysis of Federated Learning as a Distributed Solution for Learning on Edge Devices, in: 2020 International Conference on Intelligent Data Science Technologies and Applications (IDSTA), 19–22 October 2020, virtual, https://doi.org/10.1109/idsta50958.2020.9264060, 2020. a
Lee, J. C. Y., Stuart, P., Clifton, A., Fields, M. J., Perr-Sauer, J., Williams, L., Cameron, L., Geer, T., and Housley, P.: The Power Curve Working Group's assessment of wind turbine power performance prediction methods, Wind Energ. Sci., 5, 199–223, https://doi.org/10.5194/wes-5-199-2020, 2020. a
Lee, S. M., Olson, D. L., and Trimi, S.: Co‐innovation: convergenomics, collaboration, and co‐creation for organizational values, Manage. Decis., 50, 817–831, https://doi.org/10.1108/00251741211227528, 2012. a
Lund, H. and Sempreviva, A.: Semi-automatic taxonomy development for wind energy data collections, in: Proceedings of the 2019 Wind Energy Science Conference (WESC 2019), 17–20 June 2019, Cork, Ireland, https://doi.org/10.5281/zenodo.3368636, 2019. a, b
Macduff, M. and Sivaraman, C.: GC13J-1656: Preserving Data for Renewable Energy, in: presented at 2017 Fall Meeting, AGU, 11–15 December 2017, New Orleans, https://agu.confex.com/agu/fm17/meetingapp.cgi/Paper/233486 (last access: 1 February 2023), 2017. a, b
Maksimov, Y. V. and Fricker, S.: Licensing in Artificial Intelligence Competitions and Consortium Project Collaborations, in: 2020 46th Euromicro Conference on Software Engineering and Advanced Applications (SEAA), 26–28 August 2020, Portoroz, Slovenia, 292–301, https://doi.org/10.1109/SEAA51224.2020.00056, 2020. a
Marr, B.: Walt Disney Parks and Resorts: How Big Data is Transforming Our Family Holidays, in: Big Data in Practice, John Wiley & Sons, Ltd, 211–215, https://doi.org/10.1002/9781119278825.ch33, 2016. a
Mathis, C.: Data Lakes, Datenbank-Spektrum, 17, 289–293, https://doi.org/10.1007/s13222-017-0272-7, 2017. a
McMahan, H. B., Moore, E., Ramage, D., Hampson, S., and y Arcas, B. A.: Communication-Efficient Learning of Deep Networks from Decentralized Data. Proceedings of the 20th International Conference on Artificial Intelligence and Statistics (AISTATS), Proc. Mach. Learn. Res., 54, 1273–1282, 2017. a
Mergel, I., Edelmann, N., and Haug, N.: Defining digital transformation: Results from expert interviews, Govern. Inform. Quart., 36, 101385, https://doi.org/10.1016/j.giq.2019.06.002, 2019. a
Michiorri, A., Sempreviva, A. M., Philipp, S., Perez-Lopez, P., Ferriere, A., and Moser, D.: Topic Taxonomy and Metadata to Support Renewable Energy Digitalisation, Energies, 15, 9531, https://doi.org/10.3390/en15249531, 2022. a
Moriarty, P., Rodrigo, J. S., Gancarski, P., Chuchfield, M., Naughton, J. W., Hansen, K. S., Machefaux, E., Maguire, E., Castellani, F., Terzi, L., Breton, S.-P., and Ueda, Y.: IEA-Task 31 WAKEBENCH: Towards a protocol for wind farm flow model evaluation. Part 2: Wind farm wake models, J. Phys.: Conf. Ser., 524, 012185, https://doi.org/10.1088/1742-6596/524/1/012185, 2014. a
Naeher, D.: Technology Adoption Under Costly Information Processing, Int. Econ. Rev., 63, 699–753, https://doi.org/10.1111/iere.12545, 2022. a
Nagasawa, T., Pillay, C., Beier, G., Fritzsche, K., Pougel, F., Takama, T., The, K., and Bobashev, I.: Accelerating clean energy through Industry 4.0: manufacturing the next revolution, Tech. rep., UNIDO – United Nations Industrial Development Organization, Vienna, Austria, https://www.unido.org/sites/default/files/2017-08/REPORT_Accelerating_clean_energy_through_Industry_4.0.Final_0.pdf (last access: 1 February 2023), 2017. a
Nycander, E., Söder, L., Olauson, J., and Eriksson, R.: Curtailment analysis for the Nordic power system considering transmission capacity, inertia limits and generation flexibility, Renew. Energy, 152, 942–960, https://doi.org/10.1016/j.renene.2020.01.059, 2020. a
Palm, A.: Early adopters and their motives: Differences between earlier and later adopters of residential solar photovoltaics, Renew. Sustain. Energ. Rev., 133, 110142, https://doi.org/10.1016/j.rser.2020.110142, 2020. a
Perr-Sauer, J., Optis, M., Fields, J. M., Bodini, N., Lee, J. C., Todd, A., Simley, E., Hammond, R., Phillips, C., Lunacek, M., Kemper, T., Williams, L., Craig, A., Agarwal, N., Sheng, S., and Meissner, J.: OpenOA: An Open-Source Codebase For Operational Analysis of Wind Farms, J. Open Source Softw., 6, 2171, https://doi.org/10.21105/joss.02171, 2021. a
Pettas, V., Salari, M., Schlipf, D., and Cheng, P. W.: Investigation on the potential of individual blade control for lifetime extension, J. Phys.: Conf. Ser., 1037, 032006, https://doi.org/10.1088/1742-6596/1037/3/032006, 2018. a, b
Pisano, G.: You Need an Innovation Strategy, https://hbr.org/2015/06/you-need-an-innovation-strategy (last access: 22 February 2022), 2015. a
Rachinger, M., Rauter, R., Müller, C., Vorraber, W., and Schirgi, E.: Digitalization and its influence on business model innovation, J. Manufact. Technol. Manage., 30, 1143–1160, https://doi.org/10.1108/jmtm-01-2018-0020, 2019. a, b, c
Reder, M., Yürüşen, N. Y., and Melero, J. J.: Data-driven learning framework for associating weather conditions and wind turbine failures, Reliabil. Eng. Syst. Safe., 169, 554–569, https://doi.org/10.1016/j.ress.2017.10.004, 2018. a
Rezaei, M. M., Behzad, M., Haddadpour, H., and Moradi, H.: Development of a reduced order model for nonlinear analysis of the wind turbine blade dynamics, Renew. Energy, 76, 264–282, https://doi.org/10.1016/j.renene.2014.11.021, 2015. a
Rinker, J. M., Hansen, M. H., and Larsen, T. J.: Calibrating a wind turbine model using diverse datasets, J. Phys.: Conf. Ser., 1037, 062026, https://doi.org/10.1088/1742-6596/1037/6/062026, 2018. a
Robertson, A. N., Gueydon, S., Bachynski, E., Wang, L., Jonkman, J., Alarcón, D., Amet, E., Beardsell, A., Bonnet, P., Boudet, B., Brun, C., Chen, Z., Féron, M., Forbush, D., Galinos, C., Galvan, J., Gilbert, P., Gómez, J., Harnois, V., Haudin, F., Hu, Z., Dreff, J. L., Leimeister, M., Lemmer, F., Li, H., Mckinnon, G., Mendikoa, I., Moghtadaei, A., Netzband, S., Oh, S., Pegalajar-Jurado, A., Nguyen, M. Q., Ruehl, K., Schünemann, P., Shi, W., Shin, H., Si, Y., Surmont, F., Trubat, P., Qwist, J., and Wohlfahrt-Laymann, S.: OC6 Phase I: Investigating the underprediction of low-frequency hydrodynamic loads and responses of a floating wind turbine, J. Phys.: Conf. Ser., 1618, 032033, https://doi.org/10.1088/1742-6596/1618/3/032033, 2020. a
Rodrigo, J. S., Gancarski, P., Arroyo, R. C., Moriarty, P., Chuchfield, M., Naughton, J. W., Hansen, K. S., Machefaux, E., Koblitz, T., Maguire, E., Castellani, F., Terzi, L., Breton, S.-P., Ueda, Y., Prospathopoulos, J., Oxley, G. S., Peralta, C., Zhang, X., and Witha, B.: IEA-Task 31 WAKEBENCH: Towards a protocol for wind farm flow model evaluation. Part 1: Flow-over-terrain models, J. Phys.: Conf. Ser., 524, 012105, https://doi.org/10.1088/1742-6596/524/1/012105, 2014. a
Ross, P. S., Dearden, A., Araújo-Wang, C., Bejder, L., Reeves, R. R., Rose, N. A., Winkler, R., Wright, A. J., and Wang, J. Y.: RE: Wind energy: Indirect Cause of Extinction for Taiwanese white dolphins?, https://science.sciencemag.org/content/366/6464/eaau2027/tab-e-letters (last access: 1 February 2023), 2020. a
Schlipf, D., Hille, N., Raach, S., Scholbrock, A., and Simley, E.: IEA Wind Task 32: Best Practices for the Certification of Lidar-Assisted Control Applications, J. Phys.: Conf. Ser., 1102, 012010, https://doi.org/10.1088/1742-6596/1102/1/012010, 2018. a
Schwanitz, V. J., Wierling, A., Biresselioglu, M. E., Celino, M., Demir, M. H., Bałazińska, M., Kruczek, M., Paier, M., and Suna, D.: Current state and call for action to accomplish findability, accessibility, interoperability, and reusability of low carbon energy data, Sci. Rep., 12, 5208, https://doi.org/10.1038/s41598-022-08774-0, 2022. a
Schwardmann, U.: Digital Objects – FAIR Digital Objects: Which Services Are Required?, Data Sci. J., 19, 15, https://doi.org/10.5334/dsj-2020-015, 2020. a
Sempreviva, A. M.: Find the data: Metadata and taxonomy for FAIR data sharing in Wind Energy, presented at EERAdata online workshop & hackathon, 2–4 June 2020, online, Zenodo, https://doi.org/10.5281/ZENODO.5412367, 2020. a
Sempreviva, A. M., Vesth, A., Bak, C., Verelst, R. D., Giebel, G., Danielsen, H. K., Mikkelsen, L. P., Andersson, M., Vasiljevic, N., Barth, S., Rodrigo, J. S., Gancarski, P., Reigstad, T. I., Bolstad, H. C., Wagenaar, J. W., and Hermans, W. K.: Taxonomy And Metadata For Wind Energy Research & Development, Zenodo, https://doi.org/10.5281/ZENODO.1199489, 2017. a, b
Sempreviva, A. M., Dimitrov, N., Vasiljevic, N., Davis, N., Lavanchy, P., and Hüser, F.: Open science: sharing data, tools and workflows. A strategy to inspire efficient collaboration, in: Proceedings of the WindEurope Conference & Exhibition 2019 (WindEurope 2019), PO.094, Zenodo, https://doi.org/10.5281/ZENODO.2634496, 2019. a
Sethi, P. and Sarangi, S. R.: Internet of Things: Architectures, Protocols, and Applications, J. Elect. Comput. Eng., 2017, 1–25, https://doi.org/10.1155/2017/9324035, 2017. a
Simley, E., Millstein, D., Jeong, S., and Fleming, P.: The value of wake steering wind farm control in U.S. energy markets, Wind Energ. Sci. Discuss. [preprint], https://doi.org/10.5194/wes-2023-12, in review, 2023. a, b
SKF: Maintenance 4.0 in wind farms: Bringing smart analytics to clean energy, https://industrial-ai.skf.com/maintenance-4-0-in-wind-farms, last access: May 2021. a
Sorgner, A., Bode, E., and Krieger-Boden, C.: The Effects of Digitalization on the Gender Equality in the G20 economies, Tech. rep., Kiel Institute for the World Economy, Kiel, Germany, https://www.emsdialogues.org/wp-content/uploads/2017/08/20170707_W20_Studie_v2.5.pdf (last access: 1 February 2023), 2017. a
Sovacool, B. K. and Enevoldsen, P.: One style to build them all: Corporate culture and innovation in the offshore wind industry, Energy Policy, 86, 402–415, https://doi.org/10.1016/j.enpol.2015.07.015, 2015. a, b
Statista: Digital Markets Insights: App – Worldwide, https://www.statista.com/outlook/dmo/app/worldwide (last access: 1 February 2023), 2023a. a
Statista: Number of smartphones sold to end users worldwide from 2007 to 2021, https://www.statista.com/statistics/263437/global-smartphone-sales-to-end-users-since-2007/ (last access: 1 February 2023), 2023b. a
Statista: Smartphone market revenue worldwide from 2013 to 2026, https://www.statista.com/forecasts/1286699/worldwide-smartphone-market-revenue (last access: 1 February 2023), 2023c. a
US Department Of Energy: FAIR data and models for Artificial Intelligence And machine learning – Funding Opportunity Announcement (FOA) number: DE-FOA-0002306, https://science.osti.gov/-/media/grants/pdf/foas/2020/SC_FOA_0002306.pdf (last access: 22 February 2022), 2020. a
van Kuik, G. A. M., Peinke, J., Nijssen, R., Lekou, D., Mann, J., Sørensen, J. N., Ferreira, C., van Wingerden, J. W., Schlipf, D., Gebraad, P., Polinder, H., Abrahamsen, A., van Bussel, G. J. W., Sørensen, J. D., Tavner, P., Bottasso, C. L., Muskulus, M., Matha, D., Lindeboom, H. J., Degraer, S., Kramer, O., Lehnhoff, S., Sonnenschein, M., Sørensen, P. E., Künneke, R. W., Morthorst, P. E., and Skytte, K.: Long-term research challenges in wind energy – a research agenda by the European Academy of Wind Energy, Wind Energ. Sci., 1, 1–39, https://doi.org/10.5194/wes-1-1-2016, 2016. a, b
Veers, P., Dykes, K., Lantz, E., Barth, S., Bottasso, C. L., Carlson, O., Clifton, A., Green, J., Green, P., Holttinen, H., Laird, D., Lehtomäki, V., Lundquist, J. K., Manwell, J., Marquis, M., Meneveau, C., Moriarty, P., Munduate, X., Muskulus, M., Naughton, J., Pao, L., Paquette, J., Peinke, J., Robertson, A., Rodrigo, J. S., Sempreviva, A. M., Smith, J. C., Tuohy, A., and Wiser, R.: Grand challenges in the science of wind energy, Science, 366, eaau2027, https://doi.org/10.1126/science.aau2027, 2019. a, b
Venkatesh, V. and Davis, F. D.: A Model of the Antecedents of Perceived Ease of Use: Development and Test, Decis. Sci., 27, 451–481, https://doi.org/10.1111/j.1540-5915.1996.tb00860.x, 1996. a
Wang, B., Ha-Brookshire, J. E., and Bonifay, W.: Measuring Perceived Benefits and Costs of New Technology Adoption in the Chinese Textile and Apparel Industry, Cloth. Textil. Res. J., 40, 187–202, https://doi.org/10.1177/0887302X20969889, 2022. a
Wilczak, J., Finley, C., Freedman, J., Cline, J., Bianco, L., Olson, J., Djalalova, I., Sheridan, L., Ahlstrom, M., Manobianco, J., Zack, J., Carley, J. R., Benjamin, S., Coulter, R., Berg, L. K., Mirocha, J., Clawson, K., Natenberg, E., and Marquis, M.: The Wind Forecast Improvement Project (WFIP): A Public–Private Partnership Addressing Wind Energy Forecast Needs, B. Am. Meteorol. Soc., 96, 1699–1718, https://doi.org/10.1175/BAMS-D-14-00107.1, 2015. a
Wilkinson, M. D., Dumontier, M., Aalbersberg, I. J., Appleton, G., Axton, M., Baak, A., Blomberg, N., Boiten, J.-W., da Silva Santos, L. B., Bourne, P. E., Bouwman, J., Brookes, A. J., Clark, T., Crosas, M., Dillo, I., Dumon, O., Edmunds, S., Evelo, C. T., Finkers, R., Gonzalez-Beltran, A., Gray, A. J., Groth, P., Goble, C., Grethe, J. S., Heringa, J., 't Hoen, P. A., Hooft, R., Kuhn, T., Kok, R., Kok, J., Lusher, S. J., Martone, M. E., Mons, A., Packer, A. L., Persson, B., Rocca-Serra, P., Roos, M., van Schaik, R., Sansone, S.-A., Schultes, E., Sengstag, T., Slater, T., Strawn, G., Swertz, M. A., Thompson, M., van der Lei, J., van Mulligen, E., Velterop, J., Waagmeester, A., Wittenburg, P., Wolstencroft, K., Zhao, J., and Mons, B.: The FAIR Guiding Principles for scientific data management and stewardship, Sci. Data, 3, 624646, https://doi.org/10.1038/sdata.2016.18, 2016. a
Wiser, R., Rand, J., Seel, J., Beiter, P., Baker, E., Lantz, E., and Gilman, P.: Expert elicitation survey predicts 37 % to 49 % declines in wind energy costs by 2050, Nat. Energy, 6, 555–565, https://doi.org/10.1038/s41560-021-00810-z, 2021. a, b
Wood, D.: Grand Challenges in Wind Energy Research, Front. Energ. Res., 8, 624646, https://doi.org/10.3389/fenrg.2020.624646, 2020. a
Würth, I., Valldecabres, L., Simon, E., Möhrlen, C., Uzunoğlu, B., Gilbert, C., Giebel, G., Schlipf, D., and Kaifel, A.: Minute-Scale Forecasting of Wind Power – Results from the Collaborative Workshop of IEA Wind Task 32 and 36, Energies, 12, 712, https://doi.org/10.3390/en12040712, 2019. a
zenodo.org: Zenodo REST API, https://zenodo.org/api/licenses/?page=1&size=1000, last access: 1 February 2023. a
Zhu, Y., Zhu, C., Song, C., Li, Y., Chen, X., and Yong, B.: Improvement of reliability and wind power generation based on wind turbine real-time condition assessment, Int. J. Elect. Power Energ. Syst., 113, 344–354, https://doi.org/10.1016/j.ijepes.2019.05.027, 2019. a
- Abstract
- Introduction
- Using data to reduce the cost of energy from wind
- Research methods and materials
- Understanding the digital transformation
- Lessons learned from other networked industries
- The grand challenges
- Conclusions
- Data availability
- Author contributions
- Competing interests
- Disclaimer
- Acknowledgements
- Financial support
- Review statement
- References
grand challengesaround data, culture, and coopetition. This paper describes how the wind energy industry could work with R&D organisations, funding agencies, and others to overcome them.
- Abstract
- Introduction
- Using data to reduce the cost of energy from wind
- Research methods and materials
- Understanding the digital transformation
- Lessons learned from other networked industries
- The grand challenges
- Conclusions
- Data availability
- Author contributions
- Competing interests
- Disclaimer
- Acknowledgements
- Financial support
- Review statement
- References